Smart Roles: Inferring Professional Roles in Email Networks
KDD '19: The 25th ACM SIGKDD Conference on Knowledge Discovery and Data Mining Anchorage AK USA August, 2019(2019)
摘要
Email is ubiquitous in the workplace. Naturally, machine learning models that make third-party email clients "smarter" can dramatically impact employees' productivity and efficiency. Motivated by this potential, we study the task of professional role inference from email data, which is crucial for email prioritization and contact recommendation systems. The central question we address is: Given limited data about employees, as is common in third-party email applications, can we infer where in the organizational hierarchy these employees belong based on their email behavior? Toward our goal, in this paper we study professional role inference on a unique new email dataset comprising billions of email exchanges across thousands of organizations. Taking a network approach in which nodes are employees and edges represent email communication, we propose EMBER, or EMBedding Email-based Roles, which finds email-centric embeddings of network nodes to be used in professional role inference tasks. EMBER automatically captures behavioral similarity between employees in the email network, leading to embeddings that naturally distinguish employees of different hierarchical roles. EMBER often outperforms the state-of-the-art by 2-20% in role inference accuracy and 2.5-344x in speed. We also use EMBER with our unique dataset to study how inferred professional roles compare between organizations of different sizes and sectors, gaining new insights into organizational hierarchy.
更多查看译文
关键词
email exchange networks, hierarchical roles, professional roles, representation learning, role inference, structural embeddings
AI 理解论文
溯源树
样例
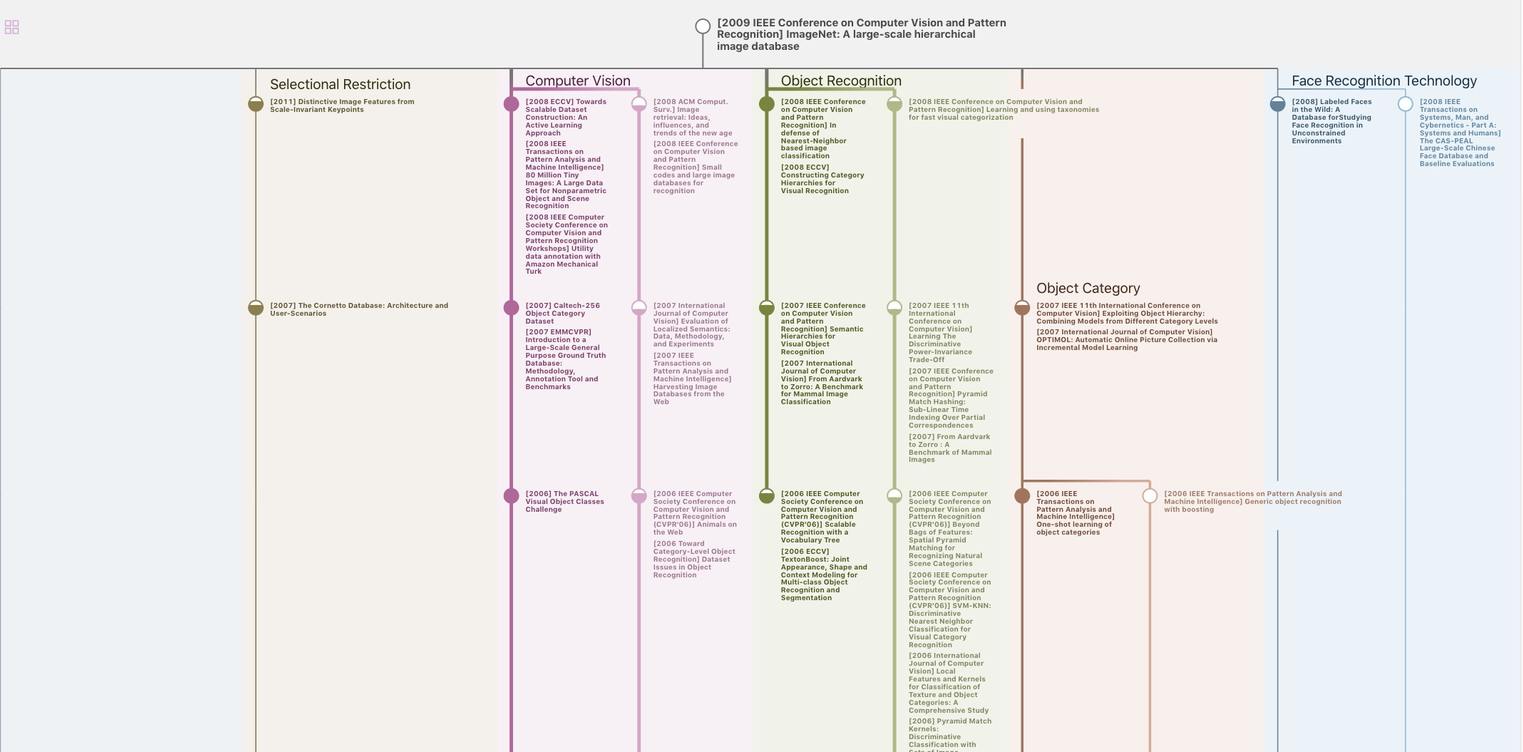
生成溯源树,研究论文发展脉络
Chat Paper
正在生成论文摘要