Identifying signal-dependent information about the preictal state: A comparison across ECoG, EEG and EKG using deep learning.
EBioMedicine(2019)
摘要
BACKGROUND:The inability to reliably assess seizure risk is a major burden for epilepsy patients and prevents developing better treatments. Recent advances have paved the way for increasingly accurate seizure preictal state detection algorithms, primarily using electrocorticography (ECoG). To develop seizure forecasting for broad clinical and ambulatory use, however, less complex and invasive modalities are needed. Algorithms using scalp electroencephalography (EEG) and electrocardiography (EKG) have also achieved better than chance performance. But it remains unknown how much preictal information is in ECoG versus modalities amenable to everyday use - such as EKG and single channel EEG - and how to optimally extract that preictal information for seizure prediction.
METHODS:We apply deep learning - a powerful method to extract information from complex data - on a large epilepsy data set containing multi-day, simultaneous recordings of EKG, ECoG, and EEG, using a variety of feature sets. We use the relative performance of our algorithms to compare the preictal information contained in each modality.
RESULTS:We find that single-channel EKG contains a comparable amount of preictal information as scalp EEG with up to 21 channels and that preictal information is best extracted not with standard heart rate measures, but from the power spectral density. We report that preictal information is not preferentially contained in EEG or ECoG channels within the seizure onset zone.
CONCLUSION:Collectively, these insights may help to devise future prospective, minimally invasive long-term epilepsy monitoring trials with single-channel EKG as a particularly promising modality.
更多查看译文
AI 理解论文
溯源树
样例
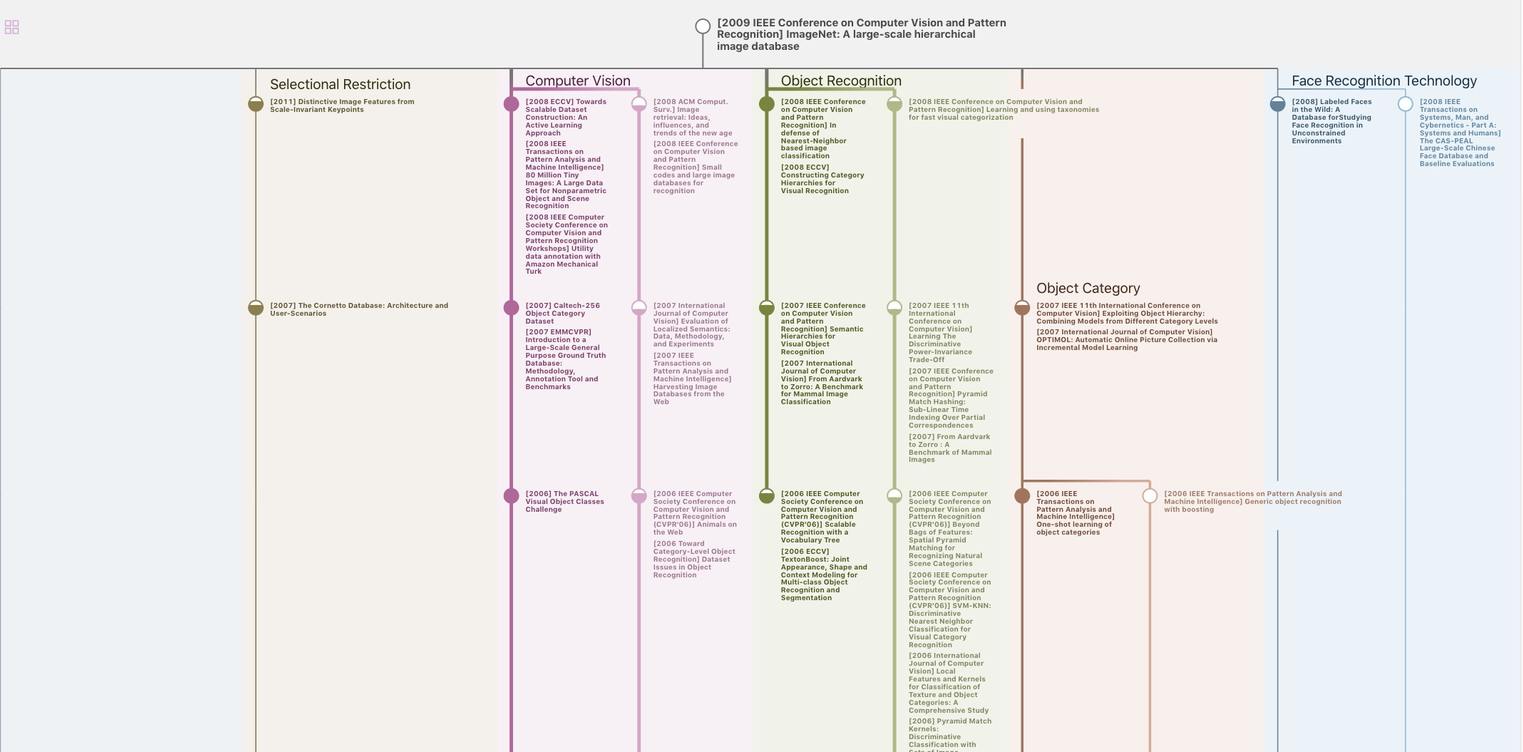
生成溯源树,研究论文发展脉络
Chat Paper
正在生成论文摘要