Robust Object Tracking Based On Deep Feature And Dual Classifier Trained With Hard Samples
ADVANCES IN NEURAL NETWORKS - ISNN 2019, PT II(2019)
摘要
Visual tracking has attracted more and more attention in recent years. In this paper, we proposed a novel tracker that is composed of a feature network, a dual classifier, a target location module, and a sample collecting and pooling module. The dual classifier contains two classifiers, called long-term classifier and short-term classifier, in which the long-term classifier is to maintain the long-term appearance of the target and the short-term classifier is for prompt response to the sudden change of the target. The training samples are divided into positive samples, negative samples, hard positive samples and hard negative samples and are used to train the two classifiers, respectively. Furthermore, in order to overcome the unreliability in locating the target by highest score, a density clustering method is introduced into the target locating process. Experimental results conducted on two benchmark datasets demonstrate the effectiveness of the proposed tracking method.
更多查看译文
关键词
Visual tracking, Ensemble tracking, Hard samples, Density clustering
AI 理解论文
溯源树
样例
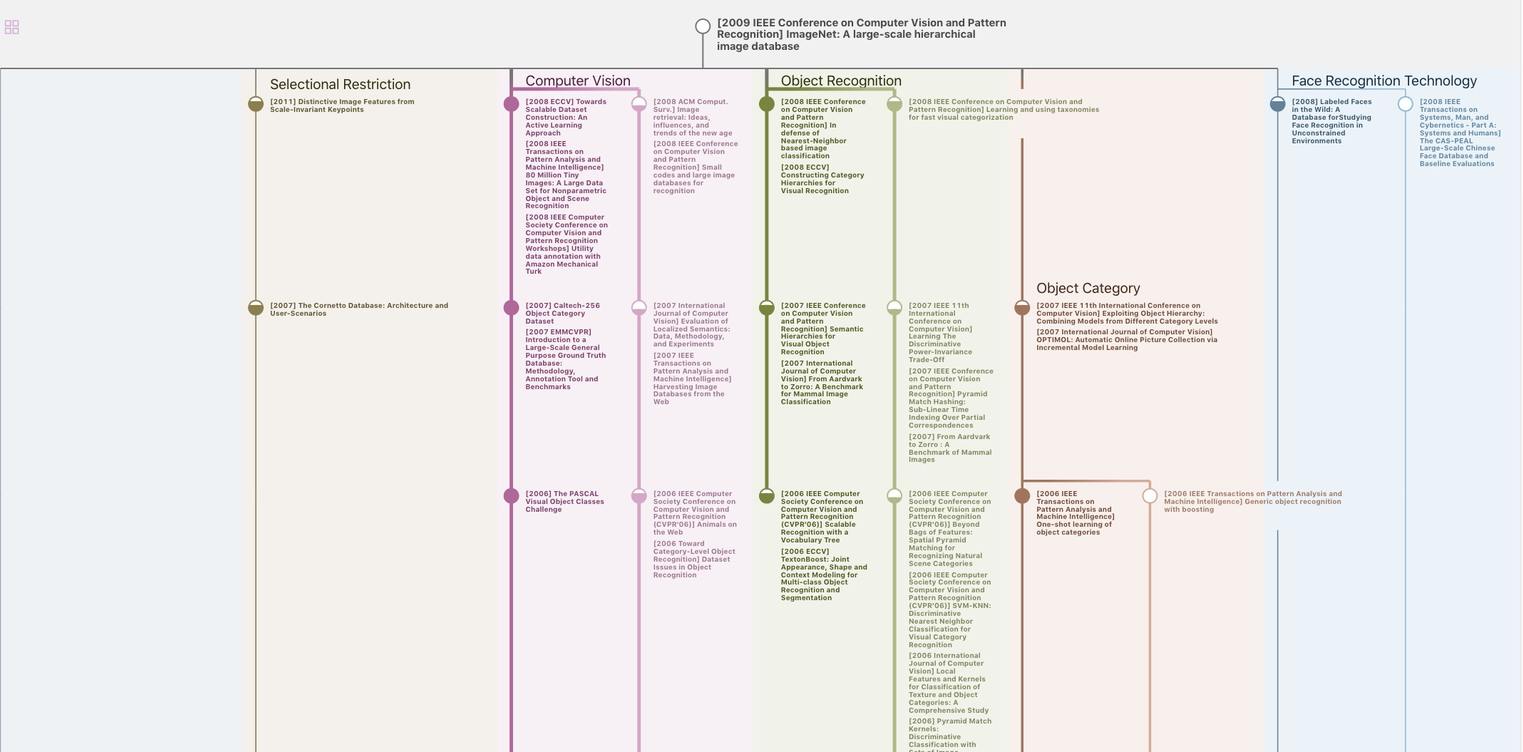
生成溯源树,研究论文发展脉络
Chat Paper
正在生成论文摘要