GraphX$$^\mathbf{\small NET } -$$ Chest X-Ray Classification Under Extreme Minimal Supervision
arxiv(2019)
摘要
The task of classifying X-ray data is a problem of both theoretical and clinical interest. Whilst supervised deep learning methods rely upon huge amounts of labelled data, the critical problem of achieving a good classification accuracy when an extremely small amount of labelled data is available has yet to be tackled. In this work, we introduce a novel semi-supervised framework for X-ray classification which is based on a graph-based optimisation model. To the best of our knowledge, this is the first method that exploits graph-based semi-supervised learning for X-ray data classification. Furthermore, we introduce a new multi-class classification functional with carefully selected class priors which allows for a smooth solution that strengthens the synergy between the limited number of labels and the huge amount of unlabelled data. We demonstrate, through a set of numerical and visual experiments, that our method produces highly competitive results on the ChestX-ray14 data set whilst drastically reducing the need for annotated data.
更多查看译文
关键词
Semi-supervised learning,Classification,Chest X-Ray,Graphs,Transductive learning
AI 理解论文
溯源树
样例
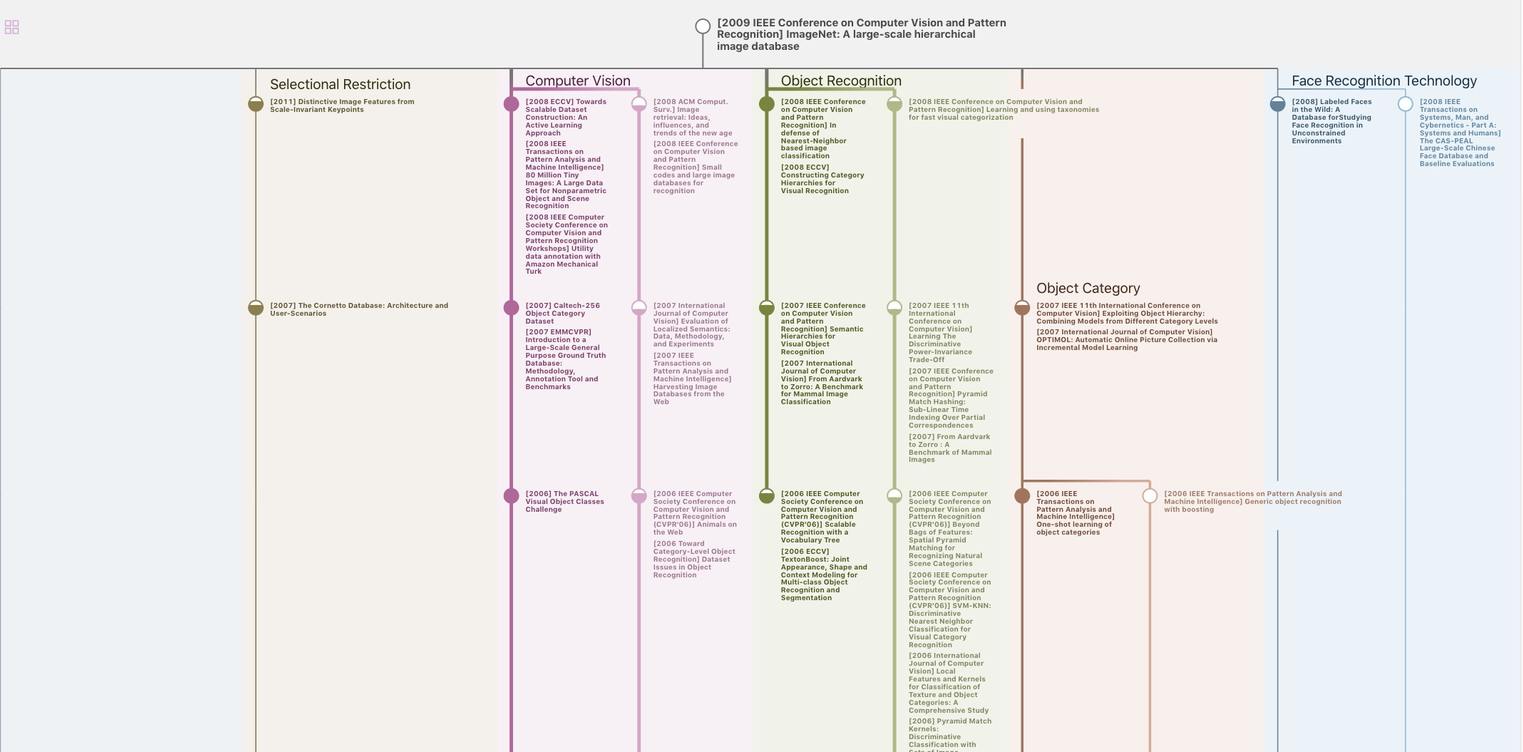
生成溯源树,研究论文发展脉络
Chat Paper
正在生成论文摘要