Segmenting The Left Ventricle In Cardiac In Cardiac Mri: From Handcrafted To Deep Region Based Descriptors
2019 IEEE 16TH INTERNATIONAL SYMPOSIUM ON BIOMEDICAL IMAGING (ISBI 2019)(2019)
摘要
Achieving robust object segmentations requires the ability to discard outliers (invalid observations) in the segmentation process. When considering an object model, such as an active shape model (ASM), described by a set of points, a one-to-one mapping from one model point to one valid observation would be ideal. However, in general, a one-to-many mapping is necessary to ensure the valid observation is detected and, thus, to have a reliable and robust fitting process. In this work, we compare three observation detectors: two of them based on handcrafted texture features and another based on a deep CNN classifier. Furthermore, to reduce from many detections to just one valid observation, we incorporate the Generalized Expectation-Maximization (GEM) algorithm in the ASM framework. This algorithm is able to neglect outliers by assigning them low weights. The proposed methodology exhibits remarkable accuracy with all the detectors in the context of the segmentation of the left ventricle in two publicly available MRI datasets, for which the proposed approach is competitive with other state-of-the-art methods.
更多查看译文
关键词
model point,active shape model,object model,segmentation process,invalid observations,robust object segmentations,deep region based descriptors,cardiac MRI,left ventricle,outliers,Generalized Expectation-Maximization algorithm,valid observation,deep CNN classifier,handcrafted texture features,observation detectors,robust fitting process,reliable process
AI 理解论文
溯源树
样例
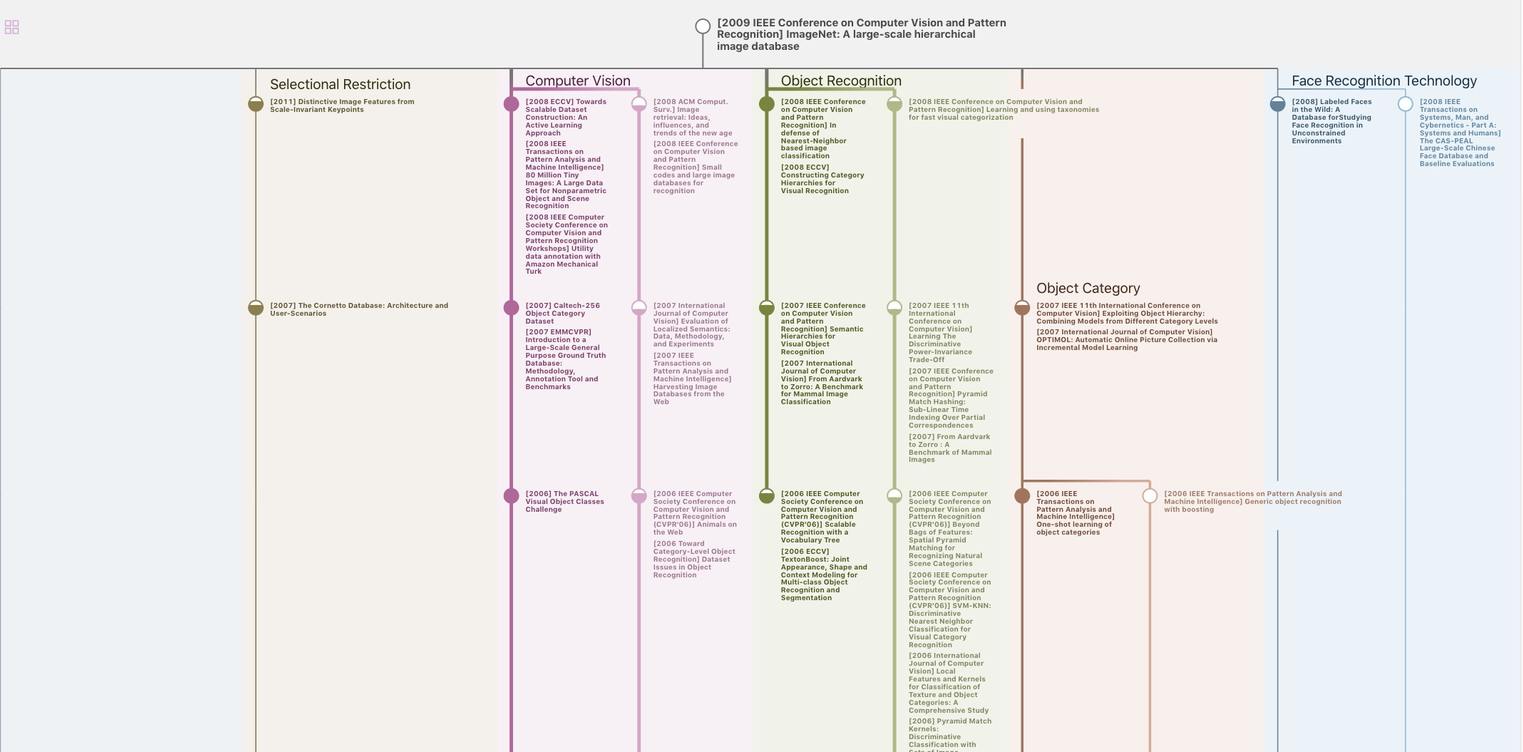
生成溯源树,研究论文发展脉络
Chat Paper
正在生成论文摘要