An Attention Mechanism for Musical Instrument Recognition.
CoRR(2019)
摘要
While the automatic recognition of musical instruments has seen significant progress, the task is still considered hard for music featuring multiple instruments as opposed to single instrument recordings. Datasets for polyphonic instrument recognition can be categorized into roughly two categories. Some, such as MedleyDB, have strong per-frame instrument activity annotations but are usually small in size. Other, larger datasets such as OpenMIC only have weak labels, i.e., instrument presence or absence is annotated only for long snippets of a song. We explore an attention mechanism for handling weakly labeled data for multi-label instrument recognition. Attention has been found to perform well for other tasks with weakly labeled data. We compare the proposed attention model to multiple models which include a baseline binary relevance random forest, recurrent neural network, and fully connected neural networks. Our results show that incorporating attention leads to an overall improvement in classification accuracy metrics across all 20 instruments in the OpenMIC dataset. We find that attention enables models to focus on (or `attend to') specific time segments in the audio relevant to each instrument label leading to interpretable results.
更多查看译文
关键词
musical instrument recognition,attention mechanism
AI 理解论文
溯源树
样例
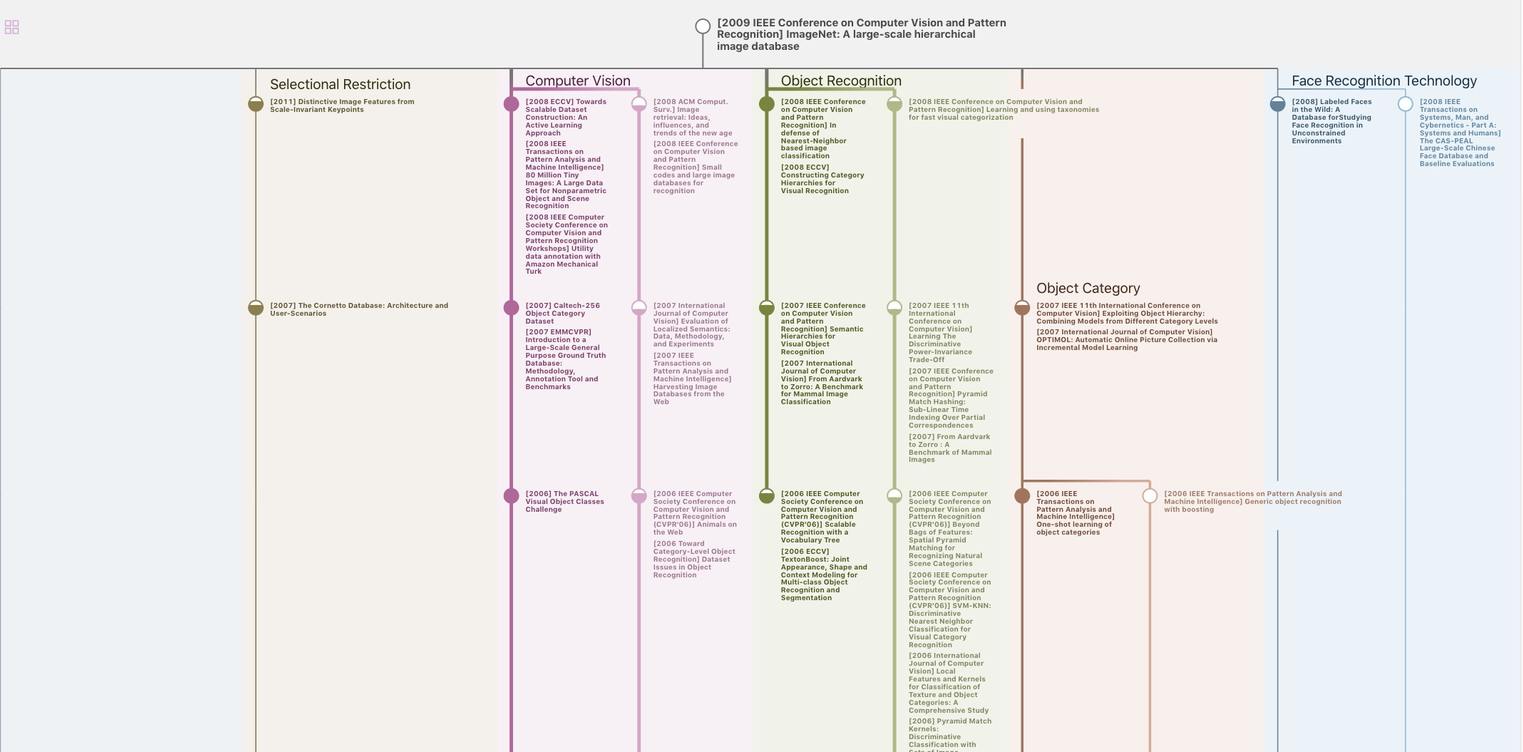
生成溯源树,研究论文发展脉络
Chat Paper
正在生成论文摘要