Application of classification for figure copying test in Parkinson's disease diagnosis by using cartesian genetic programming.
GECCO(2019)
摘要
Previous studies have proposed an objective non-invasive approach to assist diagnosing neurological diseases such as Alzheimer and Parkinson's diseases by asking patients to perform certain drawing tasks against certain figure. However, the approach of rating those drawing test results is still very subjective by relying on manual measurements. By extracting features of the drawn figure from the raw data, which is generated from the digitized tablet that patients can draw on, we can use supervised learning to train the evolutionary algorithm with those extracted data, and therefore evolves an automated classifier to analyse and classify those drawing accurately. Cartesian Genetic Programming (CGP) is an improved version of conventional Genetic Programming (GP). As GP adapts the tree structure, redundancy issue exists as the tree develops more nodes with the evolution of the GP by mutation and crossover. CGP addresses this issue by using fixed number of nodes and arities, evolves by using mutation only. The outcome of this research is a highly efficient, accurate, automated classifier that can not only classify clinical drawing test results, which can provide up to 80% accuracy, but also assisting clinicians and medical experts to investigate how those features are used by the algorithm and how each component can impact patient's cognitive function.
更多查看译文
关键词
Cartesian Genetic Programming, Clinical Drawing Test, Genetic Programming, Machine Learning
AI 理解论文
溯源树
样例
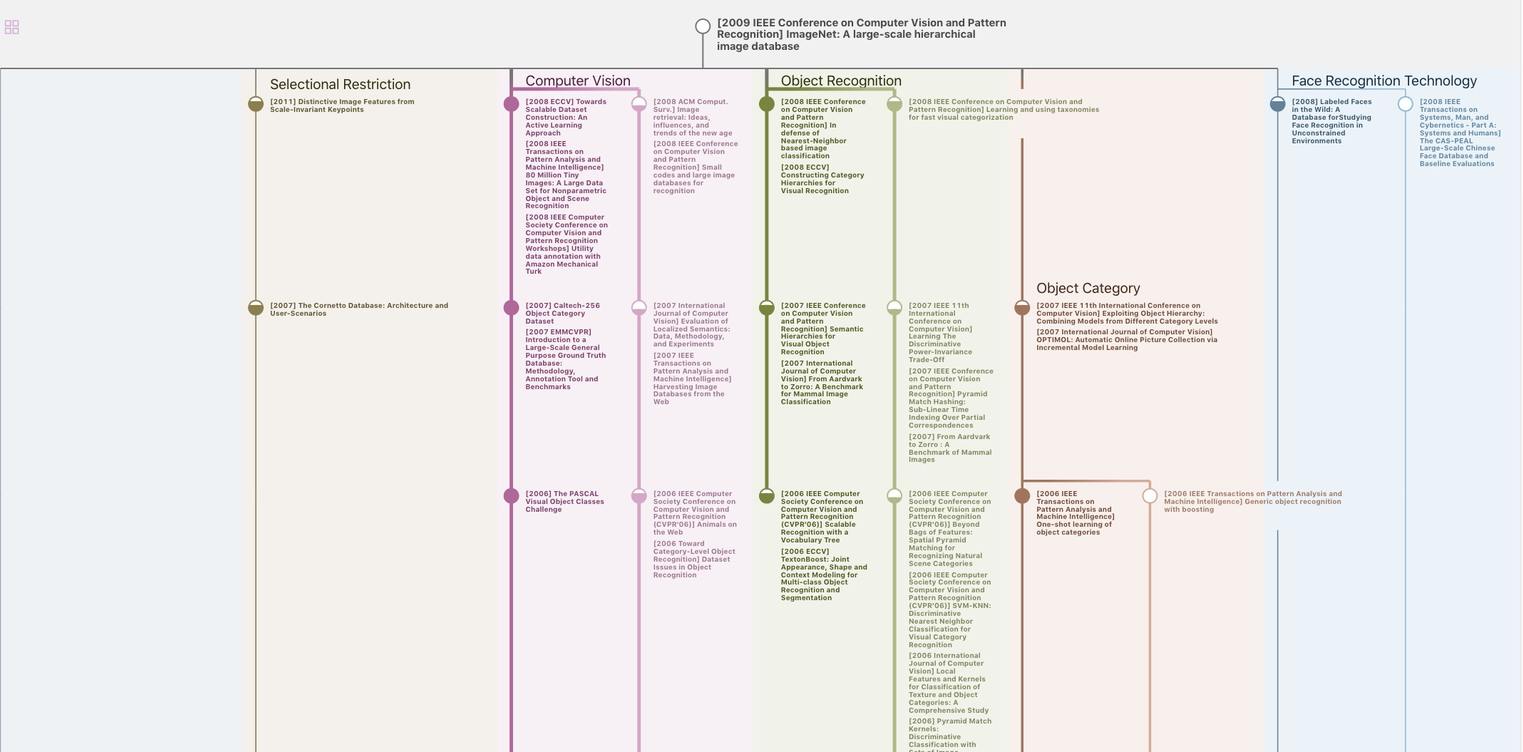
生成溯源树,研究论文发展脉络
Chat Paper
正在生成论文摘要