Tangram: Bridging Immutable And Mutable Abstractions For Distributed Data Analytics
PROCEEDINGS OF THE 2019 USENIX ANNUAL TECHNICAL CONFERENCE(2019)
摘要
Data analytics frameworks that adopt immutable data abstraction usually provide better support for failure recovery and straggler mitigation, while those that adopt mutable data abstraction are more efficient for iterative workloads thanks to their support for in-place state updates and asynchronous execution. Most existing frameworks adopt either one of the two data abstractions and do not enjoy the benefits of the other. In this paper, we propose a novel programming model named MapUpdate, which can determine whether a distributed dataset is mutable or immutable in an application. We show that MapUpdate not only offers good expressiveness, but also allows us to enjoy the benefits of both mutable and immutable abstractions. MapUpdate naturally supports iterative and asynchronous execution, and can use different recovery strategies adaptively according to failure scenarios. We implemented MapUpdate in a system, called Tangram, with novel system designs such as lightweight local task management, partition-based progress control, and context-aware failure recovery. Extensive experiments verified the benefits of Tangram on a variety of workloads including bulk processing, graph analytics, and iterative machine learning.
更多查看译文
关键词
immutable abstractions,data analytics
AI 理解论文
溯源树
样例
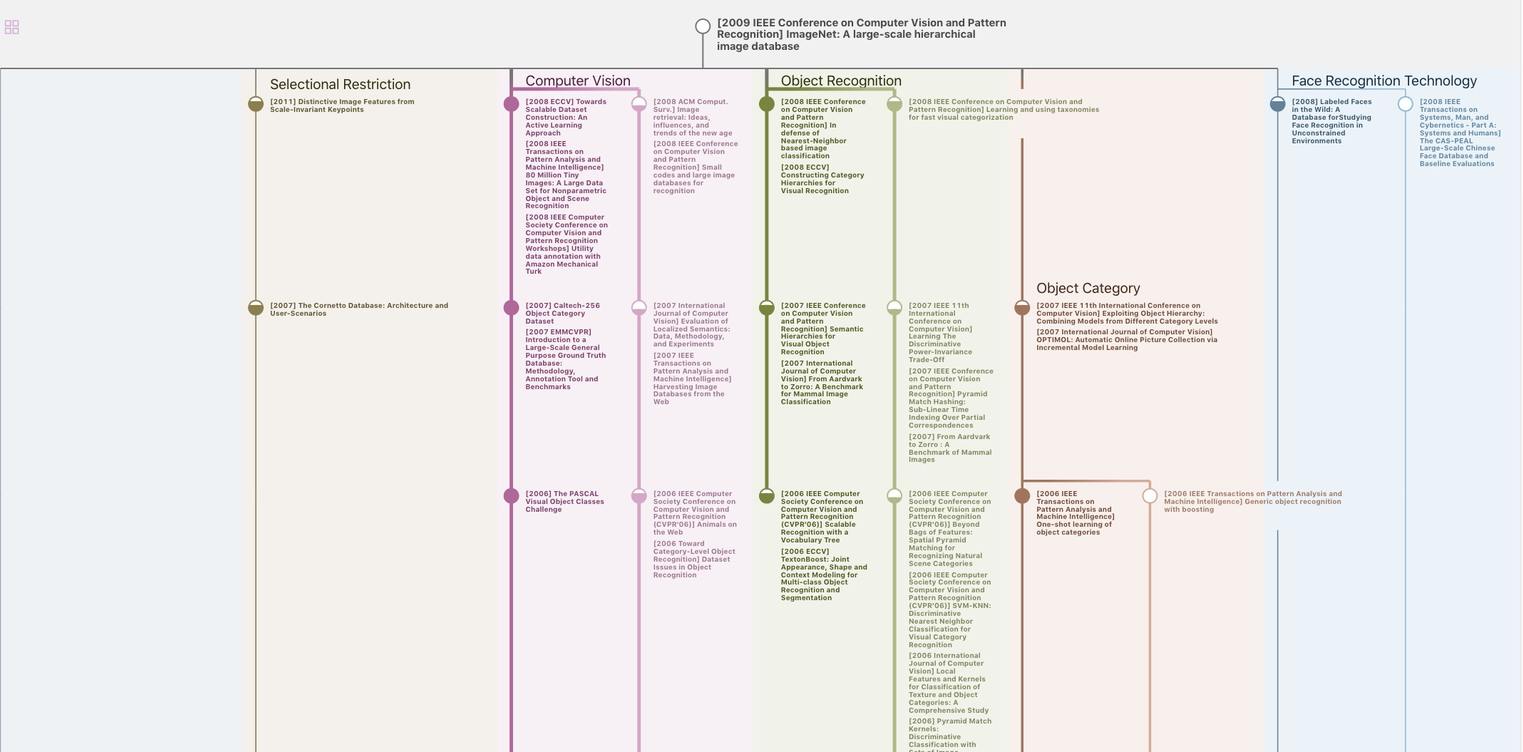
生成溯源树,研究论文发展脉络
Chat Paper
正在生成论文摘要