Data-Driven Beam Selection For Mmwave Communications With Machine And Deep Learning: An Angle Of Arrival-Based Approach
2019 IEEE INTERNATIONAL CONFERENCE ON COMMUNICATIONS WORKSHOPS (ICC WORKSHOPS)(2019)
摘要
This paper investigates the applicability of deep and machine learning techniques to perform beam selection in the uplink of a mmWave communication system. Specifically, we consider a hybrid beamforming setup comprising an analog beamforming (ABF) network followed by a zero-forcing baseband processing block. The goal is to select the optimal configuration for the ABF network bsed on the estimated angles-of-arrival (AoAs) and received powers. To that aim, we consider three machine/deep learning schemes: k-nearest neighbors (kNN), support vector classifiers (SVC), and the multilayer perceptron (MLP). We conduct an extensive performance evaluation to assess the impact of using the Capon or MUSIC methods to estimate the AoAs and powers, the size of the training dataset, the number of beamformers in the codebook, their beamwidth, or the number of active users. Computer simulations reveal that performance, in terms of classification accuracy and sum-rate, is very close to that achievable via exhaustive search.
更多查看译文
关键词
AoAs,beamformers,data-driven beam selection,mmWave communication system,hybrid beamforming setup,optimal configuration,ABF network,k-nearest neighbors,support vector classifiers,multilayer perceptron,analog beamforming network,machine learning scheme,angle of arrival-based approach,deep learning scheme,Capon method,MUSIC method,zero-forcing baseband processing block
AI 理解论文
溯源树
样例
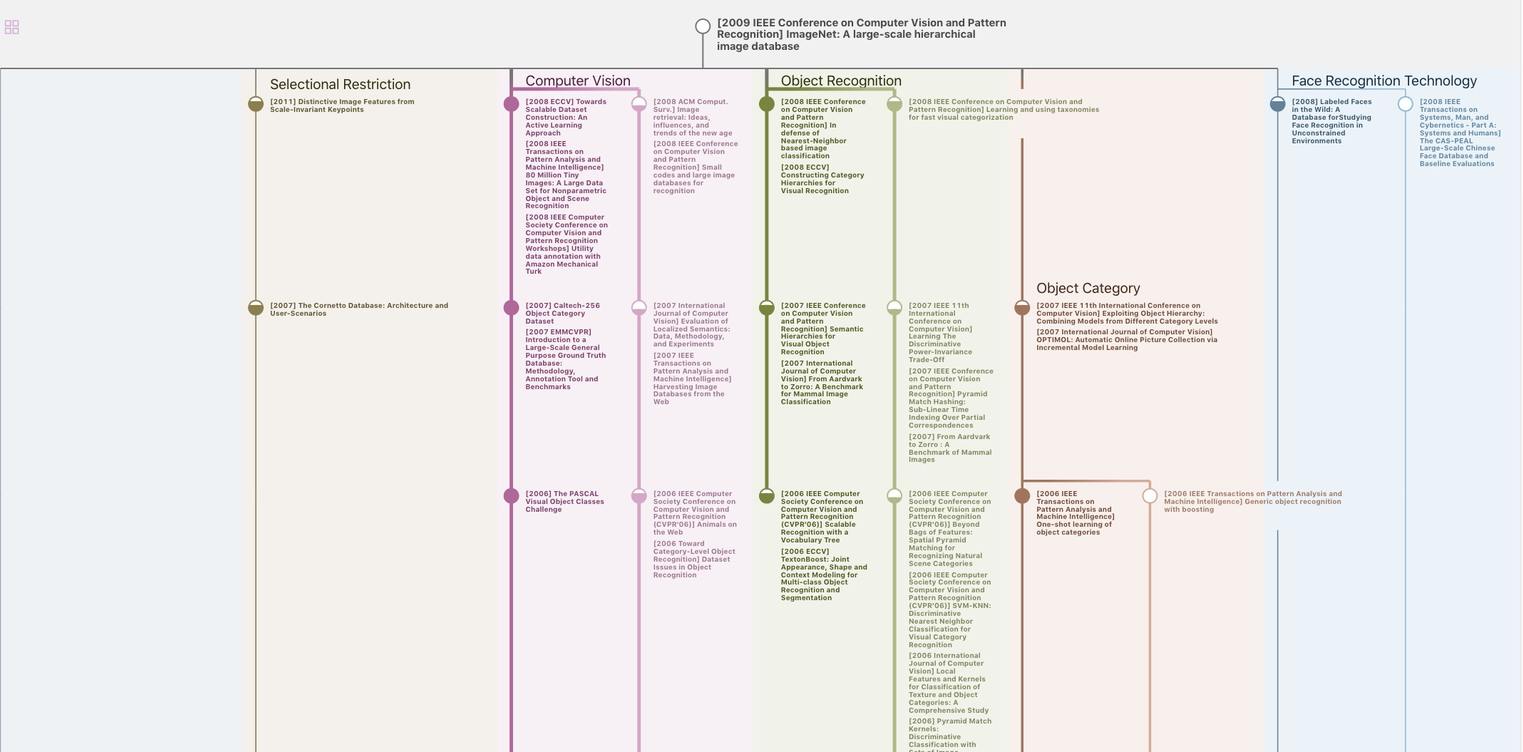
生成溯源树,研究论文发展脉络
Chat Paper
正在生成论文摘要