Interpretable Counterfactual Explanations Guided by Prototypes
MACHINE LEARNING AND KNOWLEDGE DISCOVERY IN DATABASES, ECML PKDD 2021: RESEARCH TRACK, PT II(2021)
摘要
We propose a fast, model agnostic method for finding interpretable counterfactual explanations of classifier predictions by using class prototypes. We show that class prototypes, obtained using either an encoder or through class specific k-d trees, significantly speed up the search for counterfactual instances and result in more interpretable explanations. We quantitatively evaluate interpretability of the generated counterfactuals to illustrate the effectiveness of our method on an image and tabular dataset, respectively MNIST and Breast Cancer Wisconsin (Diagnostic). Additionally, we propose a principled approach to handle categorical variables and illustrate our method on the Adult (Census) dataset. Our method also eliminates the computational bottleneck that arises because of numerical gradient evaluation for black box models.
更多查看译文
关键词
Interpretation, Transparency/Explainability, Counterfactual explanations
AI 理解论文
溯源树
样例
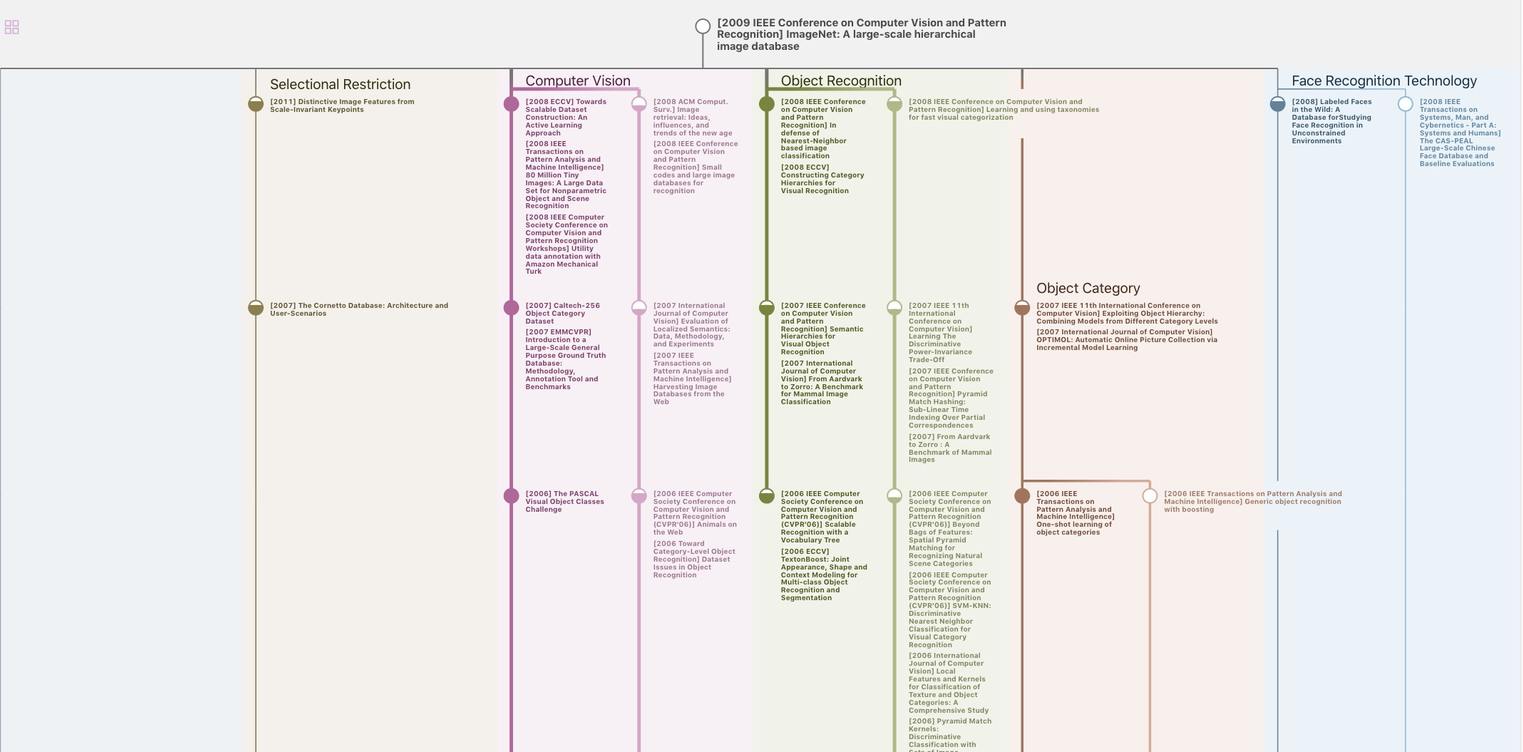
生成溯源树,研究论文发展脉络
Chat Paper
正在生成论文摘要