What And How Ot Er Datasets Can Be Leveraged For Medical Imaging Classification
ISBI(2019)
摘要
Applying deep learning for medical imaging classification suffers from limited labeled data, especially for those relying on biopsy proven labels. In this work we approach this challenge by leveraging other available datasets with focus on answering what dataset can be used, and how to use them optimally. An application was considered, classifying benign or malignant colonic polyps given colonoscopy narrow-band imaging (NB1). Experiments were conducted with 5 auxiliary datasets with various characteristics, and multiple training schemes involving transfer learning, multitask learning (MTL) with a DenseNet backbone. Results consistently suggest an optimal scheme of MTL initialized from ImageNet pretrain model and followed by fine tuning on the main task. An auxiliary dataset helps better if it's not only similar in terms of imaging mechanism or fine-grained variability, but also easy to train itself. A NB" classifier trained with such scheme and auxiliary dataset improved accuracy from 62.6% (baseline without using other dataset) to 70.7% with performance on par with 5 human experts.
更多查看译文
关键词
Machine learning, Computer-aided detection and diagnosis (CAD), Classification
AI 理解论文
溯源树
样例
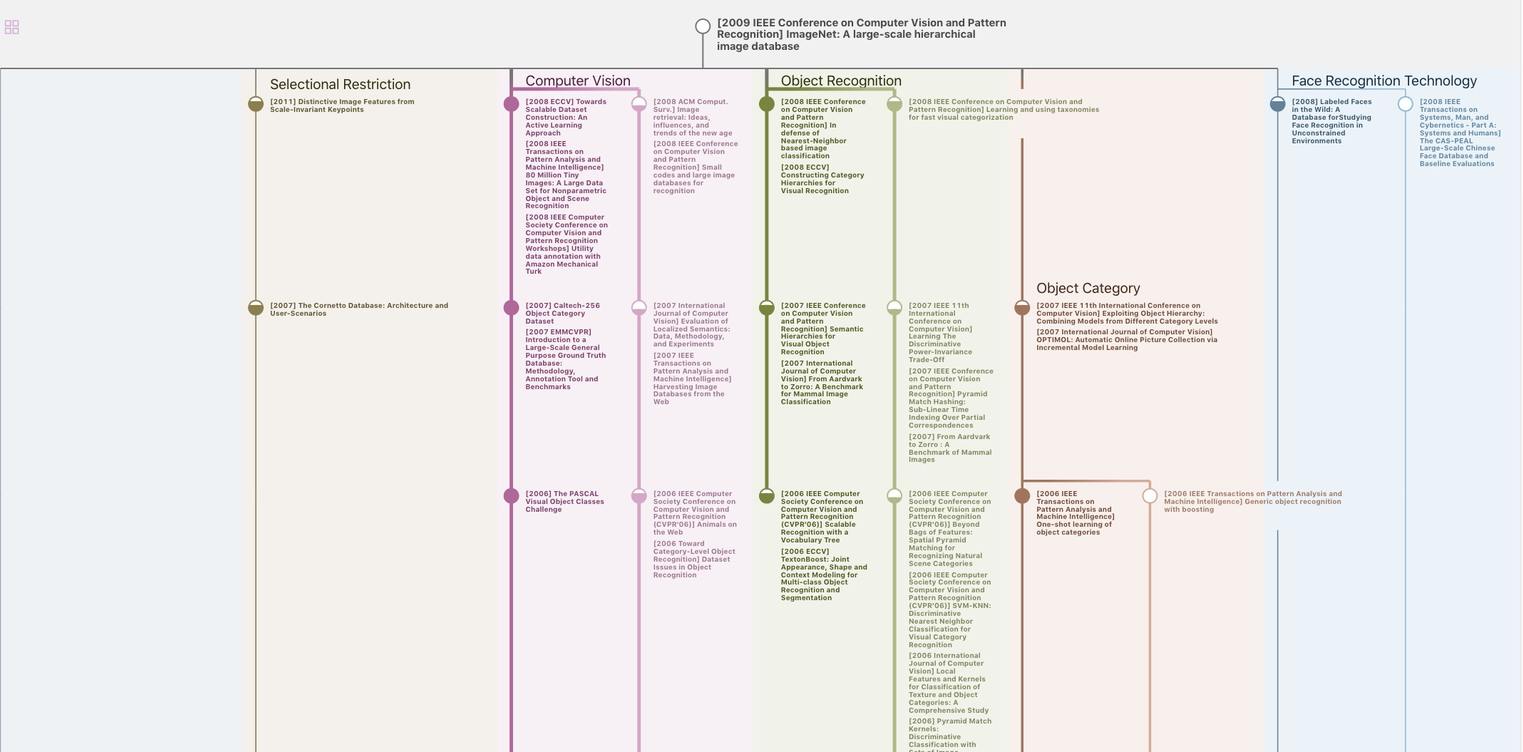
生成溯源树,研究论文发展脉络
Chat Paper
正在生成论文摘要