Social Reinforcement Learning to Combat Fake News Spread.
UAI(2020)
摘要
In this work, we develop a social reinforcement learning approach to combat the spread of fake news. Specifically, we aim to learn an intervention model to promote the spread of true news in a social network—in order to mitigate the impact of fake news. We model news diffusion as a Multivariate Hawkes Process (MHP) and make interventions that are learnt via policy optimization. The key insight is to estimate the response a user will get from the social network upon sharing a post, as it indicates her impact on diffusion, and will thus help in efficient allocation of incentive. User responses also depend on political bias and peer influence, which we model as a second MHP, interleaving it with the news diffusion process. We evaluate our model on semi-synthetic and real-world data. The results demonstrate that our proposed model outperforms other alternatives that do not consider estimates of user responses and political bias when learning how to allocate incentives.
更多查看译文
关键词
spread,news,learning,social
AI 理解论文
溯源树
样例
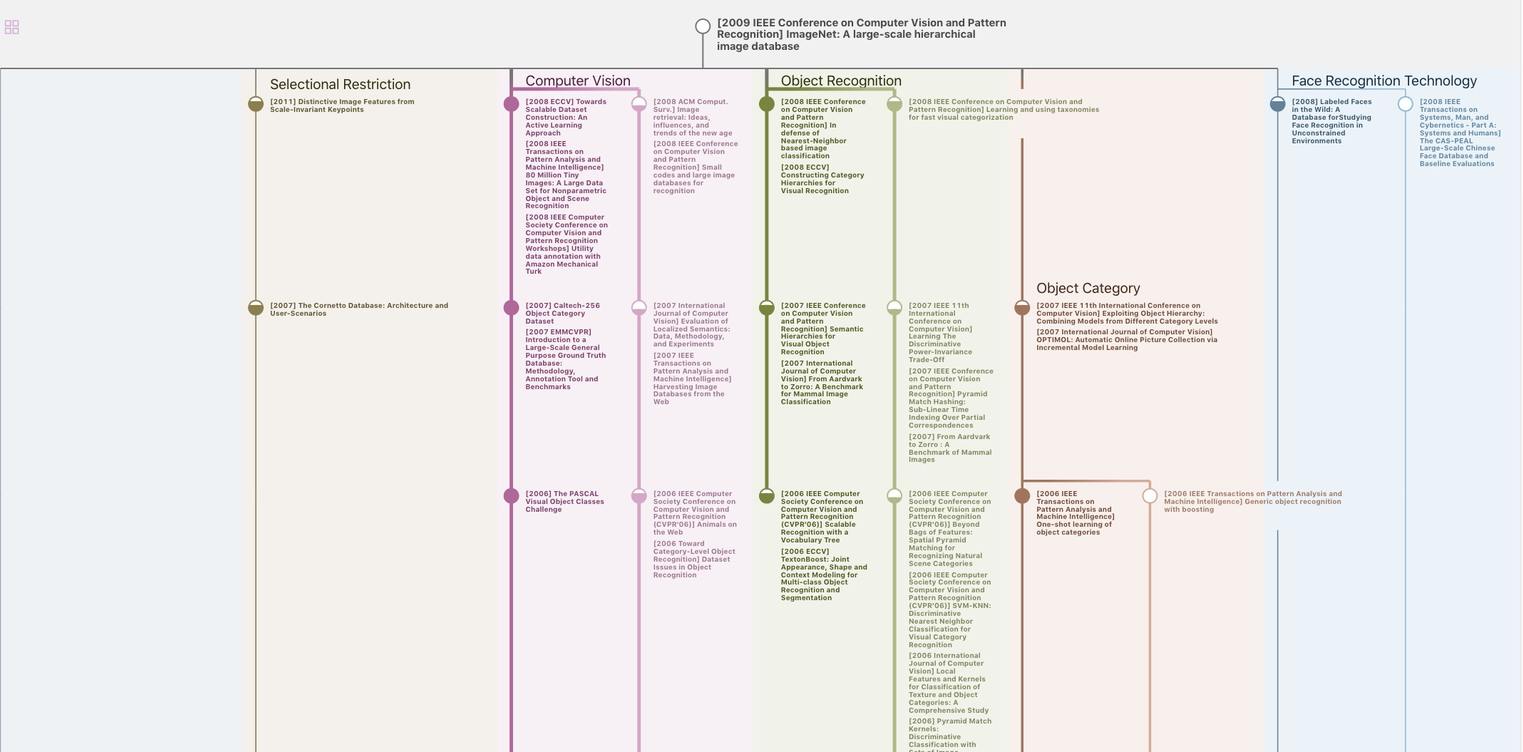
生成溯源树,研究论文发展脉络
Chat Paper
正在生成论文摘要