BRICS – Efficient Techniques for Estimating the Farness-Centrality in Parallel
2019 IEEE International Parallel and Distributed Processing Symposium Workshops (IPDPSW)(2019)
摘要
In this paper, we study scalable parallel algorithms for estimating the farness-centrality value of the nodes in a given undirected and connected graph. Our algorithms consider approaches that are more suitable for sparse graphs. To this end, we propose four optimization techniques based on removing redundant nodes, removing identical nodes, removing chain nodes, and making use of decomposition based on the biconnected components of the input graph. We test our techniques on a collection of real-world graphs for the time taken and the average error percentage. We further analyze the applicability of our techniques on various classes of real-world graphs. We suggest why certain techniques work better on certain classes of graphs.
更多查看译文
关键词
closeness centrality,parallel,real world graphs,estimation
AI 理解论文
溯源树
样例
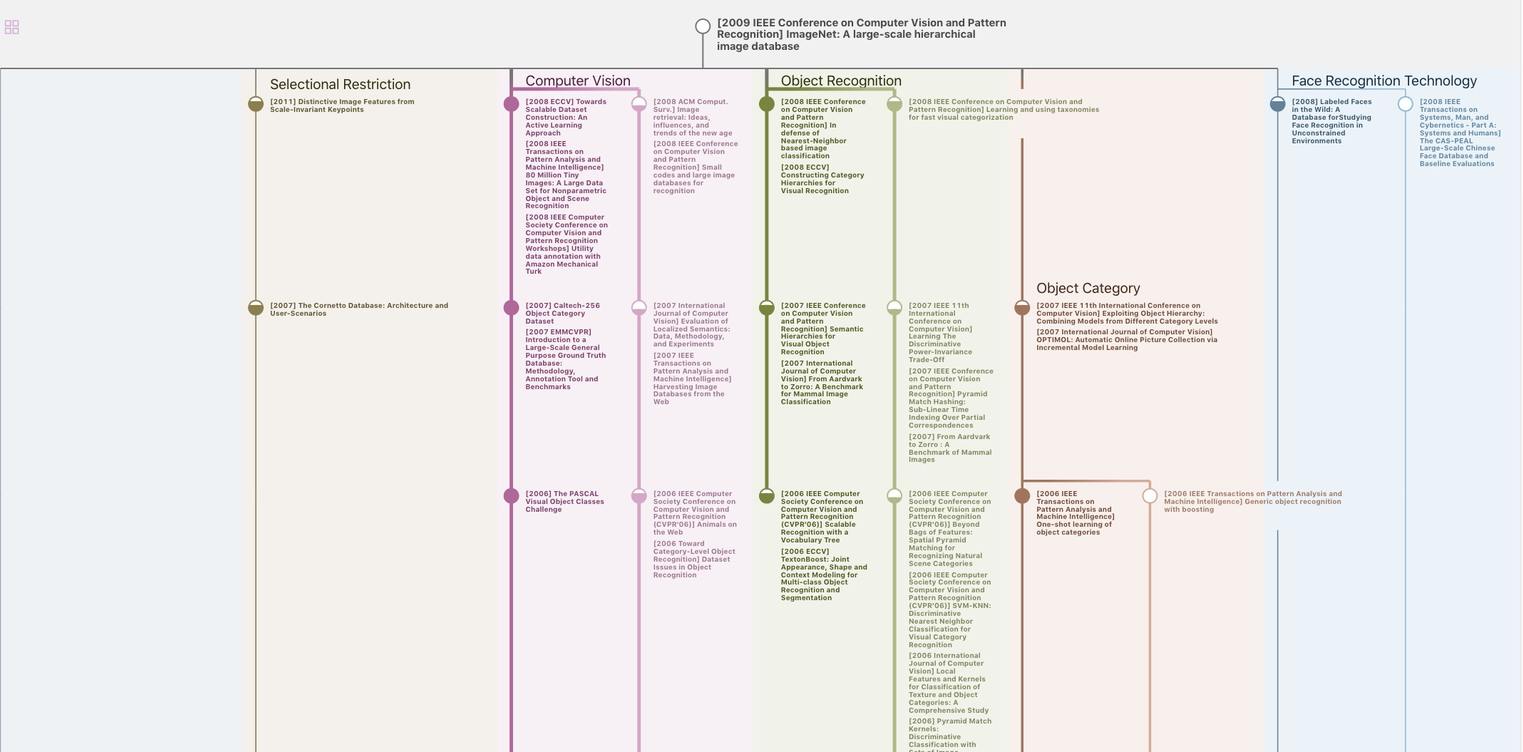
生成溯源树,研究论文发展脉络
Chat Paper
正在生成论文摘要