Large Scale Adversarial Representation Learning
ADVANCES IN NEURAL INFORMATION PROCESSING SYSTEMS 32 (NIPS 2019)(2019)
摘要
Adversarially trained generative models (GANs) have recently achieved compelling image synthesis results. But despite early successes in using GANs for unsupervised representation learning, they have since been superseded by approaches based on self-supervision. In this work we show that progress in image generation quality translates to substantially improved representation learning performance. Our approach, BigBiGAN, builds upon the state-of-the-art BigGAN model, extending it to representation learning by adding an encoder and modifying the discriminator. We extensively evaluate the representation learning and generation capabilities of these BigBiGAN models, demonstrating that these generation-based models achieve the state of the art in unsupervised representation learning on ImageNet, as well as in unconditional image generation. Pretrained BigBiGAN models - including image generators and encoders - are available on TensorFlow Hub(1).
更多查看译文
关键词
representation learning
AI 理解论文
溯源树
样例
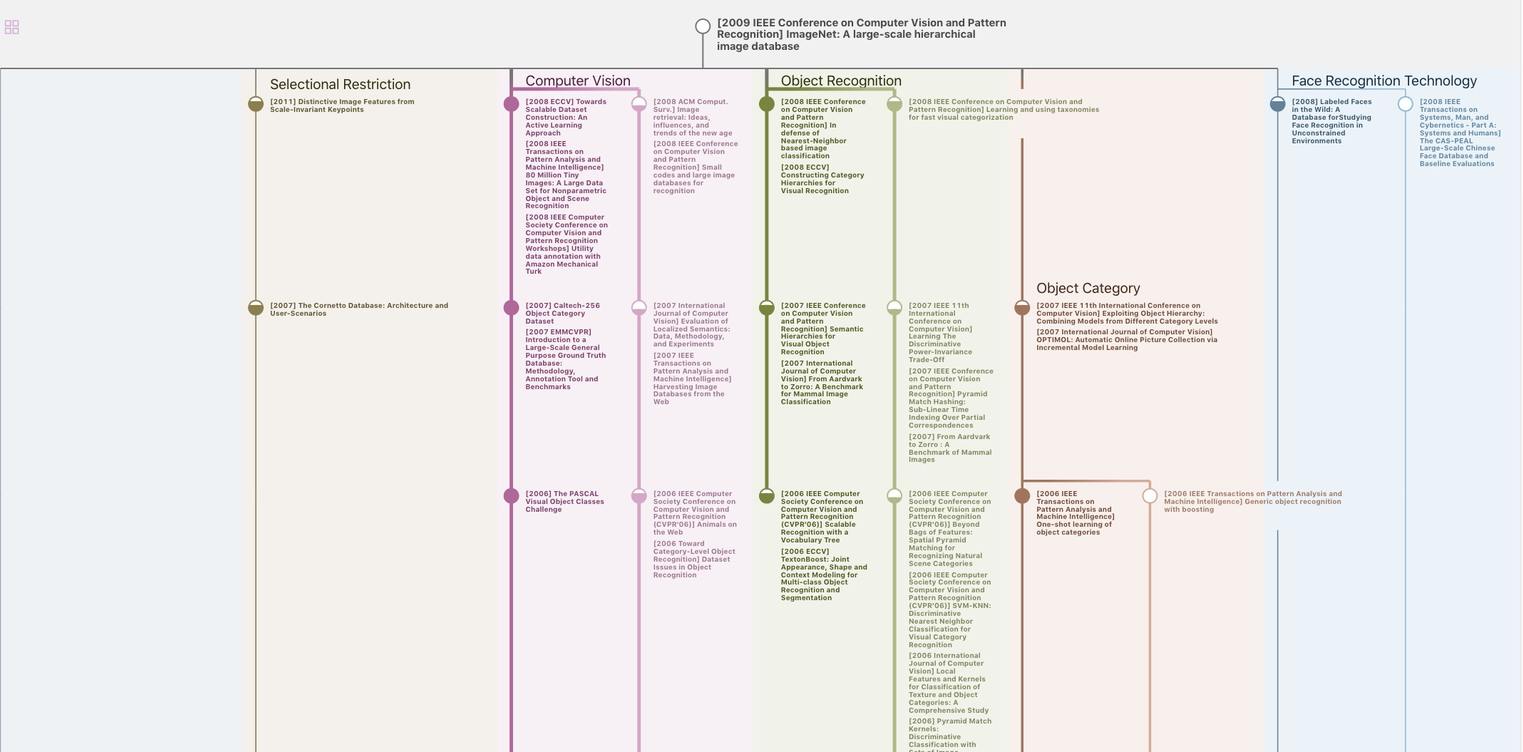
生成溯源树,研究论文发展脉络
Chat Paper
正在生成论文摘要