Path Weights In Concentration Graphs
BIOMETRIKA(2020)
摘要
A graphical model provides a compact and efficient representation of the association structure in a multivariate distribution by means of a graph. Relevant features of the distribution are represented by vertices, edges and higher-order graphical structures such as cliques or paths. Typically, paths play a central role in these models because they determine the dependence relationships between variables. However, while a theory of path coefficients is available for directed graph models, little research exists on the strength of the association represented by a path in an undirected graph. Essentially, it has been shown that the covariance between two variables can be decomposed into a sum of weights associated with each of the paths connecting the two variables in the corresponding concentration graph. In this context, we consider concentration graph models and provide an extensive analysis of the properties of path weights and their interpretation. Specifically, we give an interpretation of covariance weights through their factorization into a partial covariance and an inflation factor. We then extend the covariance decomposition over the paths of an undirected graph to other measures of association, such as the marginal correlation coefficient and a quantity that we call the inflated correlation. Application of these results is illustrated with an analysis of dietary intake networks.
更多查看译文
关键词
Concentration graph, Covariance decomposition, Inflation factor, Partial correlation, Undirected graphical model, Undirected path
AI 理解论文
溯源树
样例
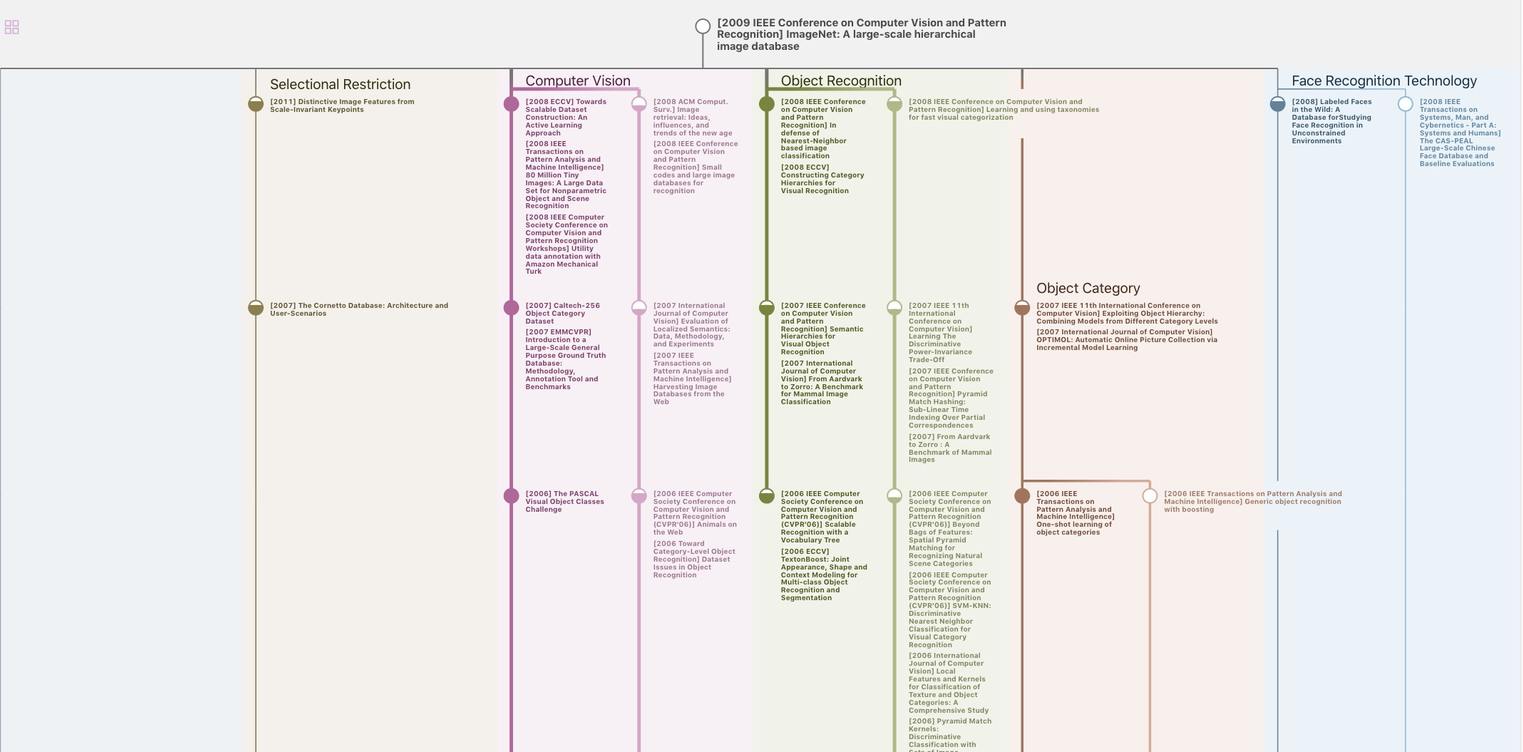
生成溯源树,研究论文发展脉络
Chat Paper
正在生成论文摘要