SVRG-MKL: A Fast and Scalable Multiple Kernel Learning Solution for Features Combination in Multi-Class Classification Problems.
IEEE Transactions on Neural Networks and Learning Systems(2020)
摘要
In this paper, we present a novel strategy to combine a set of compact descriptors to leverage an associated recognition task. We formulate the problem from a multiple kernel learning (MKL) perspective and solve it following a stochastic variance reduced gradient (SVRG) approach to address its scalability, currently an open issue. MKL models are ideal candidates to jointly learn the optimal combination of features along with its associated predictor. However, they are unable to scale beyond a dozen thousand of samples due to high computational and memory requirements, which severely limits their applicability. We propose SVRG-MKL, an MKL solution with inherent scalability properties that can optimally combine multiple descriptors involving millions of samples. Our solution takes place directly in the primal to avoid Gram matrices computation and memory allocation, whereas the optimization is performed with a proposed algorithm of linear complexity and hence computationally efficient. Our proposition builds upon recent progress in SVRG with the distinction that each kernel is treated differently during optimization, which results in a faster convergence than applying off-the-shelf SVRG into MKL. Extensive experimental validation conducted on several benchmarking data sets confirms a higher accuracy and a significant speedup of our solution. Our technique can be extended to other MKL problems, including visual search and transfer learning, as well as other formulations, such as group-sensitive (GMKL) and localized MKL (LMKL) in convex settings.
更多查看译文
关键词
Kernel,Scalability,Task analysis,Complexity theory,Memory management,Optimization,Convergence
AI 理解论文
溯源树
样例
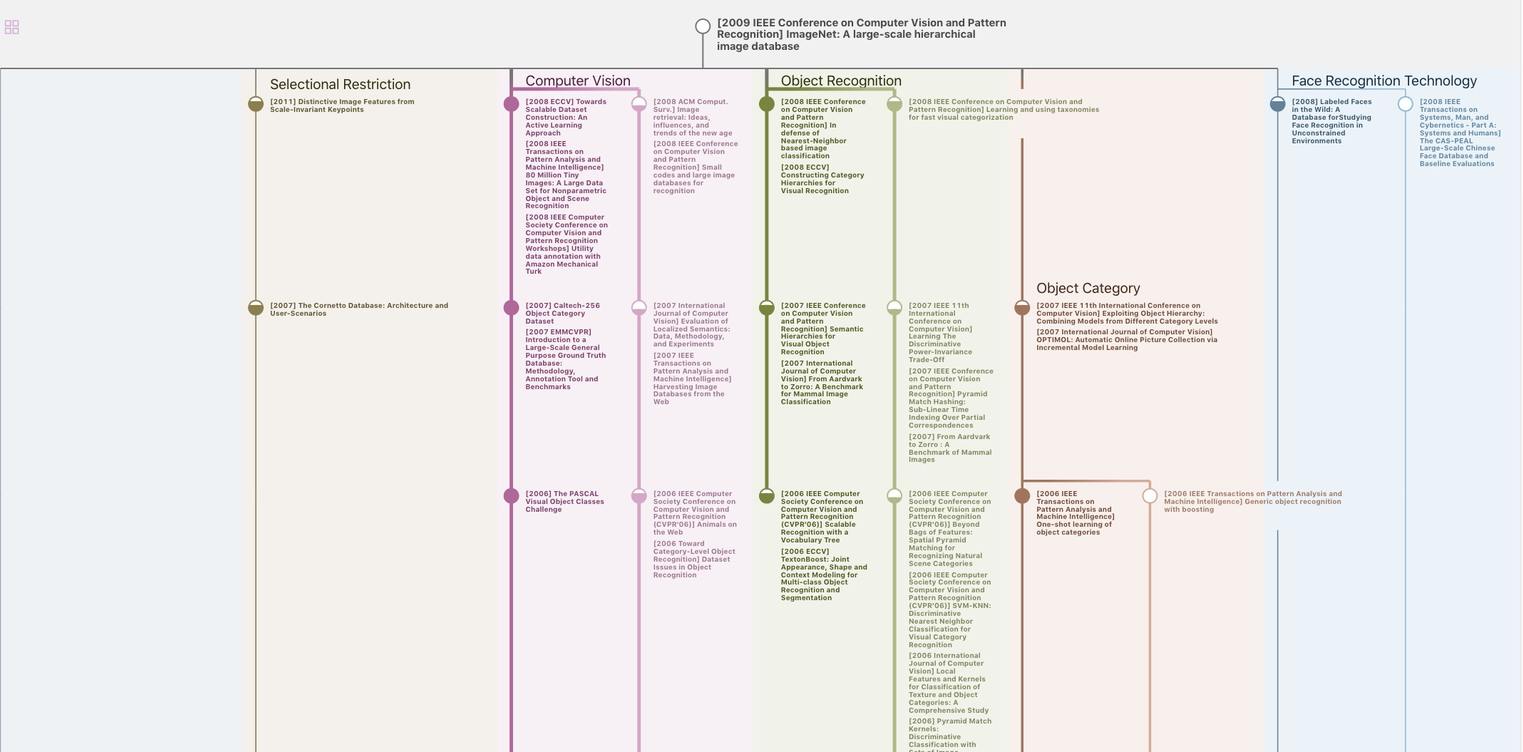
生成溯源树,研究论文发展脉络
Chat Paper
正在生成论文摘要