Quantum and classical algorithms for approximate submodular function minimization.
QUANTUM INFORMATION & COMPUTATION(2019)
摘要
Submodular functions are set functions mapping every subset of some ground set of size n into the real numbers and satisfying the diminishing returns property. Submodular minimization is an important field in discrete optimization theory due to its relevance for various branches of mathematics, computer science and economics. The currently fastest strongly polynomial algorithm for exact minimization [1] runs in time (O) over tilde (n(3) center dot EO + n(4)) where EO denotes the cost to evaluate the function on any set. For functions with range [1], the best epsilon-additive approximation algorithm [2] runs in time (O) over tilde (n(5/3)/epsilon(2) center dot EO). In this paper we present a classical and a quantum algorithm for approximate submodular minimization. Our classical result improves on the algorithm of [2] and runs in time (O) over tilde (n(3/2)/epsilon(2) center dot EO). Our quantum algorithm is, up to our knowledge, the first attempt to use quantum computing for submodular optimization. The algorithm runs in time (O) over tilde (n(5/4)/epsilon(5/2) center dot log(1/epsilon) center dot EO). The main ingredient of the quantum result is a new method for sampling with high probability T independent elements from any discrete probability distribution of support size n in time O(root Tn). Previous quantum algorithms for this problem were of complexity O(T root n).
更多查看译文
关键词
Submodular functions,approximate minimization,quantum algorithms,sub-gradient descent
AI 理解论文
溯源树
样例
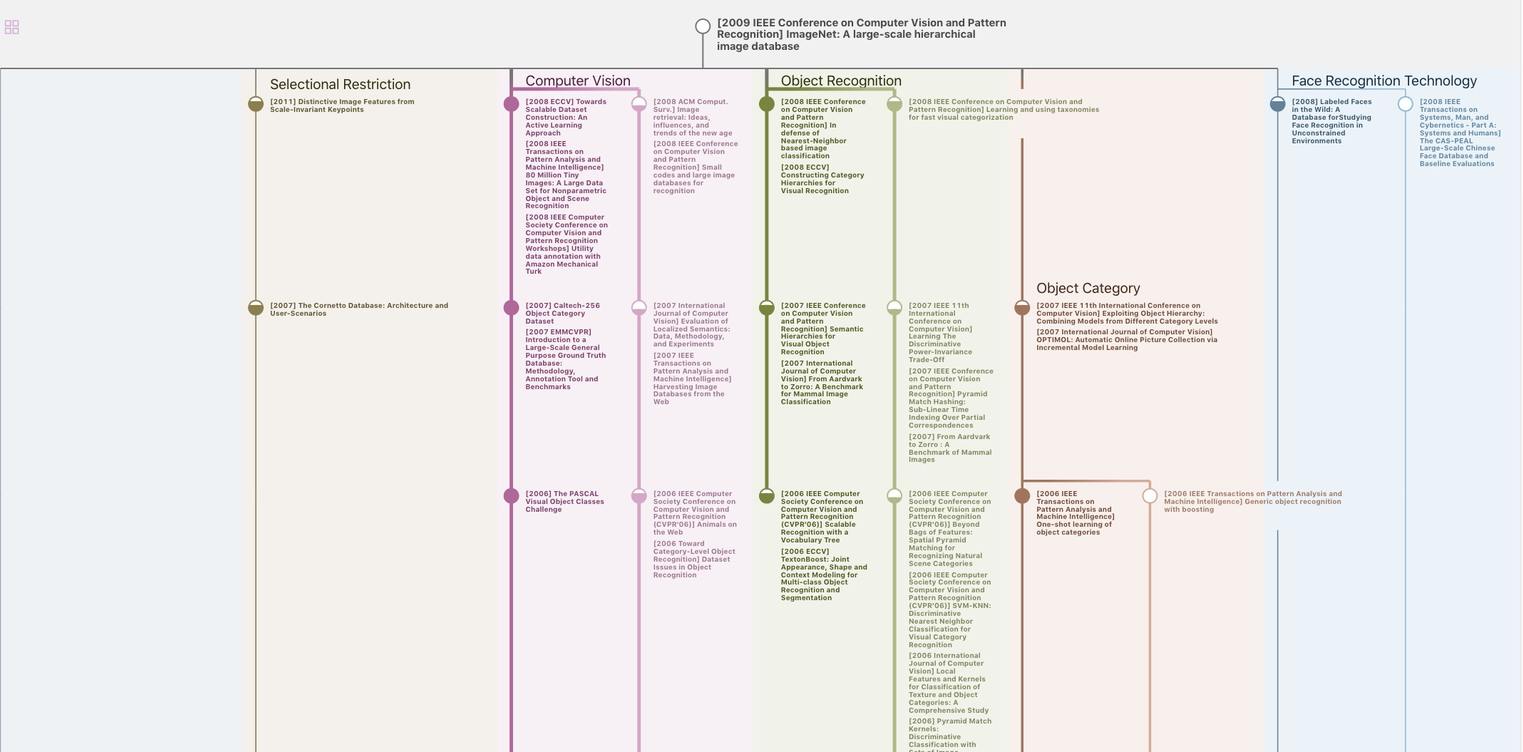
生成溯源树,研究论文发展脉络
Chat Paper
正在生成论文摘要