Efficient personalized community detection via genetic evolution
Genetic and Evolutionary Computation Conference(2019)
摘要
ABSTRACTPersonalized community detection aims to generate communities associated with user need on graphs, which benefits many downstream tasks such as node recommendation and link prediction for users, etc. It is of great importance but lack of enough attention in previous studies which are on topics of user-independent, semi-supervised, or top-K user-centric community detection. Meanwhile, most of their models are time consuming due to the complex graph structure. Different from these topics, personalized community detection requires to provide higher-resolution partition on nodes that are more relevant to user need while coarser manner partition on the remaining less relevant nodes. In this paper, to solve this task in an efficient way, we propose a genetic model including an offline and an online step. In the offline step, the user-independent community structure is encoded as a binary tree. And subsequently an online genetic pruning step is applied to partition the tree into communities. To accelerate the speed, we also deploy a distributed version of our model to run under parallel environment. Extensive experiments on multiple datasets show that our model outperforms the state-of-arts with significantly reduced running time.
更多查看译文
关键词
Personalized community detection, Graph mining, Network analysis, Genetic programming
AI 理解论文
溯源树
样例
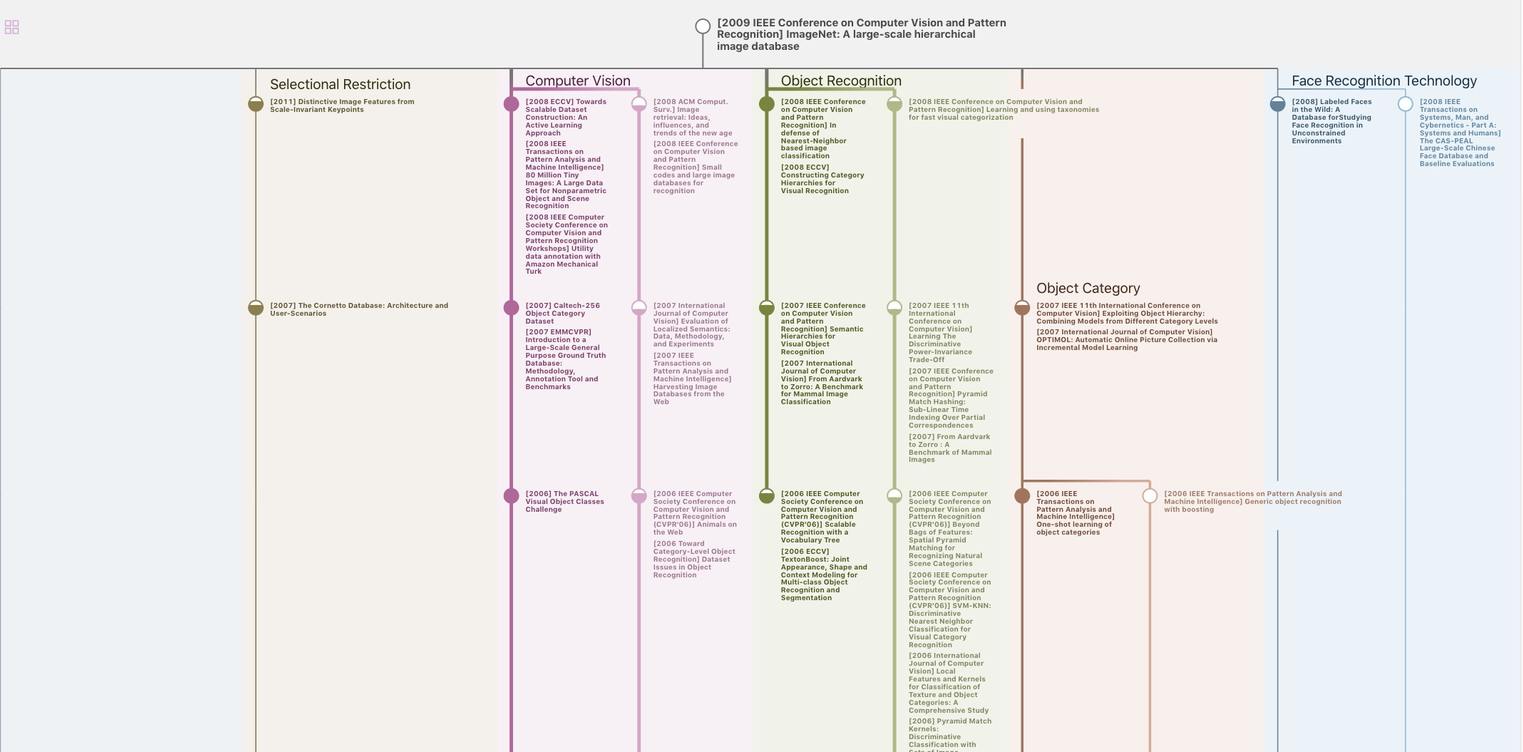
生成溯源树,研究论文发展脉络
Chat Paper
正在生成论文摘要