Learning to Select User-Specific Features for Top-N Recommendation of New Items
2019 IEEE 35th International Conference on Data Engineering Workshops (ICDEW)(2019)
摘要
Recommending new items to users remains a challenge due to the absence of user's past preferences for these items. Item features from side information are typically leveraged to tackle the problem. Existing methods formulate regression models, taking as input item features and as output user ratings. Availing of high dimensional item features, these methods are confronted with the issue of overfitting, which greatly impedes recommendation experience. In this work, we opt for feature selection to solve the problem of recommending top-N new items with high-dimensional side information. Existing feature selection methods find a common set of features for all users, which fails to differentiate user preferences over item features. To achieve personalization for feature selection, we propose to select item features specifically for users. The refined features filtered out the dimensions that are irrelevant to recommendations or unappealing to users. The experiment results on real-life datasets with high-dimensional side information reveal that the proposed method is effective in singling out features crucial to top-N recommendations and hence boosting the performance.
更多查看译文
关键词
Top-N recommendation,high dimensional side information,user-specific feature selection,cold-start
AI 理解论文
溯源树
样例
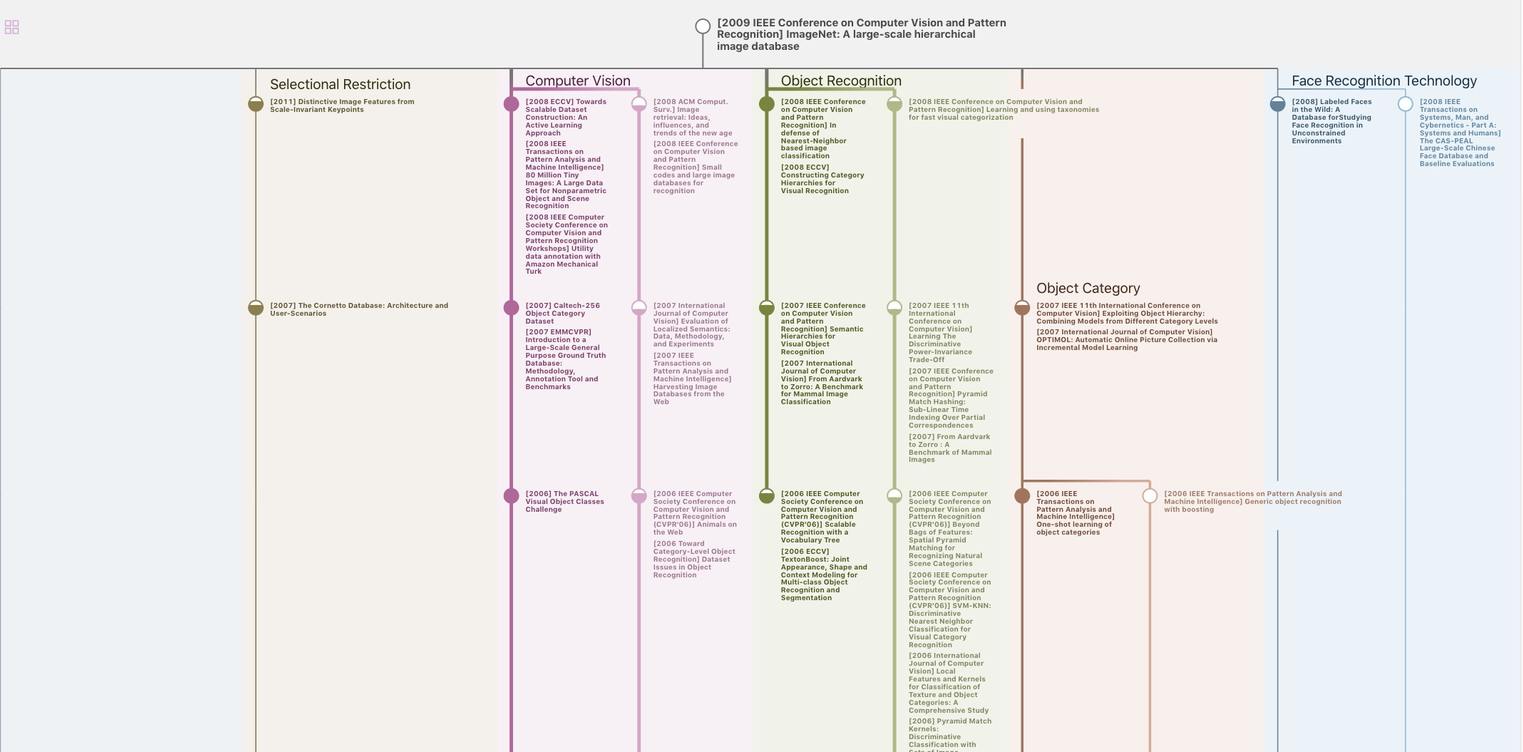
生成溯源树,研究论文发展脉络
Chat Paper
正在生成论文摘要