Dynamic Bayesian Approach for decision-making in Ego-Things
2019 IEEE 5TH WORLD FORUM ON INTERNET OF THINGS (WF-IOT)(2020)
摘要
This paper presents a novel approach to detect abnormalities in dynamic systems based on multisensory data and feature selection. The proposed method produces multiple inference models by considering several features of the observed data. This work facilitates the obtainment of the most precise features for predicting future instances and detecting abnormalities. Growing neural gas (GNG) is employed for clustering multisensory data into a set of nodes that provide a semantic interpretation of data and define local linear models for prediction purposes. Our method uses a Markov Jump particle filter (MJPF) for state estimation and abnormality detection. The proposed method can be used for selecting the optimal set features to be shared in networking operations such that state prediction, decision-making, and abnormality detection processes are favored. This work is evaluated by using a real dataset consisting of a moving vehicle performing some tasks in a controlled environment.
更多查看译文
关键词
Feature selection, Abnormality detection, multisensory data, particle filter, Kalman filter
AI 理解论文
溯源树
样例
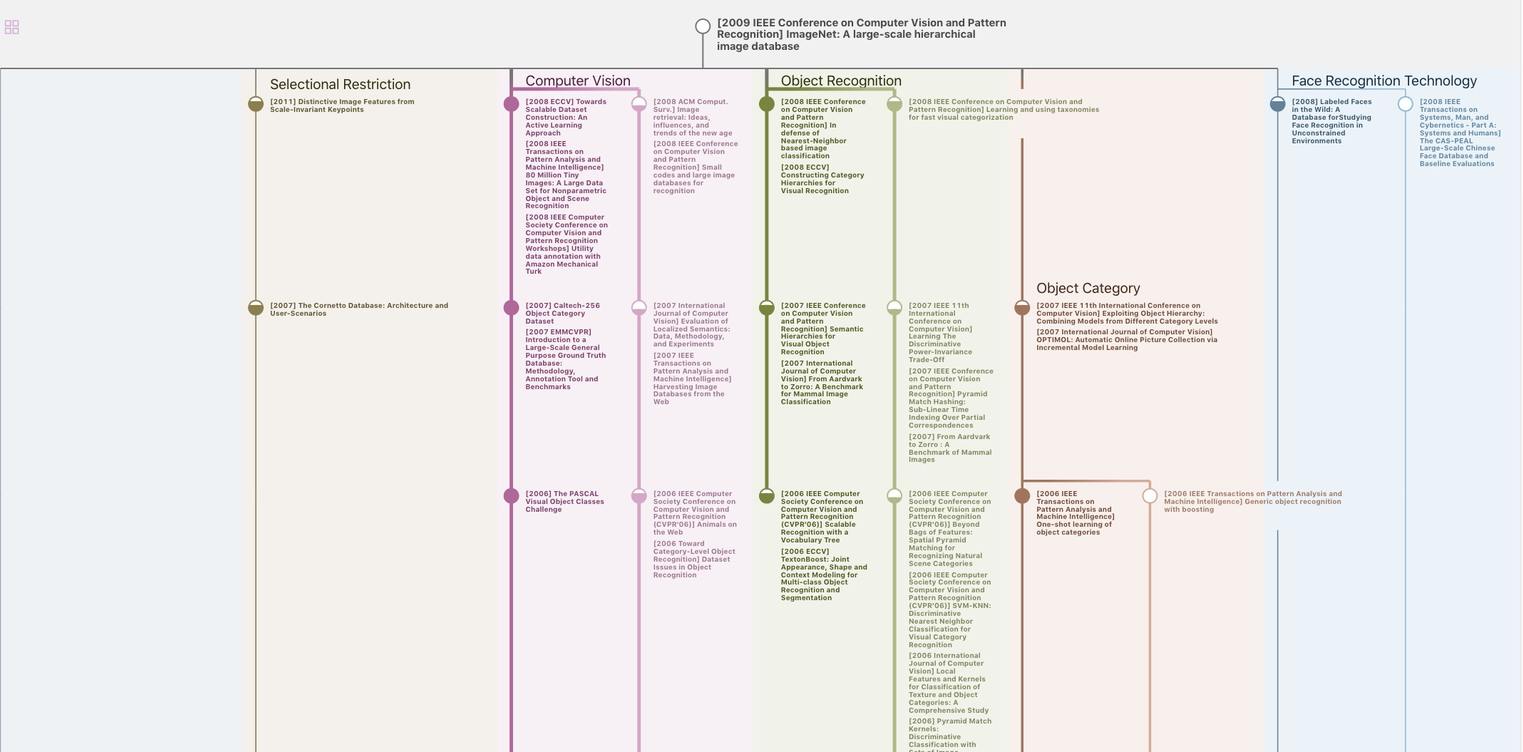
生成溯源树,研究论文发展脉络
Chat Paper
正在生成论文摘要