AutoML: A survey of the state-of-the-art
Knowledge-Based Systems(2021)
摘要
Deep learning (DL) techniques have obtained remarkable achievements on various tasks, such as image recognition, object detection, and language modeling. However, building a high-quality DL system for a specific task highly relies on human expertise, hindering its wide application. Meanwhile, automated machine learning (AutoML) is a promising solution for building a DL system without human assistance and is being extensively studied. This paper presents a comprehensive and up-to-date review of the state-of-the-art (SOTA) in AutoML. According to the DL pipeline, we introduce AutoML methods – covering data preparation, feature engineering, hyperparameter optimization, and neural architecture search (NAS) – with a particular focus on NAS, as it is currently a hot sub-topic of AutoML. We summarize the representative NAS algorithms’ performance on the CIFAR-10 and ImageNet datasets and further discuss the following subjects of NAS methods: one/two-stage NAS, one-shot NAS, joint hyperparameter and architecture optimization, and resource-aware NAS. Finally, we discuss some open problems related to the existing AutoML methods for future research.
更多查看译文
关键词
Deep learning,Automated machine learning (autoML),Neural architecture search (NAS),Hyperparameter optimization (HPO)
AI 理解论文
溯源树
样例
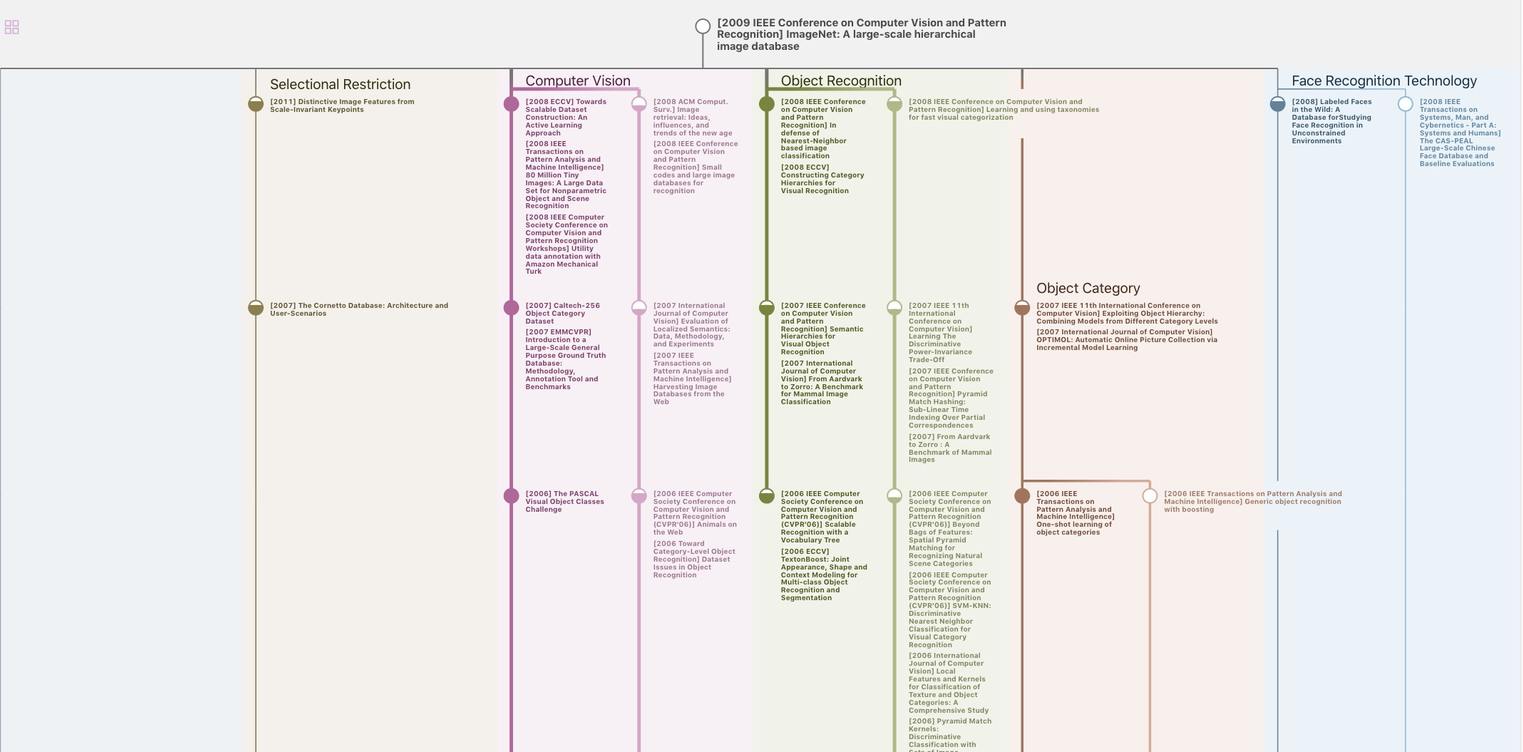
生成溯源树,研究论文发展脉络
Chat Paper
正在生成论文摘要