BAYESIAN MULTISTUDY FACTOR ANALYSIS FOR HIGH-THROUGHPUT BIOLOGICAL DATA
ANNALS OF APPLIED STATISTICS(2021)
摘要
This paper analyzes breast cancer gene expression across seven studies to identify genuine and thus replicable gene patterns shared among these studies. Our premise is that genuine biological signal is more likely to be reproducibly present in multiple studies than spurious signal. Our analysis uses a new modeling strategy for the joint analysis of high-throughput biological studies which simultaneously identifies shared as well as study-specific signal. To this end, we generalize the multi-study factor analysis model to handle high-dimensional data and generalize the sparse Bayesian infinite factor model to this context. We provide strategies for the identification of the loading matrices, common and study-specific. Through extensive simulation analysis, we characterize the performance of the proposed approach in various scenarios and show that it outperforms standard factor analysis in identifying replicable signal in all scenarios considered. The analysis of breast cancer gene expression studies identifies clear replicable gene patterns. These patterns are related to well-known biological pathways involved in breast cancer, such as the ER, cell cycle, immune system, collagen, and metabolic pathways. Some of these patterns are also associated with existing breast cancer subtypes, such as LumA, Her2, and basal subtypes, while other patterns identify novel pathways active across subtypes and missed by hierarchical clustering approaches. The R package MSFA implementing the method is available on GitHub.
更多查看译文
关键词
Dimension reduction, factor analysis, gene expression, Gibbs sampling, meta-analysis
AI 理解论文
溯源树
样例
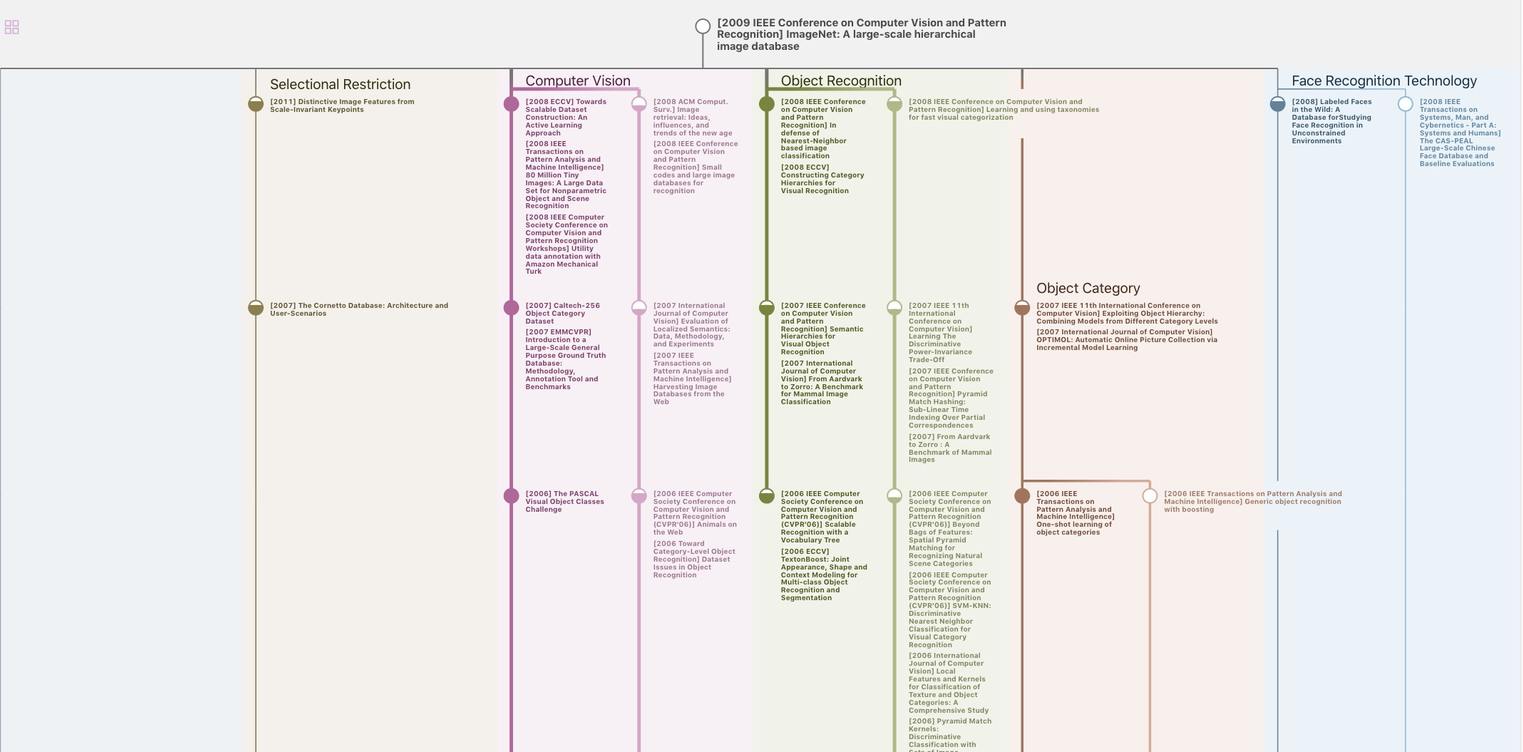
生成溯源树,研究论文发展脉络
Chat Paper
正在生成论文摘要