Channel Estimation and Equalization based on Deep BLSTM for FBMC-OQAM Systems
ICC 2019 - 2019 IEEE INTERNATIONAL CONFERENCE ON COMMUNICATIONS (ICC)(2019)
摘要
Channel estimation and equalization is one of the challenges of the filter bank multicarrier (FBMC) systems because of the existence of intrinsic imaginary interference. In this paper, we try to solve this challenge from a learning-based perspective. Based on the study of the intrinsic relationship between bidirectional long short-term memory (BLSTM) recurrent neural networks (RNN) and the FBMC radio signals, we propose a novel deep BLSTM network based channel estimation and equalization scheme, abbreviated as BLSTM-CE scheme. In the BLSTM-CE scheme, the input and output of some traditional independent modules are seen as an unknown nonlinear mapping and use a deep BLSTM network to approximate it. Numerical simulation shows that our proposed BLSTM-CE scheme can obtain perfect channel estimation performance in the FBMC systems.
更多查看译文
关键词
FBMC,channel estimation,recurrent neural networks,long short-term memory,deep learning
AI 理解论文
溯源树
样例
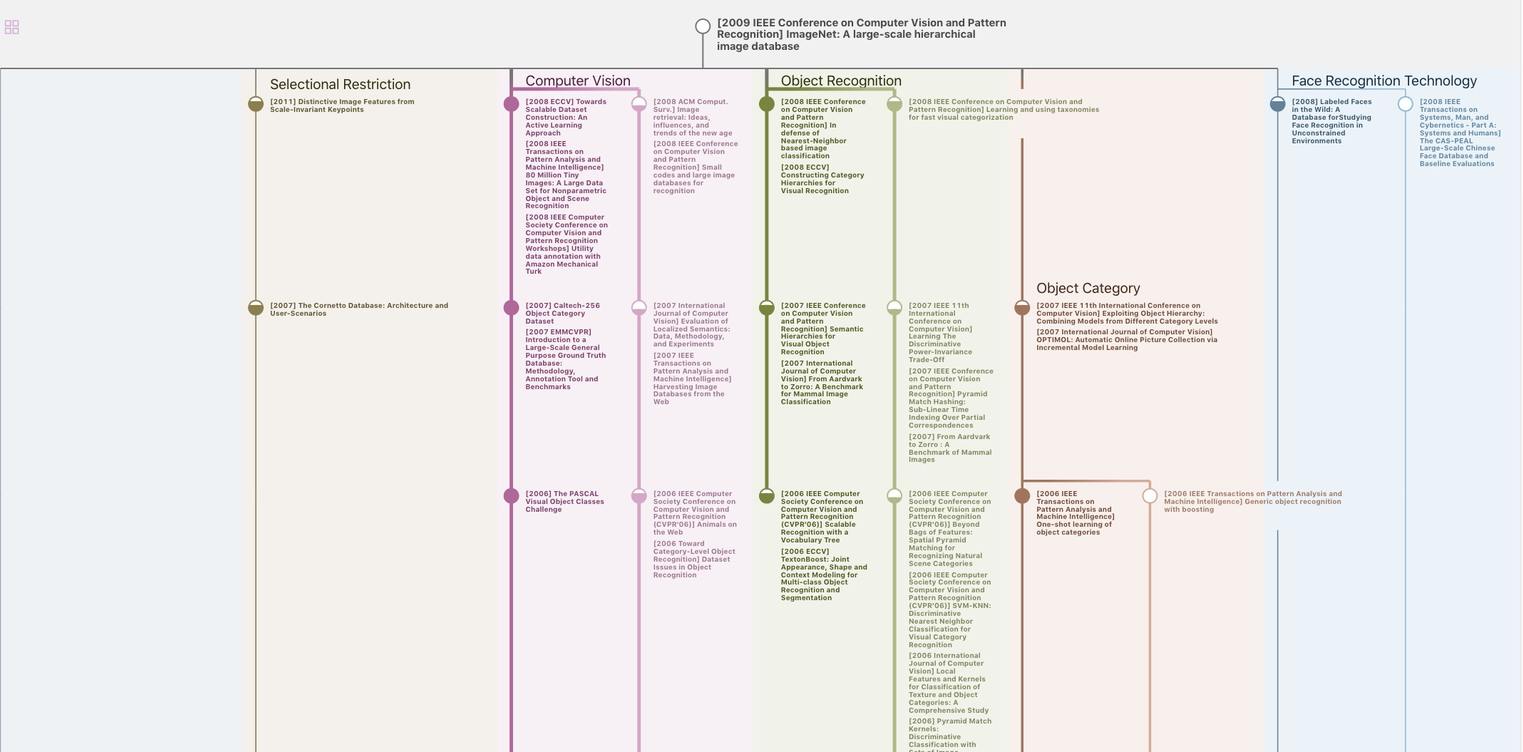
生成溯源树,研究论文发展脉络
Chat Paper
正在生成论文摘要