Towards Sustainable Dairy Management - A Machine Learning Enhanced Method for Estrus Detection
KDD '19: The 25th ACM SIGKDD Conference on Knowledge Discovery and Data Mining Anchorage AK USA August, 2019(2019)
摘要
Our research tackles the challenge of milk production resource use efficiency in dairy farms with machine learning methods. Reproduction is a key factor for dairy farm performance since cows milk production begin with the birth of a calf. Therefore, detecting estrus, the only period when the cow is susceptible to pregnancy, is crucial for farm efficiency. Our goal is to enhance estrus detection (performance, interpretability), especially on the currently undetected silent estrus (35% of total estrus), and allow farmers to rely on automatic estrus detection solutions based on affordable data (activity, temperature). In this paper, we first propose a novel approach with real-world data analysis to address both behavioral and silent estrus detection through machine learning methods. Second, we present LCE, a local cascade based algorithm that significantly outperforms a typical commercial solution for estrus detection, driven by its ability to detect silent estrus. Then, our study reveals the pivotal role of activity sensors deployment in estrus detection. Finally, we propose an approach relying on global and local (behavioral versus silent) algorithm interpretability (SHAP) to reduce the mistrust in estrus detection solutions.
更多查看译文
关键词
classification, interpretability, machine learning, sustainable dairy management
AI 理解论文
溯源树
样例
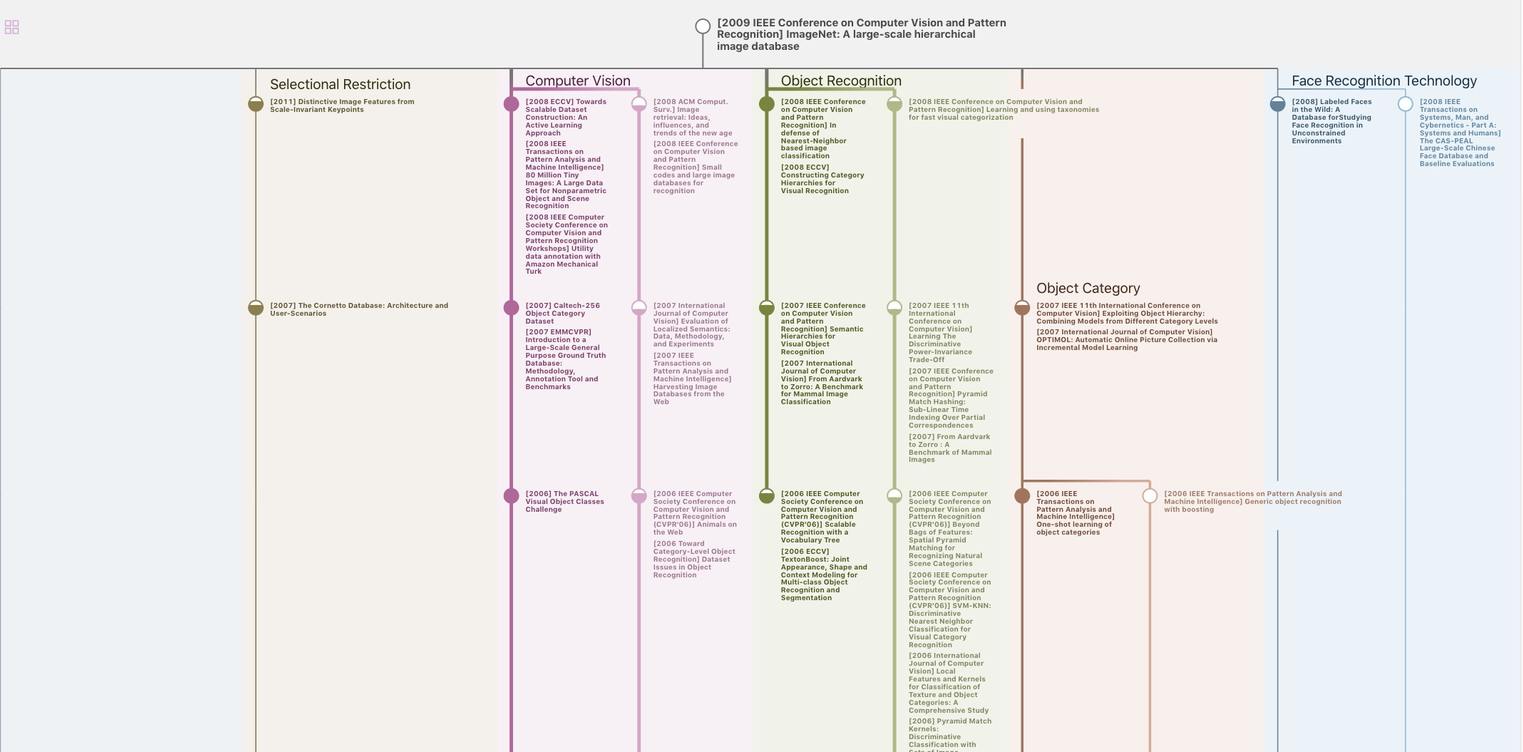
生成溯源树,研究论文发展脉络
Chat Paper
正在生成论文摘要