Cap2det: Learning To Amplify Weak Caption Supervision For Object Detection
2019 IEEE/CVF INTERNATIONAL CONFERENCE ON COMPUTER VISION (ICCV 2019)(2019)
摘要
Learning to localize and name object instances is a fundamental problem in vision, but state-of-the-art approaches rely on expensive bounding box supervision. While weakly supervised detection (WSOD) methods relax the need for boxes to that of image-level annotations, even cheaper supervision is naturally available in the form of unstructured textual descriptions that users may freely provide when uploading image content. However, straightforward approaches to using such data for WSOD wastefully discard captions that do not exactly match object names. Instead, we show how to squeeze the most information out of these captions by training a text-only classifier that generalizes beyond dataset boundaries. Our discovery provides an opportunity for learning detection models from noisy but more abundant and freely-available caption data. We also validate our model on three classic object detection benchmarks and achieve state-of-the-art WSOD performance. Our code is available at https:// github. com/yekeren/Cap2Det.
更多查看译文
关键词
bounding box supervision,weakly supervised detection methods,image-level annotations,unstructured textual descriptions,image content,freely-available caption data,object detection,WSOD performance,Cap2Det,weak caption supervision amplification learning,text-only classifier training
AI 理解论文
溯源树
样例
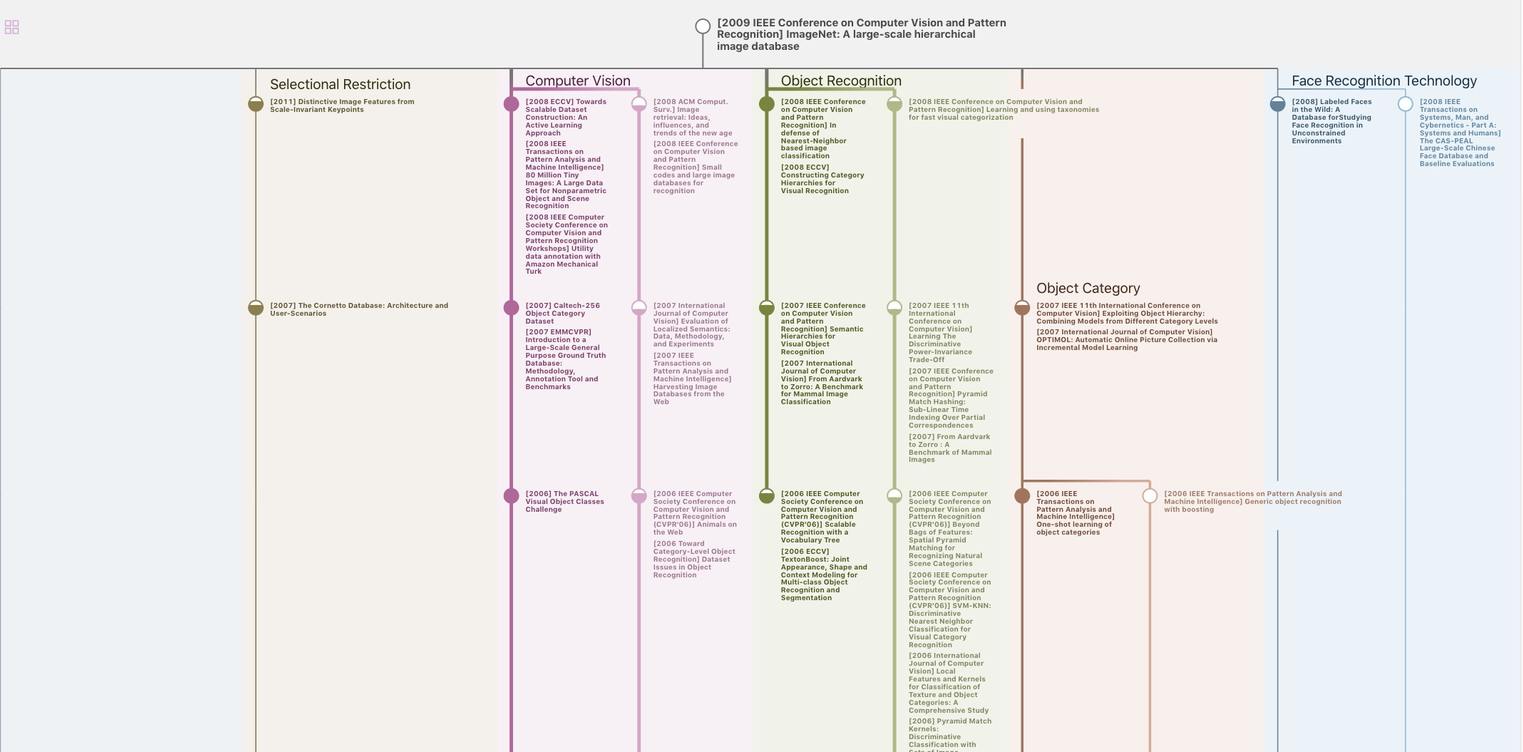
生成溯源树,研究论文发展脉络
Chat Paper
正在生成论文摘要