Automated False Alarm Reduction in a Real-Life Intensive Care Setting Using Motion Detection
Neurocritical Care(2019)
摘要
Background Contemporary monitoring systems are sensitive to motion artifacts and cause an excess of false alarms. This results in alarm fatigue and hazardous alarm desensitization. To reduce the number of false alarms, we developed and validated a novel algorithm to classify alarms, based on automatic motion detection in videos. Methods We considered alarms generated by the following continuously measured parameters: arterial oxygen saturation, systolic blood pressure, mean blood pressure, heart rate, and mean intracranial pressure. The movements of the patient and in his/her surroundings were monitored by a camera situated at the ceiling. Using the algorithm, alarms were classified into RED (true), ORANGE (possibly false), and GREEN alarms (false, i.e., artifact). Alarms were reclassified by blinded clinicians. The performance was evaluated using confusion matrices. Results A total of 2349 alarms from 45 patients were reclassified. For RED alarms, sensitivity was high (87.0%) and specificity was low (29.6%) for all parameters. As the sensitivities and specificities for RED and GREEN alarms are interrelated, the opposite was observed for GREEN alarms, i.e., low sensitivity (30.2%) and high specificity (87.2%). As RED alarms should not be missed, even at the expense of false positives, the performance was acceptable. The low sensitivity for GREEN alarms is acceptable, as it is not harmful to tag a GREEN alarm as RED/ORANGE. It still contributes to alarm reduction. However, a 12.8% false-positive rate for GREEN alarms is critical. Conclusions The proposed system is a step forward toward alarm reduction; however, implementation of additional layers, such as signal curve analysis, multiple parameter correlation analysis and/or more sophisticated video-based analytics are needed for improvement.
更多查看译文
关键词
Smart alarms, Motion sensor, Alarm reduction, ICU, Alarm fatigue, False alarms
AI 理解论文
溯源树
样例
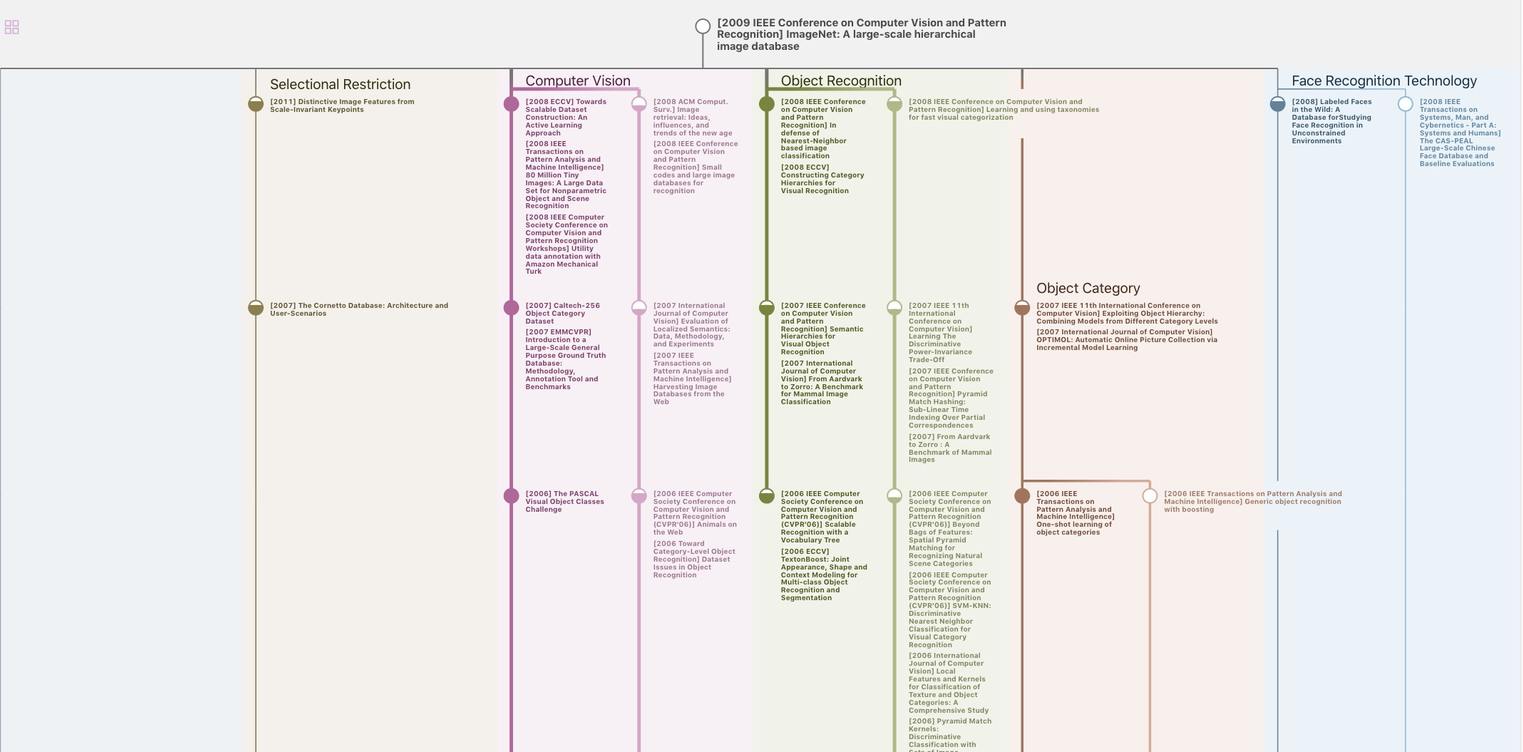
生成溯源树,研究论文发展脉络
Chat Paper
正在生成论文摘要