Administrative database as a source for assessment of systemic lupus erythematosus prevalence: Estonian experience
BMC RHEUMATOLOGY(2019)
摘要
Background Administrative database research is widely applied in the field of epidemiology. However, the results of the studies depend on the type of database used and the algorithms applied for case ascertainment. The optimal methodology for identifying patients with rheumatic diseases from administrative databases is yet not known. Our aim was to describe an administrative database as a source for estimation of epidemiological characteristics on an example of systemic lupus erythematosus (SLE, ICD-10 code M32) prevalence assessment in the database of the Estonian Health Insurance Fund (EHIF). Methods Code M32 billing episodes were extracted from the EHIF database 2006–2010. For all cases where M32 was assigned by a rheumatologist less than four times during the study period, diagnosis verification process using health care providers’ (HCP) databases was applied. For M32 cases assigned by a rheumatologist four times or more, diagnoses were verified for a randomly selected sample. Results From 677 persons with code M32 assigned in EHIF database, 404 were demonstrated having “true SLE”. The code M32 positive predictive value (PPV) for the whole EHIF database was 60%; PPV varies remarkably by specialty of a physician and repetition of the code assignment. The false positive M32 codes were predominantly initial diagnoses which were not confirmed afterwards; in many cases, a rheumatic condition other than SLE was later diagnosed. Conclusions False positive codes due to tentative diagnoses may be characteristic for conditions with a complicated diagnosis process like SLE and need to be taken into account when performing administrative database research.
更多查看译文
关键词
Administrative database research, Systemic lupus erythematosus, Epidemiology, Positive predictive value, Rheumatic diseases
AI 理解论文
溯源树
样例
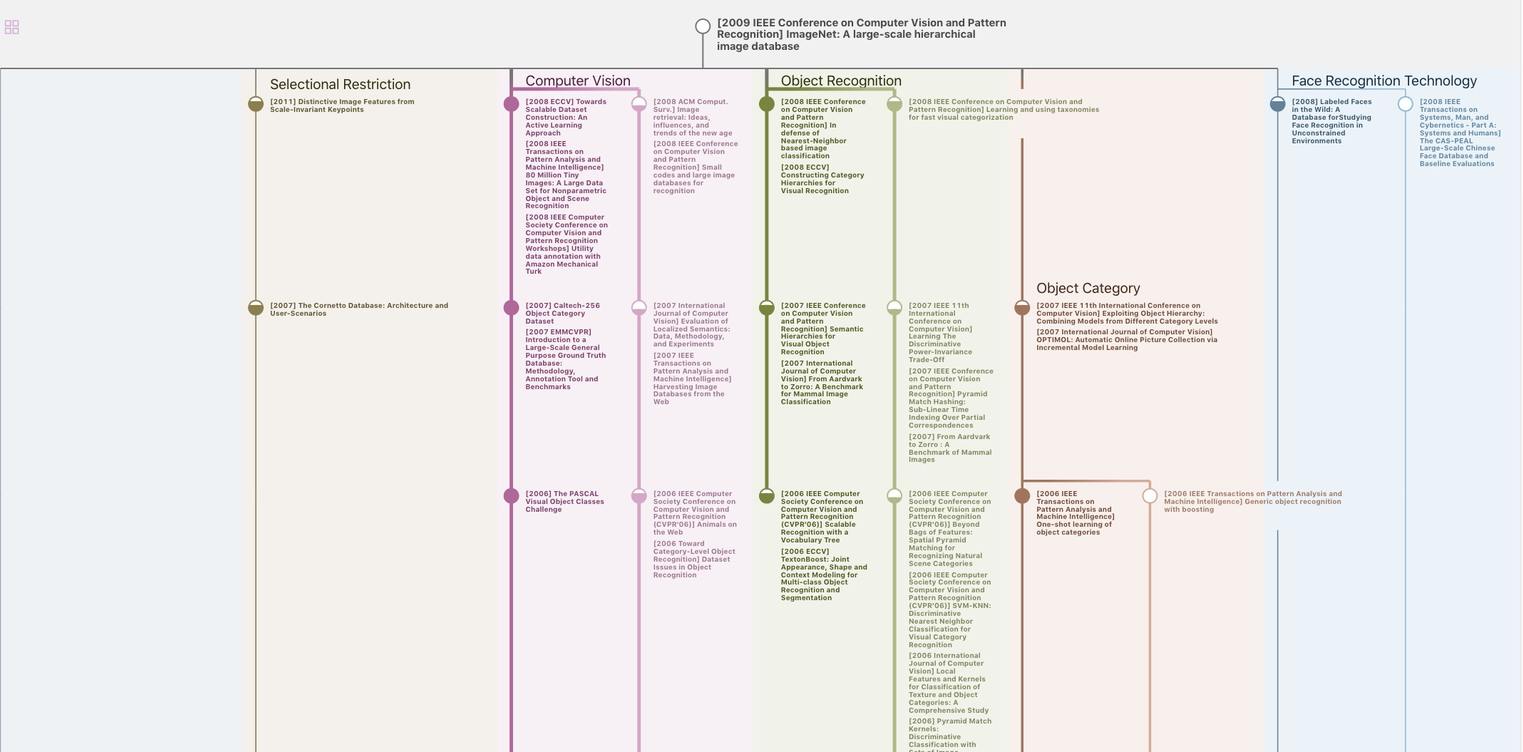
生成溯源树,研究论文发展脉络
Chat Paper
正在生成论文摘要