Function And Pattern Extrapolation With Product-Unit Networks
COMPUTATIONAL SCIENCE - ICCS 2019, PT II(2019)
摘要
Neural networks are a popular method for function approximation and data classification and have recently drawn much attention because of the success of deep-learning strategies. Artificial neural networks are built from elementary units that generate a piecewise, often almost linear approximation of the function or pattern. To improve the extrapolation of nonlinear functions and patterns beyond the training domain, we propose to augment the fundamental algebraic structure of neural networks by a product unit that computes the product of its inputs raised to the power of their weights. Linearly combining their outputs in a weighted sum allows representing most nonlinear functions known in calculus, including roots, fractions and approximations of power series. We train the network using stochastic gradient descent. The enhanced extrapolation capabilities of the network are demonstrated by comparing the results for a function and pattern extrapolation task with those obtained using the nonlinear support vector machine (SVM) and a standard neural network (standard NN). Convergence behavior of stochastic gradient descent is discussed and the feasibility of the approach is demonstrated in a real-world application in image segmentation.
更多查看译文
关键词
Product units, Neural network, Function extrapolation
AI 理解论文
溯源树
样例
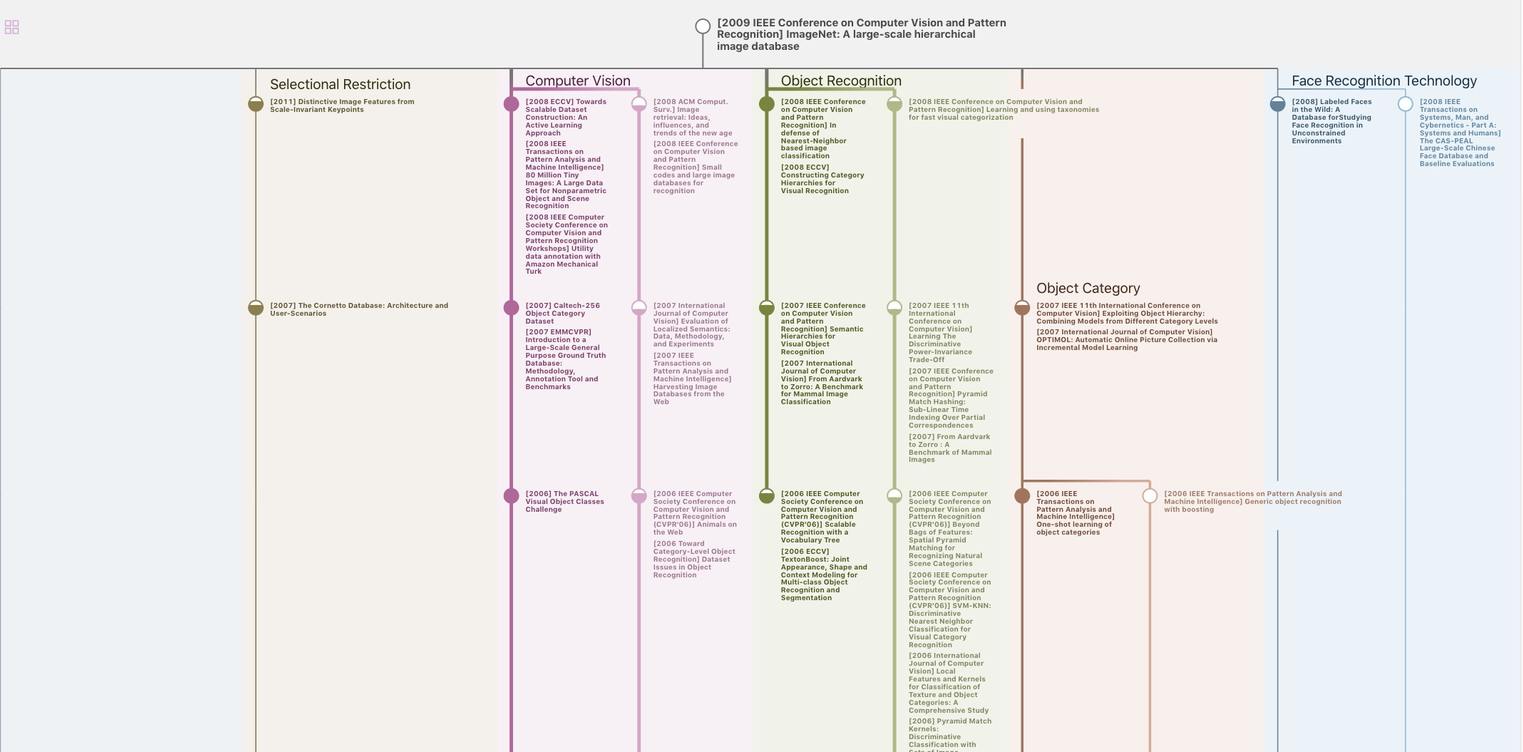
生成溯源树,研究论文发展脉络
Chat Paper
正在生成论文摘要