Using Inferred Gestures from sEMG Signal to Teleoperate a Domestic Robot for the Disabled.
ADVANCES IN COMPUTATIONAL INTELLIGENCE, IWANN 2019, PT II(2019)
摘要
With the lightning speed of technological evolution, several methods have been proposed with the aim of controlling robots and using them to serve humanity. In this work, we present and evaluate a novel learning-based system to control Pepper, the humanoid robot. We lever-aged an existing low-cost surface electromyography (sEMG) sensor, that is in the consumer market, Myo armband. To achieve our goal, we created a dataset including 6 hand gestures recorded from 35 intact people by the usage of the Myo Armband device, which has 8 non-intrusive sEMG sensors. Using raw signals extracted from Myo armband, we have been able to train a gated recurrent unit-based network to perform gesture classification. Afterwards, we integrated our system with a live hand gesture recognition application, transmitting the commands to the robot for implementing a live teleoperation method. In this way, we are able to evaluate in real-time the capabilities of our system. According to the experiments, the teleoperation of a Pepper robot achieved an average of 77.5% accuracy during test.
更多查看译文
关键词
Surface electromyography sensor,Dataset,Gated recurrent units,Gesture recognition,Pepper
AI 理解论文
溯源树
样例
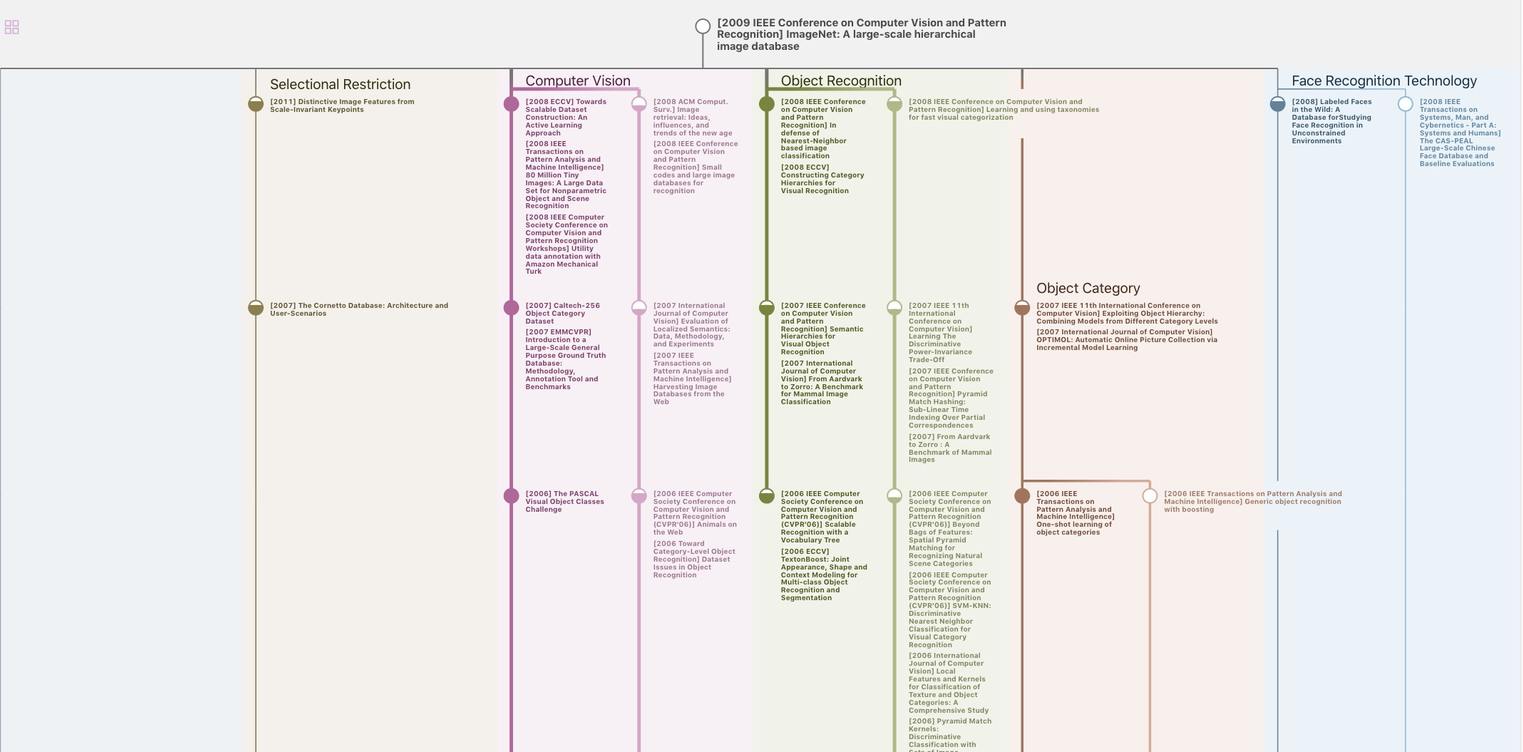
生成溯源树,研究论文发展脉络
Chat Paper
正在生成论文摘要