Classification As A Criterion To Select Model Order For Dynamic Functional Connectivity States In Rest-Fmri Data
2019 IEEE 16TH INTERNATIONAL SYMPOSIUM ON BIOMEDICAL IMAGING (ISBI 2019)(2019)
摘要
Currently, there is extensive research ongoing to analyze the dynamics of brain connectivity in resting fMRI data. In such approaches, there is often a selection of the number of connectivity states which arc present within the data. Typically, an order selection approach such as the elbow criteria is used for this when time-varying connectivity estimates are acquired using sliding-window approach and clustered using k-means algorithm. In this work we evaluate the benefits of using classification (e.g. of patients versus controls) as a criterion for evaluating the optimal number of states (or clusters). We compare different classification strategies to perform the classification while optimizing for the number of clusters (selected via a k-means approach). In our approach, we compute cross-validated classification accuracy and variability at different numbers of states for healthy control (HC) versus schizophrenia (SZ) patients. Consistent with our earlier reports, we find improvement in classification performance when dynamic connectivity measures are combined with static connectivity measures. We also show that the model order at which maximal classification accuracy is obtained (four dynamic states for this data) can be different from the order obtained using standard k-means model order selection methods (that result in five states for the data at hand) across different combinations of features trained. We also investigate if additional information from hierarchical clustering of first level states can contribute to the performance of classification accuracy and observe no evidence for additional sub-clusters in short 5-minute resting scans. In sum, the results suggest the use of classification accuracy as a promising metric for selecting the number of states in a dynamic connectivity analysis.
更多查看译文
关键词
dFNC, Classification, Hierarchical clustering, Model order
AI 理解论文
溯源树
样例
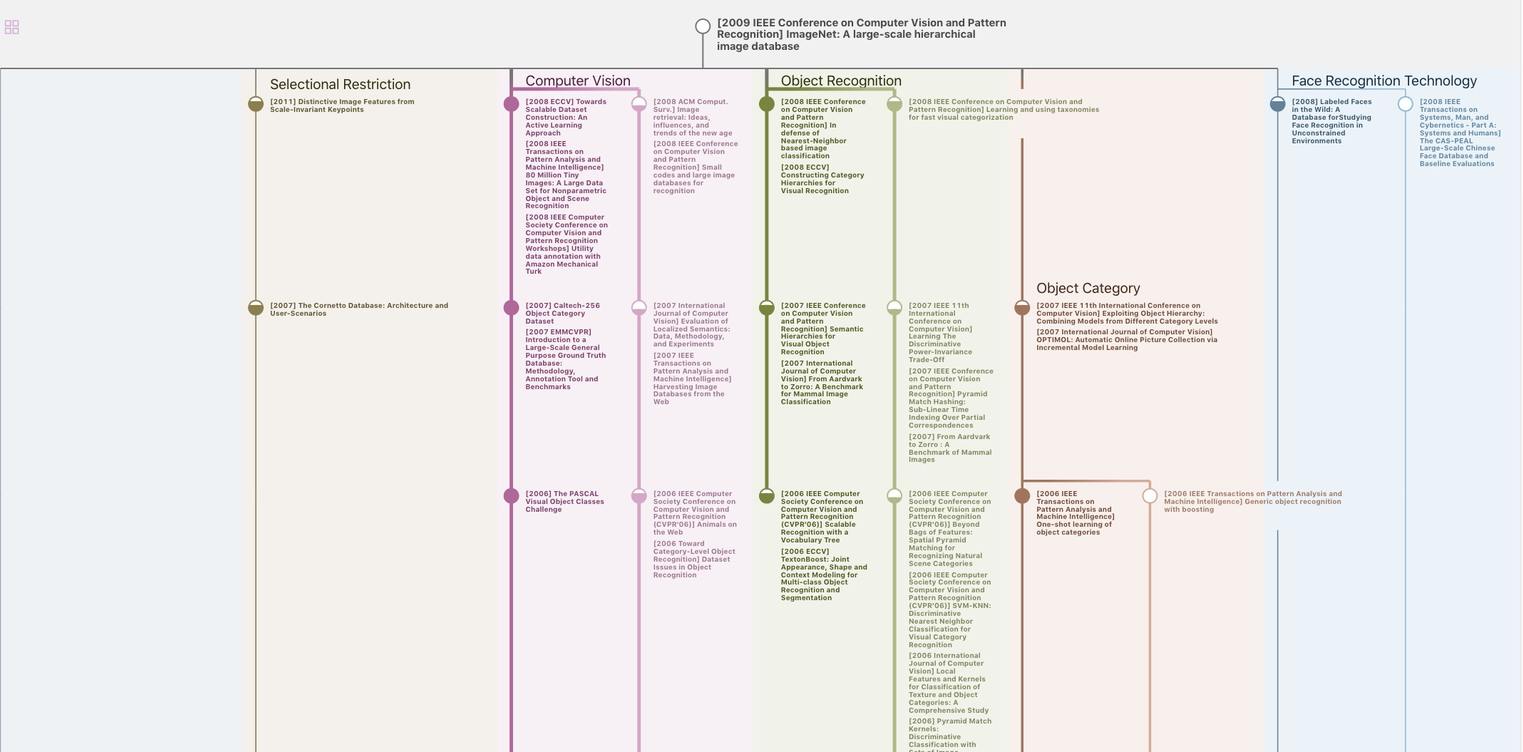
生成溯源树,研究论文发展脉络
Chat Paper
正在生成论文摘要