Adaptive Objective Functions And Distance Metrics For Recommendation Systems
COMPUTATIONAL SCIENCE - ICCS 2019, PT II(2019)
摘要
We describe, develop, and implement different models for the standard matrix completion problem from the field of recommendation systems. We benchmark these models against the publicly available Netflix Prize challenge dataset, consisting of users' ratings of movies on a 1-5 scale. While the original competition concentrated only on RMSE, we experiment with different objective functions for model training, ensemble construction, and model/ensemble testing.Our best-performing estimators were (1) a linear ensemble of base models trained using linear regression (see ensemble e(1), RMSE: 0.912) and (2) a neural network that aggregated predictions from individual models (see ensemble e(4), RMSE: 0.912). Many of the constituent models in our ensembles had yet to be developed at the time the Netflix competition concluded in 2009. To our knowledge, not much research has been done to establish best practices for combining these models into ensembles. We consider this problem, with a particular emphasis on the role that the choice of objective function plays in ensemble construction.
更多查看译文
AI 理解论文
溯源树
样例
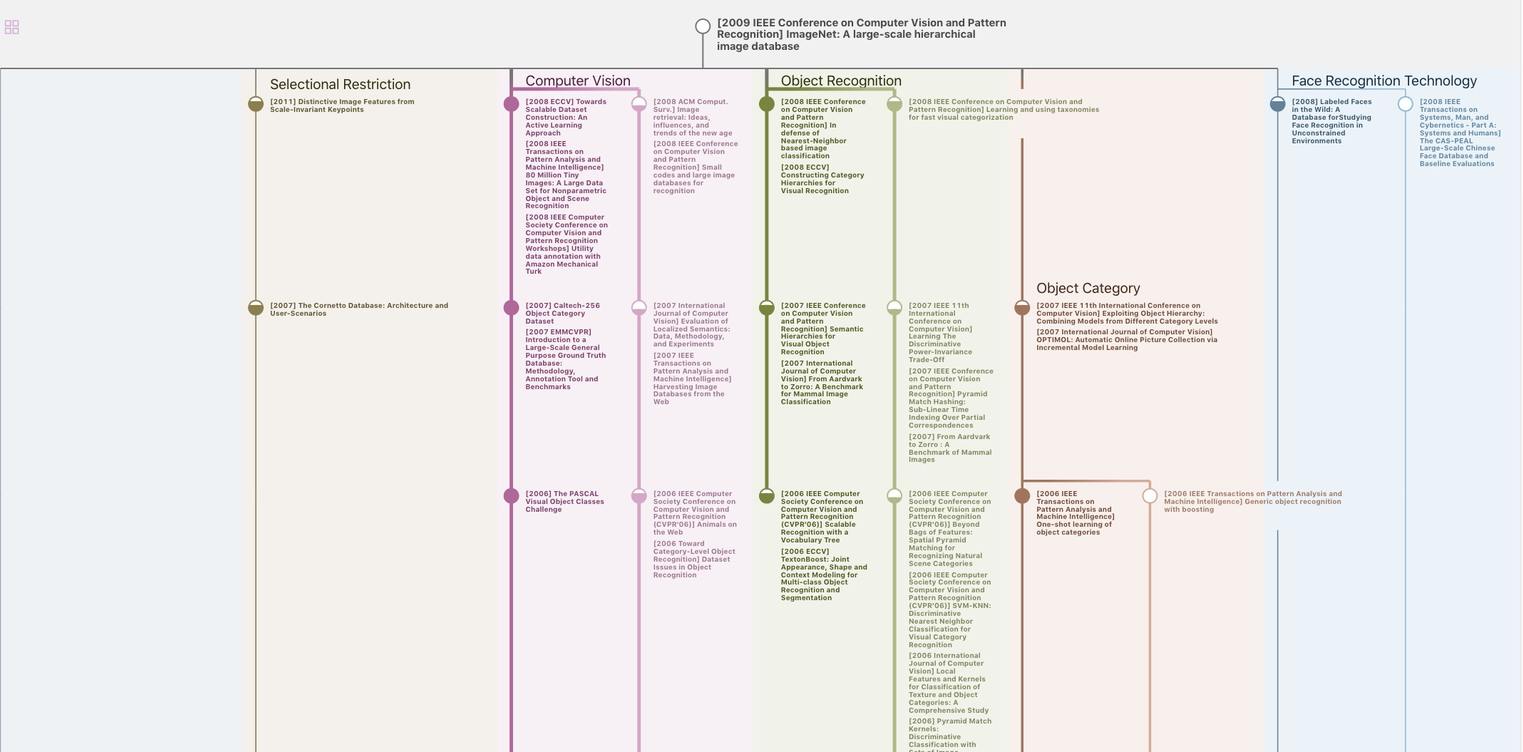
生成溯源树,研究论文发展脉络
Chat Paper
正在生成论文摘要