Pose-Guided Spatial Alignment and Key Frame Selection for One-Shot Video-Based Person Re-Identification.
IEEE ACCESS(2019)
摘要
One-shot video-based person re-identification exploits the unlabeled data by using a single-labeled sample for each individual to train a model and to reduce the need for laborious labeling. Although recent works focusing on this task have made some achievements, most state-of-the-art models are vulnerable to misalignment, pose variation and corrupted frames. To address these challenges, we propose a one-shot video-based person re-identification model based on pose-guided spatial alignment and KFS. First, a spatial transformer sub-network trained using pose-guided regression is employed to perform the spatial alignment. Second, we propose a novel training strategy based on KFS. Key frames with abruptly changing poses are deliberately identified and selected to make the network adaptive to pose variation. Finally, we propose a frame feature pooling method by incorporating long short-term memory with an attention mechanism to reduce the influence of corrupted frames. Comprehensive experiments are presented based on the MARS and DukeMTMC-VideoReID datasets. The mAP values for these datasets reach 46.5% and 68.4%, respectively, demonstrating that the proposed model achieves significant improvements over state-of-the-art one-shot person re-identification methods.
更多查看译文
关键词
Person re-identification,one-shot learning,spatial alignment,key frame selection,frame feature pooling
AI 理解论文
溯源树
样例
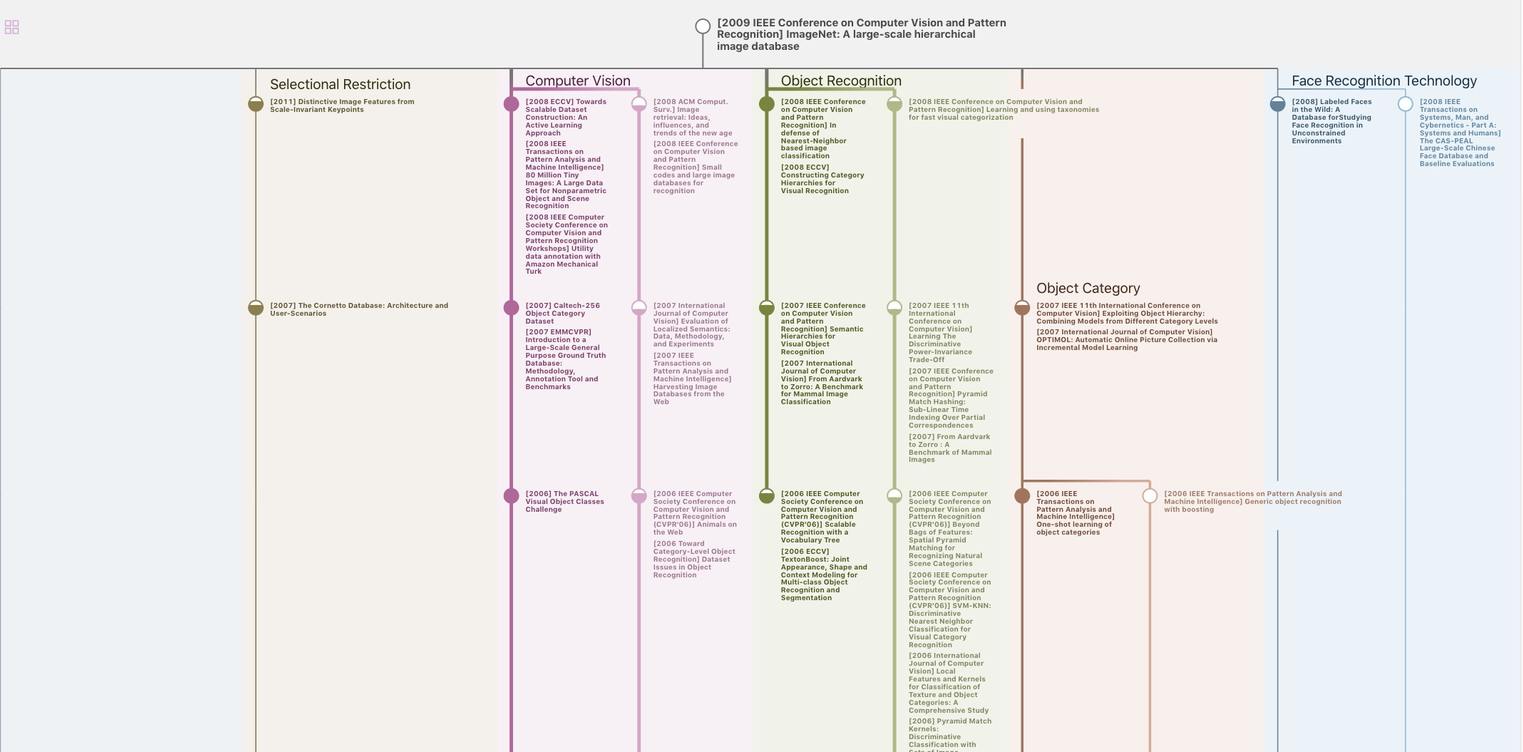
生成溯源树,研究论文发展脉络
Chat Paper
正在生成论文摘要