Pyramidal region context module for semantic segmentation
Proceedings of the ACM Turing Celebration Conference - China(2019)
摘要
Context modeling is widely exploited to enhance semantic correlation in semantic segmentation task. Recent approaches (e.g., OCNet, CCNet and DANet) apply non-local type of network to capture the context information. However, they are not accurate enough for handling scale-varying objects due to that they consider very little local dependencies of the adjacent pixels. In this work, we address the complex scene segmentation problem by combining region dependencies and global contextual information. Motivated by the fact that scale of objects largely varies on images, we design the Pyramidal Region Context Module(PRCM) to handle the neighbor relationship of multi-scale regions. In addition, we adopt a depth-to-space layer(PixelShuffle) to form the Scale Transfer Classifier (STC). Based on the two newly proposed modules, we introduce an end-to-end segmentation network - Pyramidal Region Network(PRNet). We empirically demonstrate the effectiveness of our approach on Cityscapes dataset, the results have shown impressive improvement compared with baselines. Notably, PRNet obtains mean IoU of 81.3 on test set of Cityscapes.
更多查看译文
关键词
neural networks, self-attention, semantic segmentation
AI 理解论文
溯源树
样例
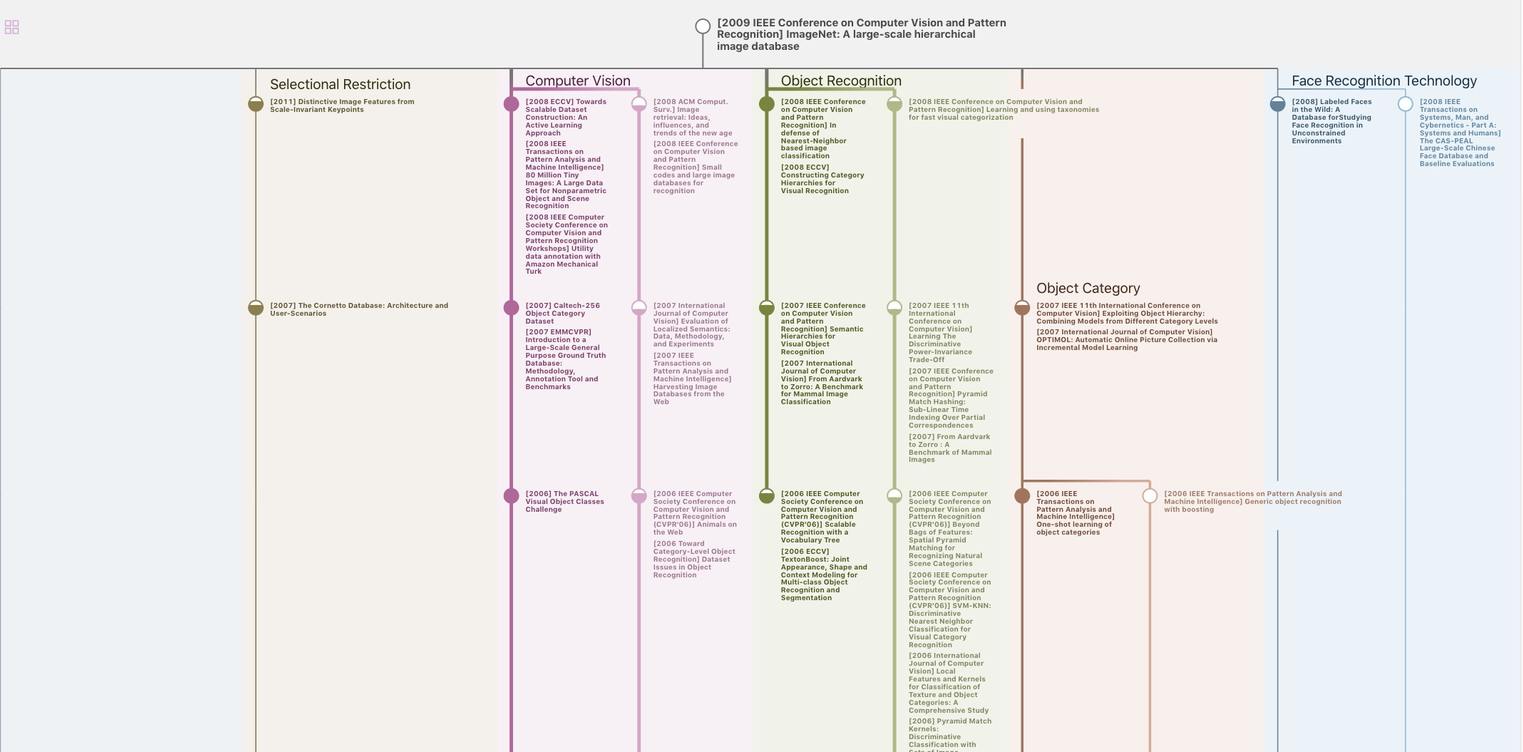
生成溯源树,研究论文发展脉络
Chat Paper
正在生成论文摘要