Toxicity Prediction by Multimodal Deep Learning
CoRR(2019)
摘要
Prediction of toxicity levels of chemical compounds is an important issue in Quantitative Structure-Activity Relationship (QSAR) modeling. Although toxicity prediction has achieved significant progress in recent times through deep learning, prediction accuracy levels obtained by even very recent methods are not yet very high. We propose a multimodal deep learning method using multiple heterogeneous neural network types and data representations. We represent chemical compounds by strings, images, and numerical features. We train fully connected, convolutional, and recurrent neural networks and their ensembles. Each data representation or neural network type has its own strengths and weaknesses. Our motivation is to obtain a collective performance that could go beyond individual performance of each data representation or each neural network type. On a standard toxicity benchmark, our proposed method obtains significantly better accuracy levels than that by the state-of-the-art toxicity prediction methods.
更多查看译文
关键词
Molecular activities,Toxicity prediction,Deep learning
AI 理解论文
溯源树
样例
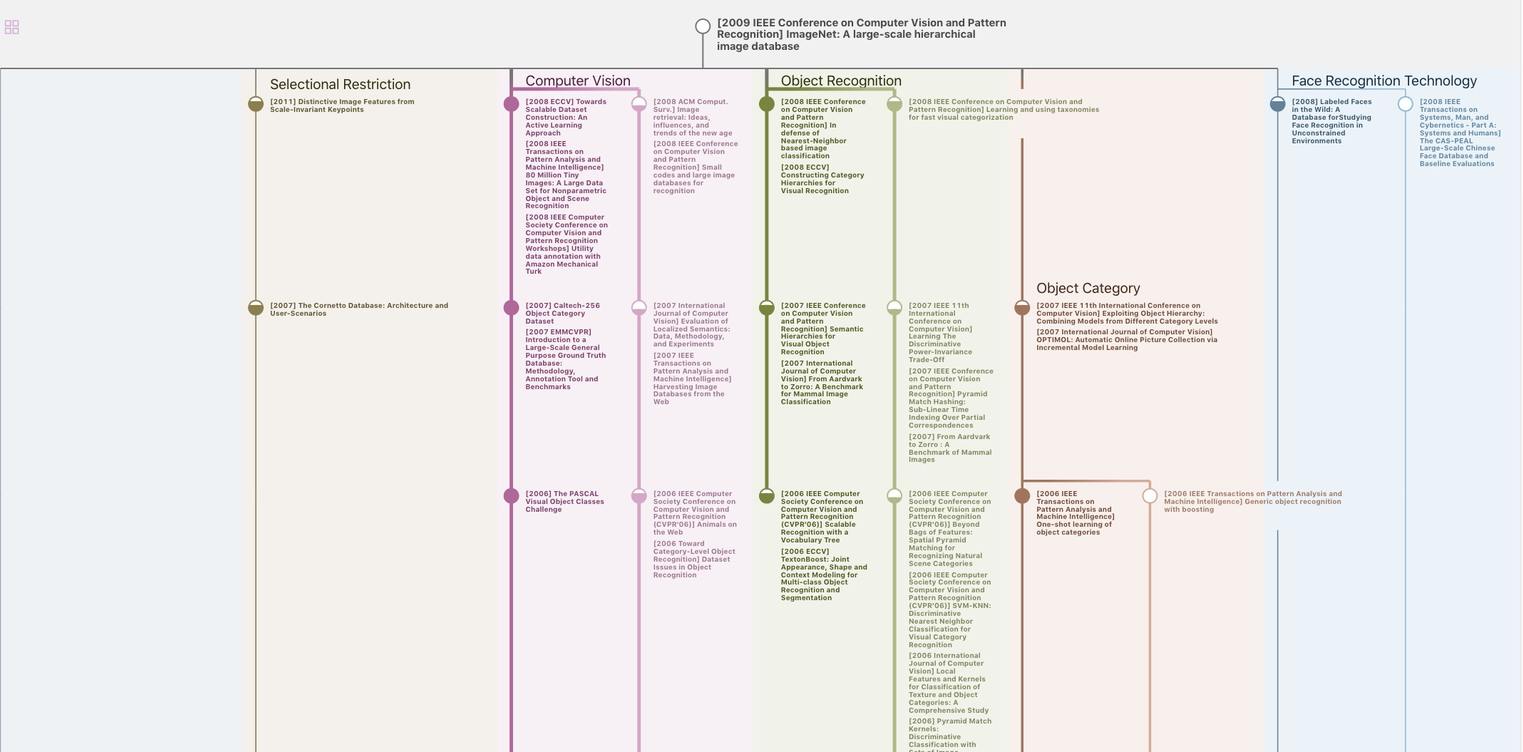
生成溯源树,研究论文发展脉络
Chat Paper
正在生成论文摘要