Learning Interpretable Expression-sensitive Features for 3D Dynamic Facial Expression Recognition
2019 14th IEEE International Conference on Automatic Face & Gesture Recognition (FG 2019)(2019)
摘要
Different facial components carry different amount of information being conveyed for 3D dynamic expression recognition. Hence, identifying facial components that are highly relevant to specific expression changes is crucial for discriminative facial expression recognition. This work aims to learn expression-sensitive features, which are expected to not only yield comparable recognition performance with the state-of-the-art ones, but also can be interpreted by human. Firstly, spatio-temporal features (HOG3D) are extracted from local depth patch-sequences to represent facial expression dynamics. A two-phase feature selection process is then proposed to determine the facial components that can best distinguish the expressions. In order to verify the effectiveness of the resulting facial components, the expression-sensitive features from the corresponding area are fed into a hierarchical classifier for facial expression recognition. The proposed method is evaluated on the BU-4DFE benchmark database, and results show that learned expression-sensitive features can achieve a comparable recognition performance with existing methods. Additionally, the resulting HOG3D features after feature selection can be used to generate semantic interpretation of the expression dynamics.
更多查看译文
关键词
interpretable expression-sensitive features,3D dynamic facial expression recognition,3D dynamic expression recognition,discriminative facial expression recognition,spatio-temporal features,facial expression dynamics,two-phase feature selection process,learned expression-sensitive features,facial components,HOG3D features,local depth patch-sequences,hierarchical classifier,BU-4DFE benchmark database
AI 理解论文
溯源树
样例
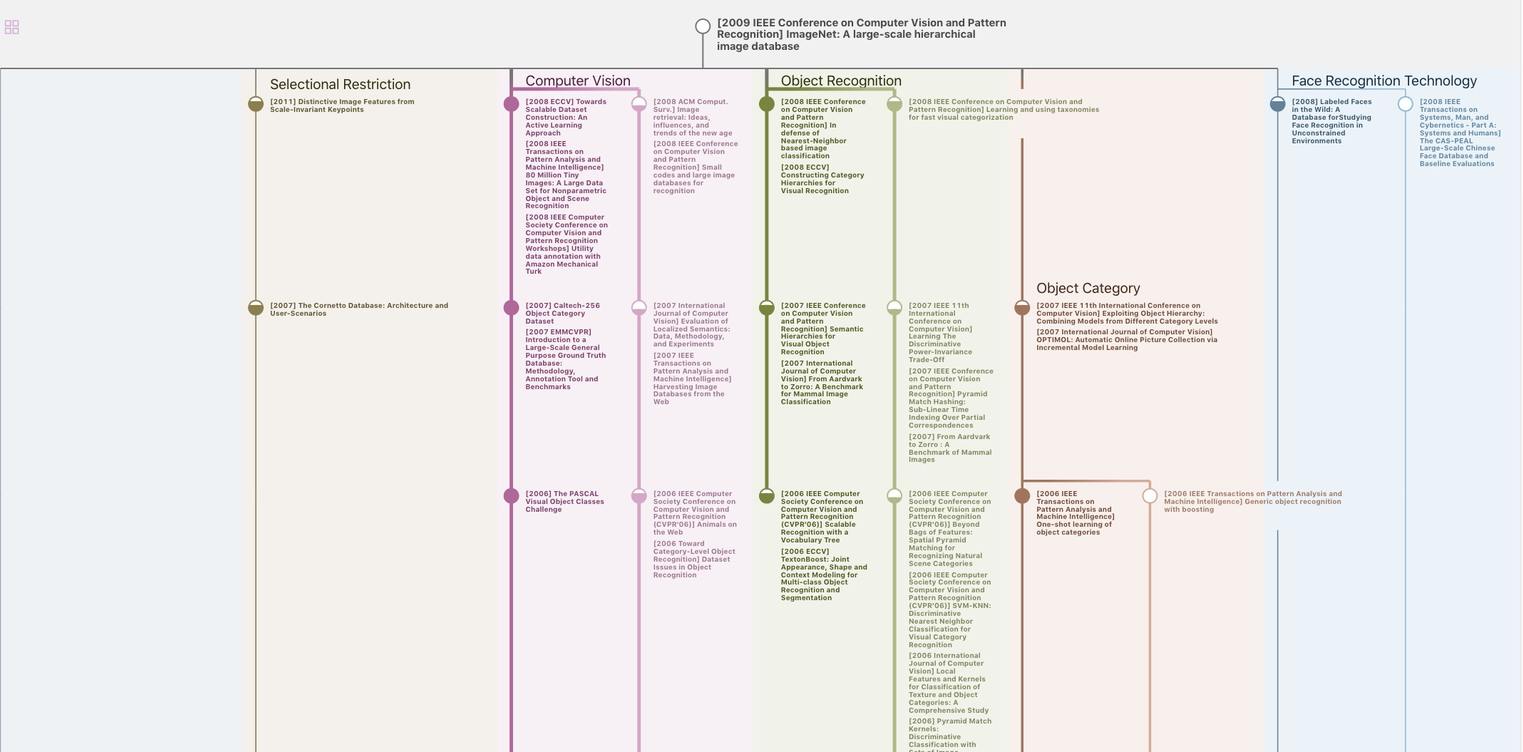
生成溯源树,研究论文发展脉络
Chat Paper
正在生成论文摘要