Multilayer feature descriptors fusion CNN models for fine-grained visual recognition.
COMPUTER ANIMATION AND VIRTUAL WORLDS(2019)
摘要
Fine-grained image classification is a challenging topic in the field of computer vision. General models based on first-order local features cannot achieve acceptable performance because the features are not so efficient in capturing fine-grained difference. A bilinear convolutional neural network (CNN) model exhibits that a second-order statistical feature is more efficient in capturing fine-grained difference than a first-order local feature. However, this framework only considers the extraction of a second-order feature descriptor, using a single convolutional layer. The potential effective classification features of other convolutional layers are ignored, resulting in loss of recognition accuracy. In this paper, a multilayer feature descriptors fusion CNN model is proposed. It fully considers the second-order feature descriptors and the first-order local feature descriptor generated by different layers. Experimental verification was carried out on fine-grained classification benchmark data sets, CUB-200-2011, Stanford Cars, and FGVC-aircraft. Compared with the bilinear CNN model, the proposed method has improved accuracy by 0.8%, 1.1%, and 5.5%. Compared with the compact bilinear pooling model, there is an accuracy increase of 0.64%, 1.63%, and 1.45%, respectively. In addition, the proposed model effectively uses multiple 1x1 convolution kernels to reduce dimension. The experimental results show that the multilayer low-dimensional second-order feature descriptors fusion model has comparable recognition accuracy of the original model.
更多查看译文
关键词
convolutional neural network,deep learning,dimensionality reduction,fine-grained image classification,multilayer feature descriptors
AI 理解论文
溯源树
样例
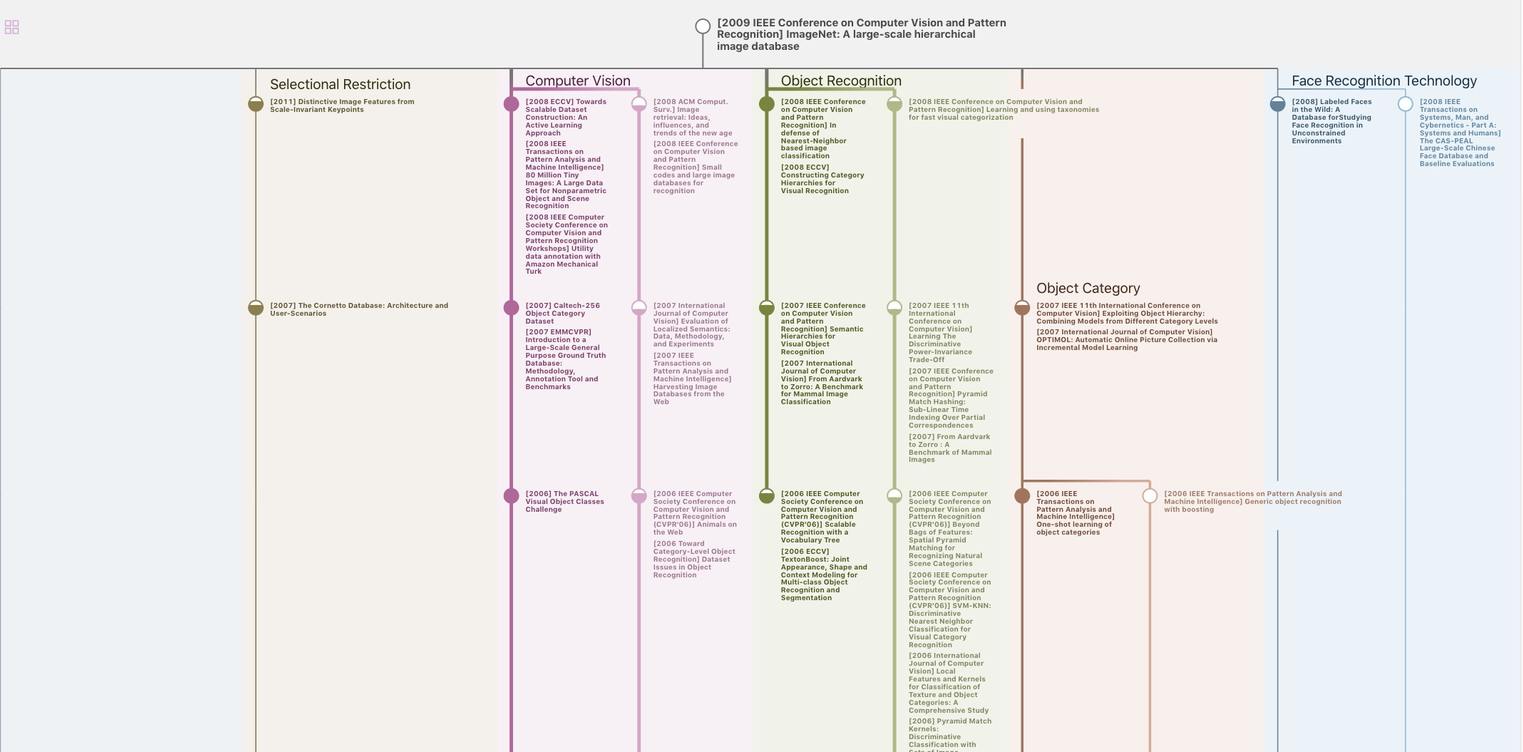
生成溯源树,研究论文发展脉络
Chat Paper
正在生成论文摘要