Adversarial Collaborative Neural Network for Robust Recommendation
Proceedings of the 42nd International ACM SIGIR Conference on Research and Development in Information Retrieval(2019)
摘要
Most of recent neural network(NN)-based recommendation techniques mainly focus on improving the overall performance, such as hit ratio for top-N recommendation, where the users' feedbacks are considered as the ground-truth. In real-world applications, those feedbacks are possibly contaminated by imperfect user behaviours, posing challenges on the design of robust recommendation methods. Some methods apply man-made noises on the input data to train the networks more effectively (e.g. the collaborative denoising auto-encoder). In this work, we propose a general adversarial training framework for NN-based recommendation models, improving both the model robustness and the overall performance. We apply our approach on the collaborative auto-encoder model, and show that the combination of adversarial training and NN-based models outperforms highly competitive state-of-the-art recommendation methods on three public datasets.
更多查看译文
关键词
adversarial learning, deep learning, item recommendation
AI 理解论文
溯源树
样例
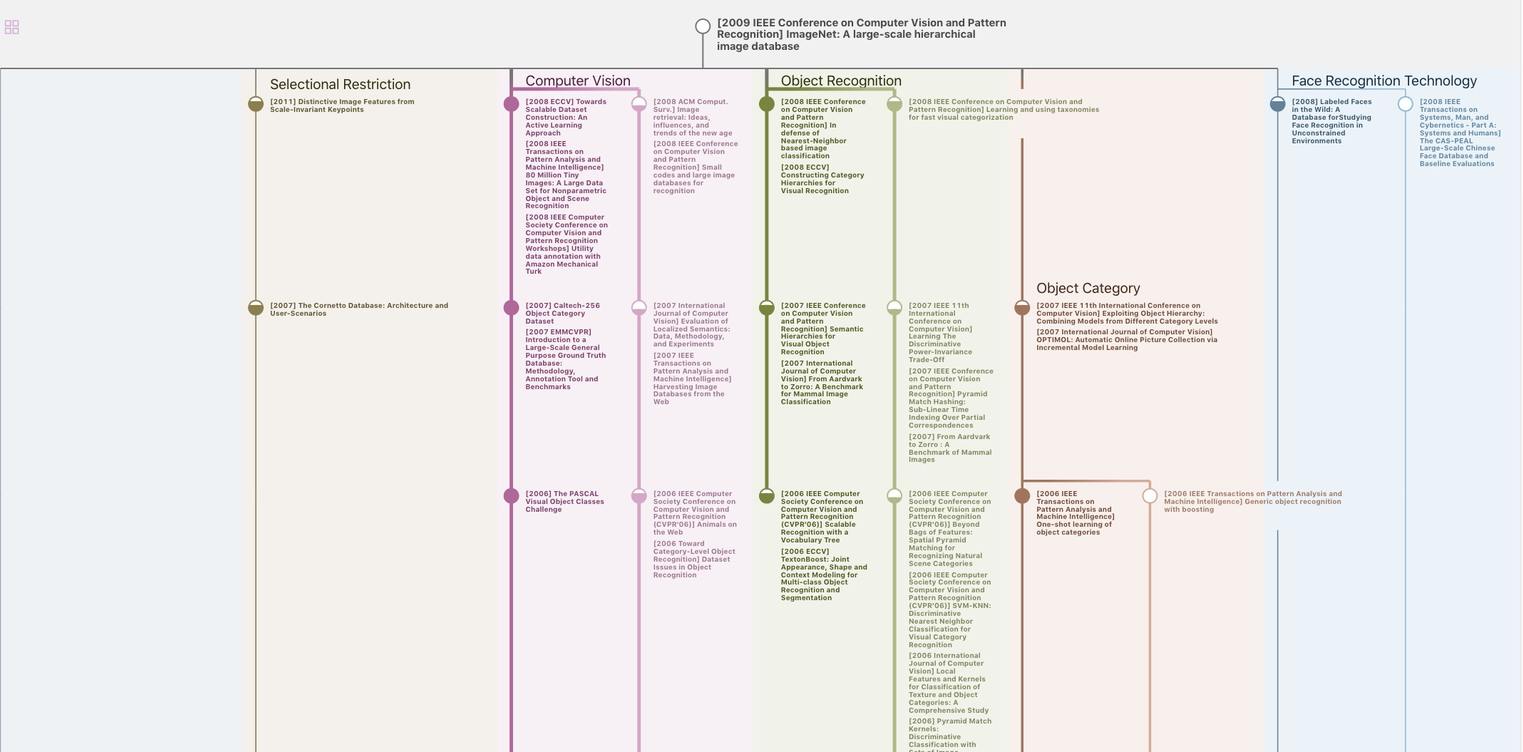
生成溯源树,研究论文发展脉络
Chat Paper
正在生成论文摘要