Learning Invariant Representations for Sentiment Analysis: The Missing Material is Datasets
arxiv(2019)
摘要
Learning representations which remain invariant to a nuisance factor has a great interest in Domain Adaptation, Transfer Learning, and Fair Machine Learning. Finding such representations becomes highly challenging in NLP tasks since the nuisance factor is entangled in a raw text. To our knowledge, a major issue is also that only few NLP datasets allow assessing the impact of such factor. In this paper, we introduce two generalization metrics to assess model robustness to a nuisance factor: \textit{generalization under target bias} and \textit{generalization onto unknown}. We combine those metrics with a simple data filtering approach to control the impact of the nuisance factor on the data and thus to build experimental biased datasets. We apply our method to standard datasets of the literature (\textit{Amazon} and \textit{Yelp}). Our work shows that a simple text classification baseline (i.e., sentiment analysis on reviews) may be badly affected by the \textit{product ID} (considered as a nuisance factor) when learning the polarity of a review. The method proposed is generic and applicable as soon as the nuisance variable is annotated in the dataset.
更多查看译文
关键词
sentiment analysis,representations,datasets,learning
AI 理解论文
溯源树
样例
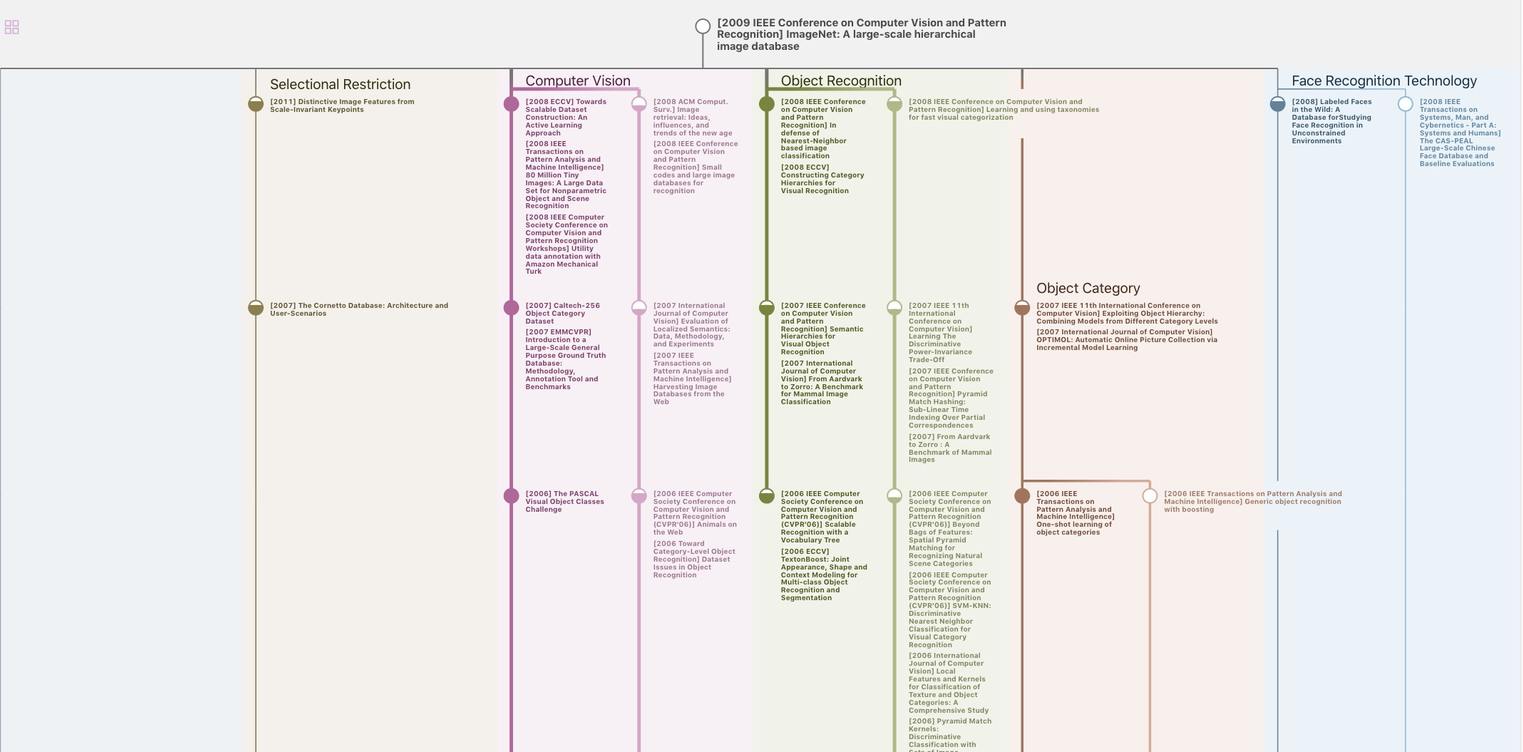
生成溯源树,研究论文发展脉络
Chat Paper
正在生成论文摘要