Sulcal And Cortical Features For Classification Of Alzheimer'S Disease And Mild Cognitive
IMAGE ANALYSIS(2019)
摘要
Prominent changes in sulcal morphology and cortical thickness characterize the neurodegeneration in Alzheimer's disease (AD). A combination of these measures has a potential of predicting AD and distinguishing it from mild cognitive impairment (MCI) and cognitively normal control subjects (CN). The purpose of this study was to propose a machine learning and pattern recognition approach of combining sulcal morphology features and cortical thickness measures as biomarkers for AD. Sulcal features (depth, length, mean and Gaussian curvature, surface area) and cortical thickness measures were extracted from 241 T1 MRI scans from ADNI database (81 AD, 75 MCI, 85 CN). SVM classifiers provided the highest accuracy of 95.0%, 93.0% sensitivity, and 97.0% specificity (AUC of 0.95) when classifying CN and AD. The majority of the features were located in the left hemisphere, which in AD is reported to be more severely affected by atrophy, and to lose gray matter faster than the right. Results indicate that a combination of sulcal and cortical features provides high classification results, which are competitive with the state-of-the-art techniques.
更多查看译文
关键词
Pattern recognition, Alzheimer's disease, SVM, Morphology, Feature extraction
AI 理解论文
溯源树
样例
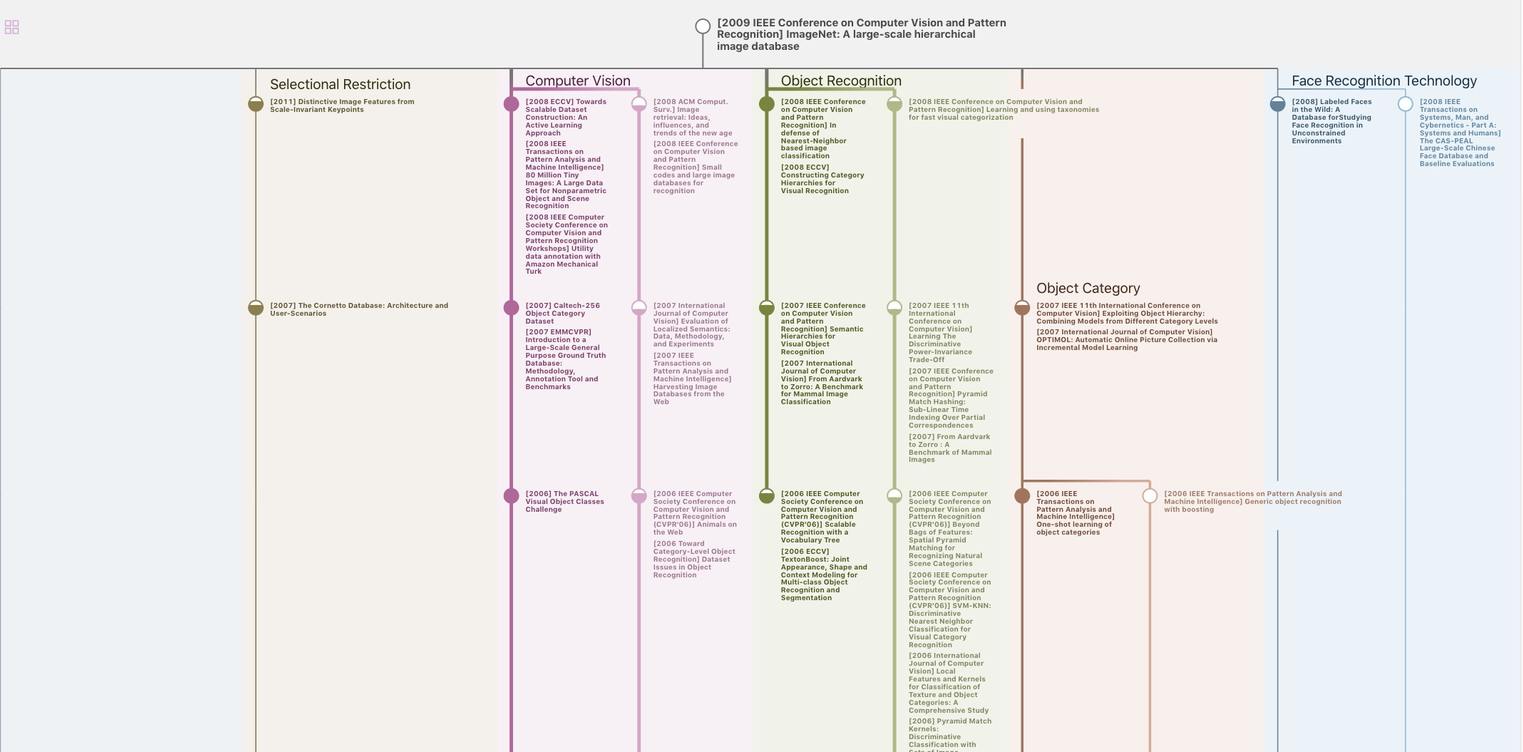
生成溯源树,研究论文发展脉络
Chat Paper
正在生成论文摘要