Improving Short Answer Grading Using Transformer-Based Pre-Training
ARTIFICIAL INTELLIGENCE IN EDUCATION (AIED 2019), PT I(2019)
摘要
Dialogue-based tutoring platforms have shown great promise in helping individual students improve mastery. Short answer grading is a crucial component of such platforms. However, generative short answer grading using the same platform for diverse disciplines and titles is a crucial challenge due to data distribution variations across domains and a frequent occurrence of non-sentential answers. Recent NLP research has introduced novel deep learning architectures such as the Transformer, which merely uses self-attention mechanisms. Pre-trained models based on the Transformer architecture have been used to produce impressive results across a range of NLP tasks. In this work, we experiment with fine-tuning a pre-trained self-attention language model, namely Bidirectional Encoder Representations from Transformers (BERT) applying it to short answer grading, and show that it produces superior results across multiple domains. On the benchmarking dataset of SemEval-2013, we report up to 10% absolute improvement in macro-average-F1 over state-of-the-art results. On our two psychology domain datasets, the fine-tuned model yields classification almost up to the human-agreement levels. Moreover, we study the effectiveness of fine-tuning as a function of the size of the task-specific labeled data, the number of training epochs, and its generalizability to cross-domain and join-domain scenarios.
更多查看译文
关键词
Self-attention, Transfer learning, Student answer scoring
AI 理解论文
溯源树
样例
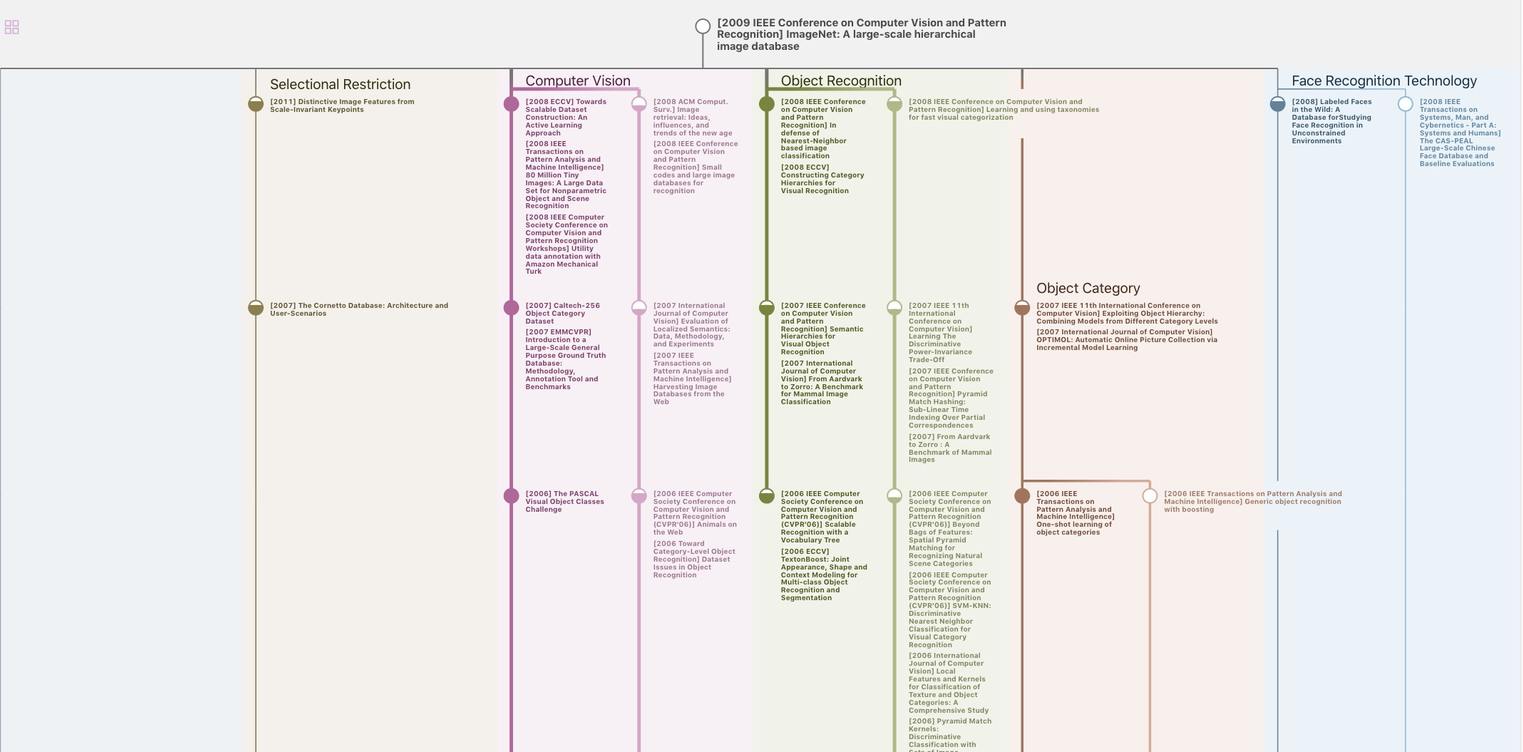
生成溯源树,研究论文发展脉络
Chat Paper
正在生成论文摘要