Discriminant Manifold Learning With Graph Convolution Based Regression For Image Classification
GRAPH-BASED REPRESENTATIONS IN PATTERN RECOGNITION, GBRPR 2019(2019)
摘要
Many learning problems can be cast into learning from data-driven graphs. This paper introduces a framework for supervised and semi-supervised learning by estimating a non-linear embedding that incorporates Spectral Graph Convolutions structure. The proposed algorithm exploits data-driven graphs in two ways. First, it integrates data smoothness over graphs. Second, the regression is solved by the joint use of the data and their graph in the sense that the regressor sees convolved data samples. The resulting framework can solve the problem of over-fitting on local neighborhood structures for image data having varied natures like outdoor scenes, faces and man-made objects. Our proposed approach not only provides a new perspective to non-linear embedding research but also induces the standpoint on Spectral Graph Convolutions methods. In order to evaluate the performance of the proposed method, a series of experiments are conducted on four image datasets in order to compare the proposed method with some state-of-art algorithms. This evaluation demonstrates the effectiveness of the proposed embedding method.
更多查看译文
关键词
Graph-based embedding, Supervised learning, Semi-supervised learning, Spectral graph convolutions, Discriminative embedding
AI 理解论文
溯源树
样例
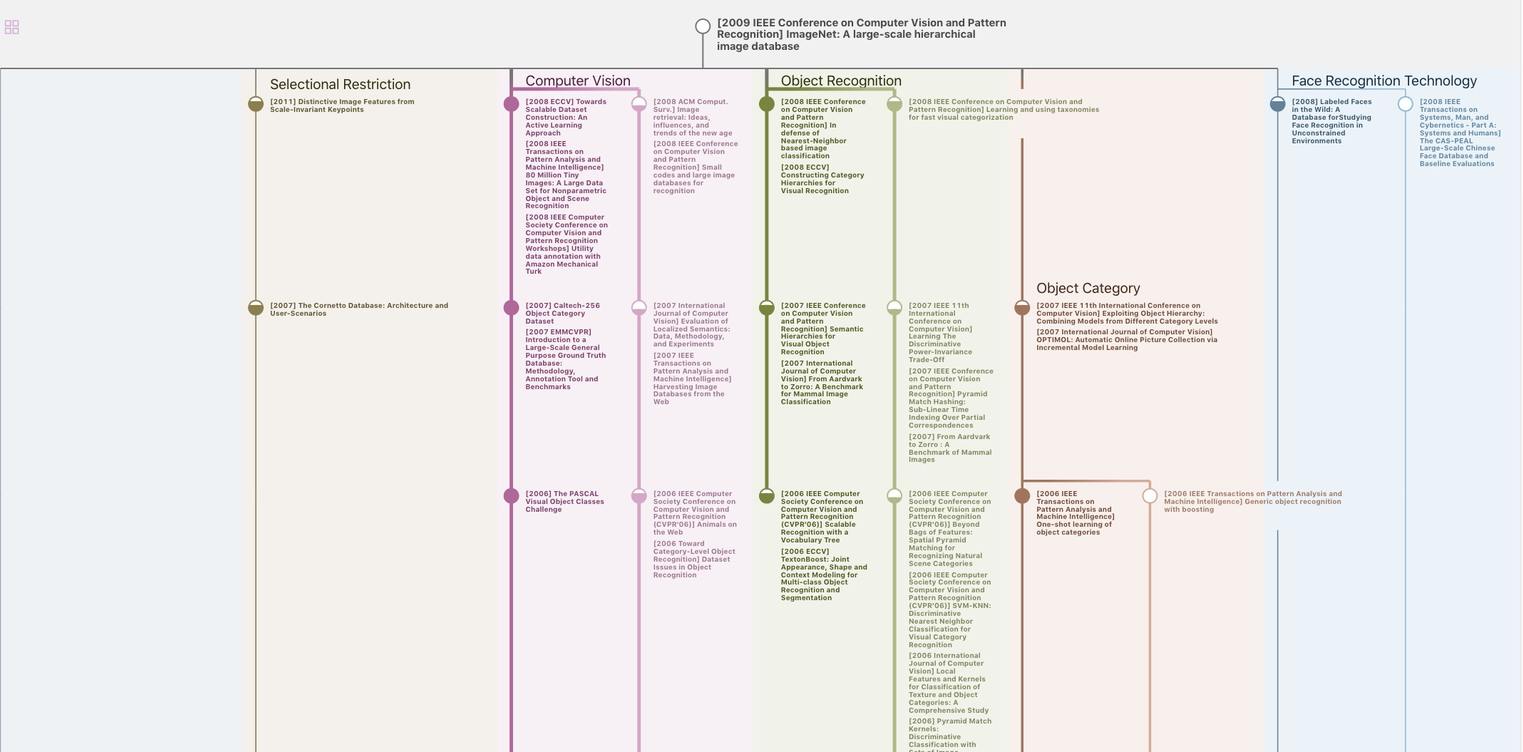
生成溯源树,研究论文发展脉络
Chat Paper
正在生成论文摘要