Skip-Connected Covariance Network for Remote Sensing Scene Classification.
IEEE Transactions on Neural Networks and Learning Systems(2020)
摘要
This paper proposes a novel end-to-end learning model, called skip-connected covariance (SCCov) network, for remote sensing scene classification (RSSC). The innovative contribution of this paper is to embed two novel modules into the traditional convolutional neural network (CNN) model, i.e., skip connections and covariance pooling. The advantages of newly developed SCCov are twofold. First, by means of the skip connections, the multi-resolution feature maps produced by the CNN are combined together, which provides important benefits to address the presence of large-scale variance in RSSC data sets. Second, by using covariance pooling, we can fully exploit the second-order information contained in such multi-resolution feature maps. This allows the CNN to achieve more representative feature learning when dealing with RSSC problems. Experimental results, conducted using three large-scale benchmark data sets, demonstrate that our newly proposed SCCov network exhibits very competitive or superior classification performance when compared with the current state-of-the-art RSSC techniques, using a much lower amount of parameters. Specifically, our SCCov only needs 10% of the parameters used by its counterparts.
更多查看译文
关键词
Feature extraction,Learning systems,Neural networks,Training,Aggregates,Computational modeling,Remote sensing
AI 理解论文
溯源树
样例
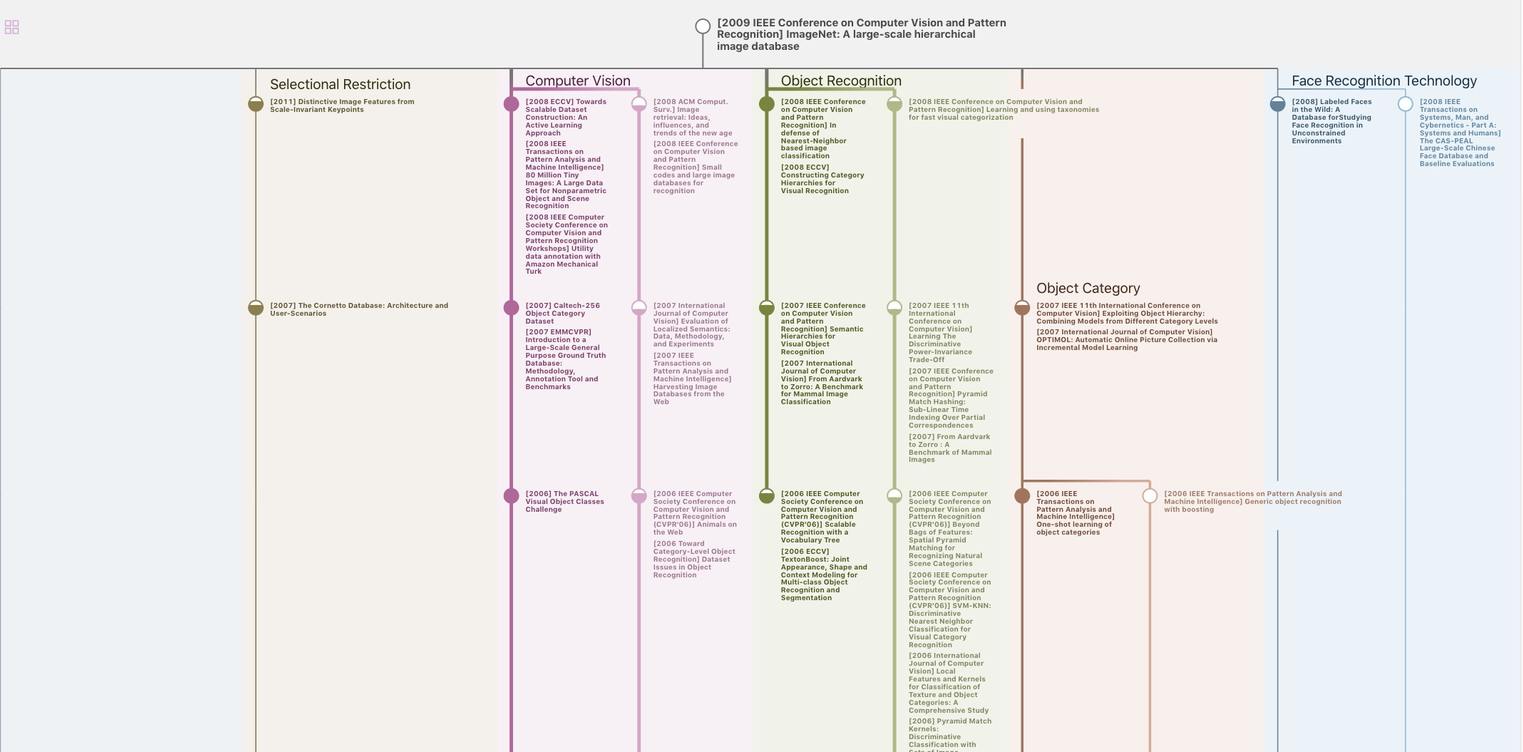
生成溯源树,研究论文发展脉络
Chat Paper
正在生成论文摘要