Whole-lesion histogram and texture analyses of breast lesions on inline quantitative DCE mapping with CAIPIRINHA-Dixon-TWIST-VIBE
European radiology(2019)
摘要
Purpose To investigate the diagnostic capability of whole-lesion (WL) histogram and texture analysis of dynamic contrast-enhanced (DCE) MRI inline-generated quantitative parametric maps using CAIPIRINHA-Dixon-TWIST-VIBE (CDTV) to differentiate malignant from benign breast lesions and breast cancer subtypes. Materials and methods From February 2018 to November 2018, DCE MRI using CDTV was performed on 211 patients. The inline-generated parametric maps included K trans , k ep , V e , and IAUGC 60 . Histogram and texture features were extracted from the above parametric maps respectively based on a WL analysis. Student’s t tests, one-way ANOVAs, Mann-Whitney U tests, Jonckheere-Terpstra tests, and ROC curves were used for statistical analysis. Results Compared with benign breast lesions, malignant breast lesions showed significantly higher K trans _median, 5th percentile, entropy, and diff-entropy , IAUGC 60_median, 5th percentile, entropy, and diff-entropy , k ep_mean, median, 5th percentile, entropy, and diff-entropy , and V e_95th percentile, diff-variance, and contrast , and significantly lower k ep_skewness and V e_SD, entropy, diff-entropy, and skewness (all p ≤ 0.011). The combination of all the extracted parameters yielded an AUC of 0.85 (sensitivity 76%, specificity 86%). k ep_contrast showed a significant difference among different subtypes of breast cancer ( p = 0.006). k ep_skewness showed a significant difference between lymph node–positive and lymph node–negative breast cancer ( p = 0.007). The IAGC 60_5th percentile had an AUC of 0.71 (sensitivity 50%, specificity 91%) for differentiating between high- and low-proliferation groups of breast cancer. Conclusions The WL histogram and texture analyses of CDTV-DCE-derived parameters may give additional information for further evaluation of breast cancer. Key Points • Inline DCE mapping with CDTV is effective and time-saving. • WL histogram and texture-extracted features could distinguish breast cancer from benign lesions accurately. • k ep_contrast , k ep_skewness , and IAUGC 60_5th percentile could predict breast cancer subtypes, lymph node metastasis, and proliferation abilities, respectively.
更多查看译文
关键词
Breast neoplasm,Dynamic,Pharmacokinetics,Magnetic resonance imaging
AI 理解论文
溯源树
样例
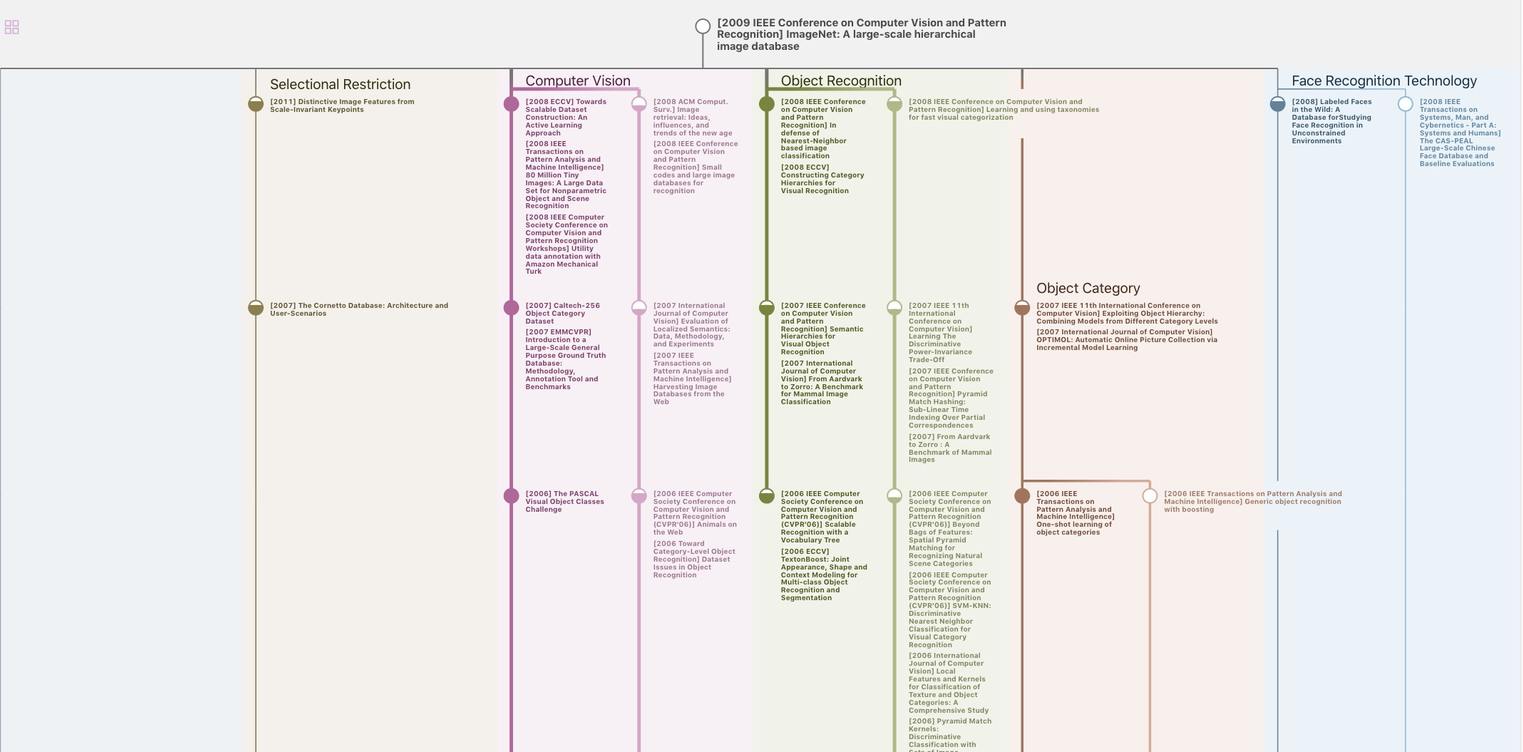
生成溯源树,研究论文发展脉络
Chat Paper
正在生成论文摘要