Towards Distributed Multi-model Learning on Apache Spark for Model-Based Recommender
2019 IEEE 35th International Conference on Data Engineering Workshops (ICDEW)(2019)
摘要
Model-based approaches for Content-based Filtering (CBF) recommendation have the potential of generating representative users models owing to their ability to learn from users actions. However, the need for training an individual model for each user leads to a scalability issue and brings a high computational cost that contributes to the limited adaptation of model-based approaches as efficient CBF recommenders. This is particularly relevant for production systems where the recommender is expected to serve a large number of users. In this work, we address the efficiency issue of model-based CBF recommender systems and present a new approach for distributed multi-model learning based on Apache Spark. We use Ranking SVM as the underlying recommendation algorithm and present a distributed implementation that allows efficient training of multiple models in parallel using a collection of machines. We demonstrate the efficiency of our approach on a real-world dataset from citeulike and show that our approach can reduce the cost of multi-model learning without affecting the prediction accuracy.
更多查看译文
关键词
Content based Filtering, Recommender Systems, Parallel computation, Apache Spark
AI 理解论文
溯源树
样例
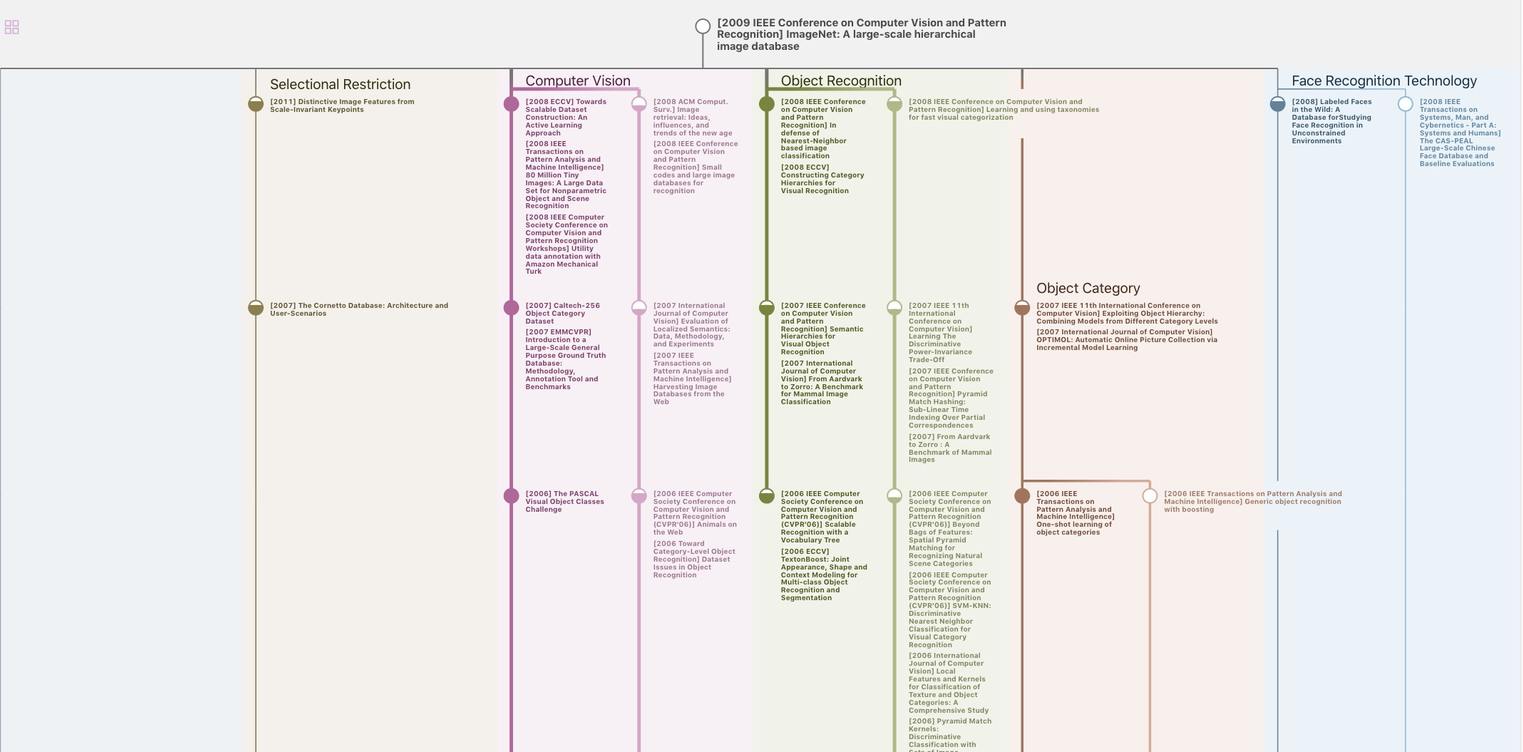
生成溯源树,研究论文发展脉络
Chat Paper
正在生成论文摘要