Provably Efficient Reinforcement Learning with Linear Function Approximation
COLT(2020)
摘要
Modern Reinforcement Learning (RL) is commonly applied to practical problems with an enormous number of states, where function approximation must be deployed to approximate either the value function or the policy. The introduction of function approximation raises a fundamental set of challenges involving computational and statistical efficiency, especially given the need to manage the exploration/exploitation tradeoff. As a result, a core RL question remains open: how can we design provably efficient RL algorithms that incorporate function approximation? This question persists even in a basic setting with linear dynamics and linear rewards, for which only linear function approximation is needed. This paper presents the first provable RL algorithm with both polynomial runtime and polynomial sample complexity in this linear setting, without requiring a "simulator" or additional assumptions. Concretely, we prove that an optimistic modification of Least-Squares Value Iteration (LSVI)---a classical algorithm frequently studied in the linear setting---achieves $\tilde{\mathcal{O}}(\sqrt{d^3H^3T})$ regret, where $d$ is the ambient dimension of feature space, $H$ is the length of each episode, and $T$ is the total number of steps. Importantly, such regret is independent of the number of states and actions.
更多查看译文
关键词
Primary: 90C40,secondary: 68T05,reinforcement learning,episodic MDP,linear function approximation,exploration
AI 理解论文
溯源树
样例
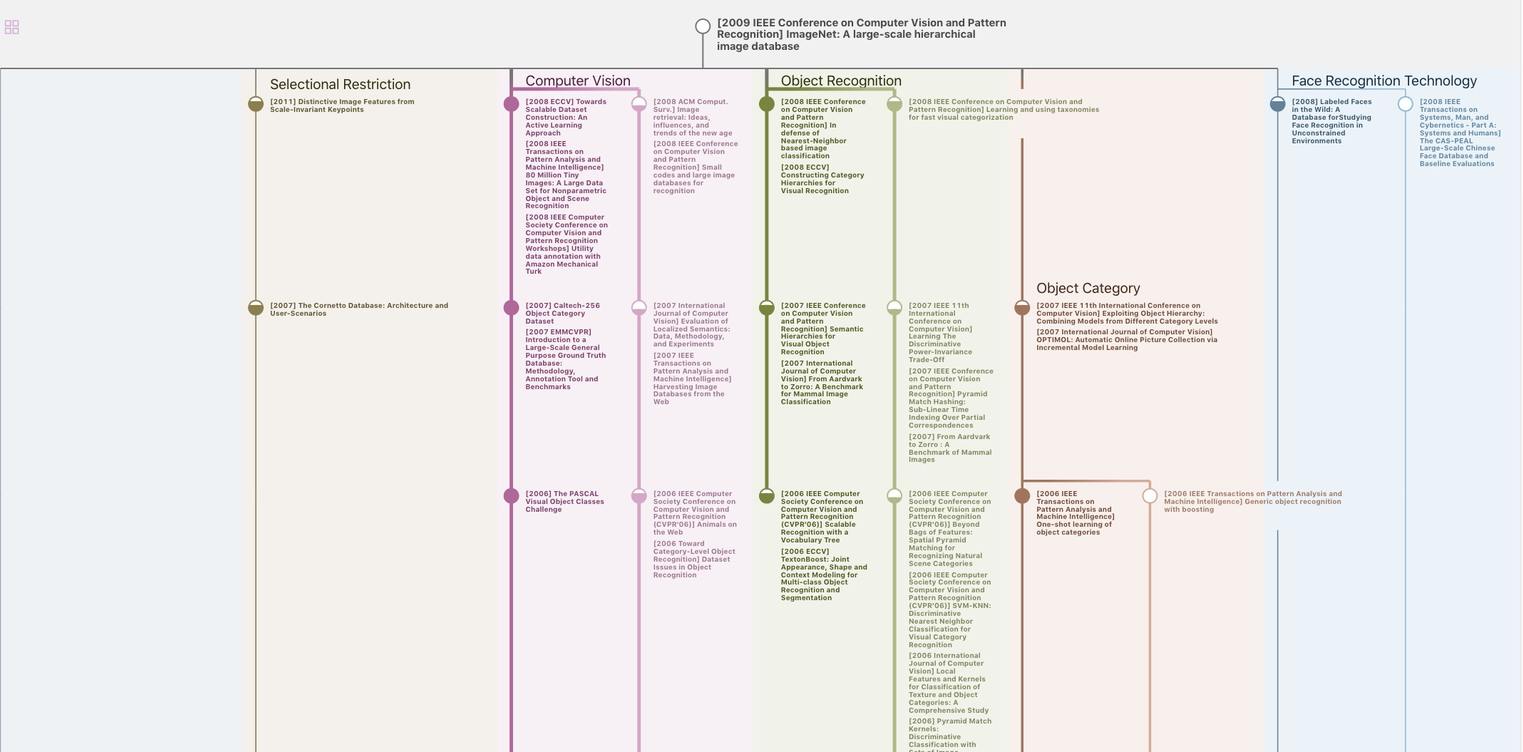
生成溯源树,研究论文发展脉络
Chat Paper
正在生成论文摘要