Generative Models For Graph-Based Protein Design
ADVANCES IN NEURAL INFORMATION PROCESSING SYSTEMS 32 (NIPS 2019)(2019)
摘要
Engineered proteins offer the potential to solve many problems in biomedicine, energy, and materials science, but creating designs that succeed is difficult in practice. A significant aspect of this challenge is the complex coupling between protein sequence and 3D structure, with the task of finding a viable design often referred to as the inverse protein folding problem. In this work, we introduce a conditional generative model for protein sequences given 3D structures based on graph representations. Our approach efficiently captures the complex dependencies in proteins by focusing on those that are long-range in sequence but local in 3D space. This graph-based approach improves in both speed and reliability over conventional and other neural network-based approaches, and takes a step toward rapid and targeted biomolecular design with the aid of deep generative models.
更多查看译文
关键词
3d space
AI 理解论文
溯源树
样例
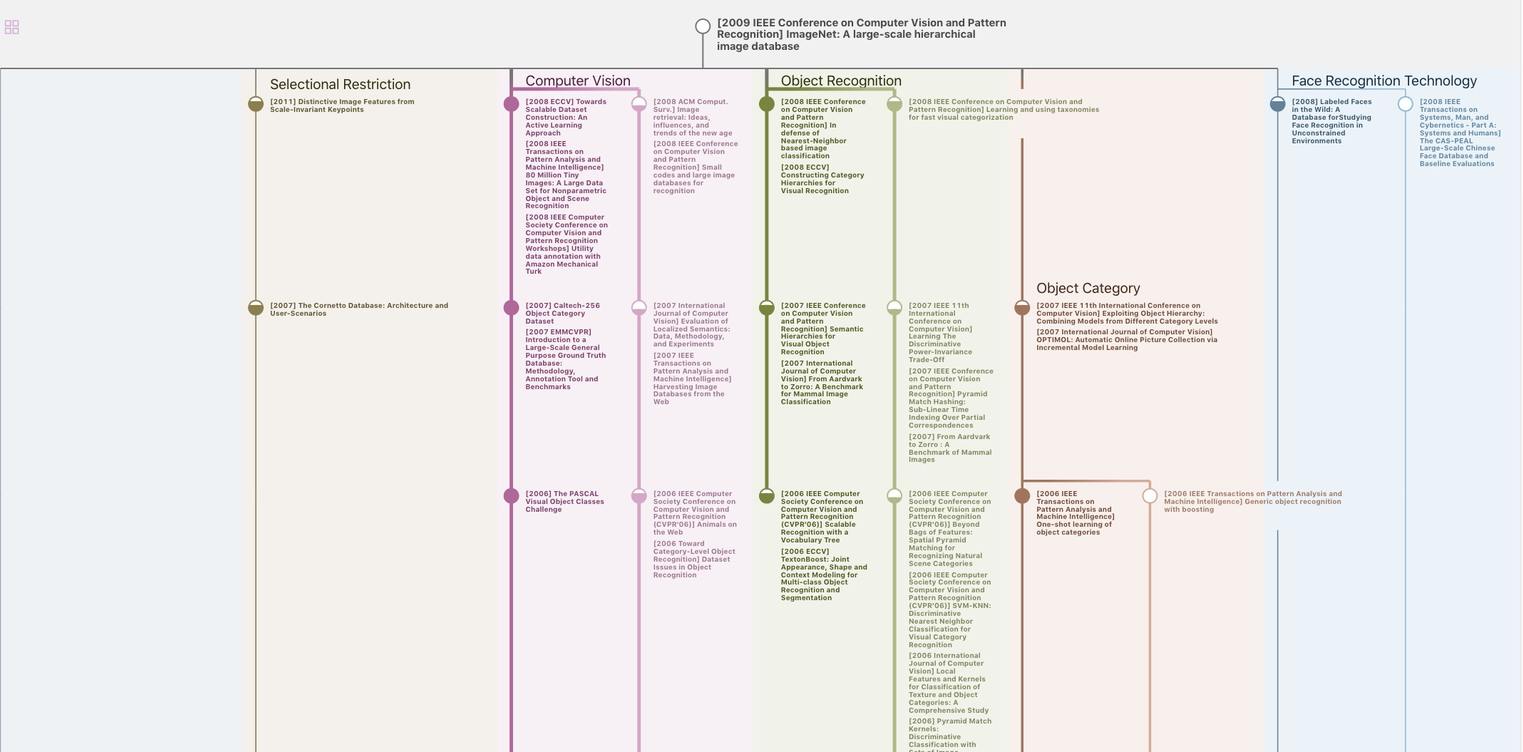
生成溯源树,研究论文发展脉络
Chat Paper
正在生成论文摘要