Learning a Domain-Invariant Embedding for Unsupervised Domain Adaptation Using Class-Conditioned Distribution Alignment
2019 57th Annual Allerton Conference on Communication, Control, and Computing (Allerton)(2019)
摘要
We address the problem of unsupervised domain adaptation (UDA) by learning a cross-domain agnostic embedding space, where the distance between the probability distributions of the two source and target visual domains is minimized. We use the output space of a shared cross-domain deep encoder to model the embedding space and use the Sliced-Wasserstein Distance (SWD) to measure and minimize the distance between the embedded distributions of two source and target domains to enforce the embedding to be domain-agnostic. Additionally, we use the source domain labeled data to train a deep classifier from the embedding space to the label space to enforce the embedding space to be discriminative. As a result of this training scheme, we provide an effective solution to train the deep classification network on the source domain such that it will generalize well on the target domain, where only unlabeled training data is accessible. To mitigate the challenge of class matching, we also align corresponding classes in the embedding space by using high confidence pseudo-labels for the target domain, i.e. assigning the class for which the source classifier has a high prediction probability. We provide experimental results on UDA benchmark tasks to demonstrate that our method is effective and leads to state-of-the-art performance.
更多查看译文
关键词
domain-invariant embedding,unsupervised domain adaptation,class-conditioned distribution alignment,cross-domain agnostic embedding space,target visual domains,output space,shared cross-domain deep encoder,embedded distributions,target domains,domain-agnostic,source domain,label space,target domain
AI 理解论文
溯源树
样例
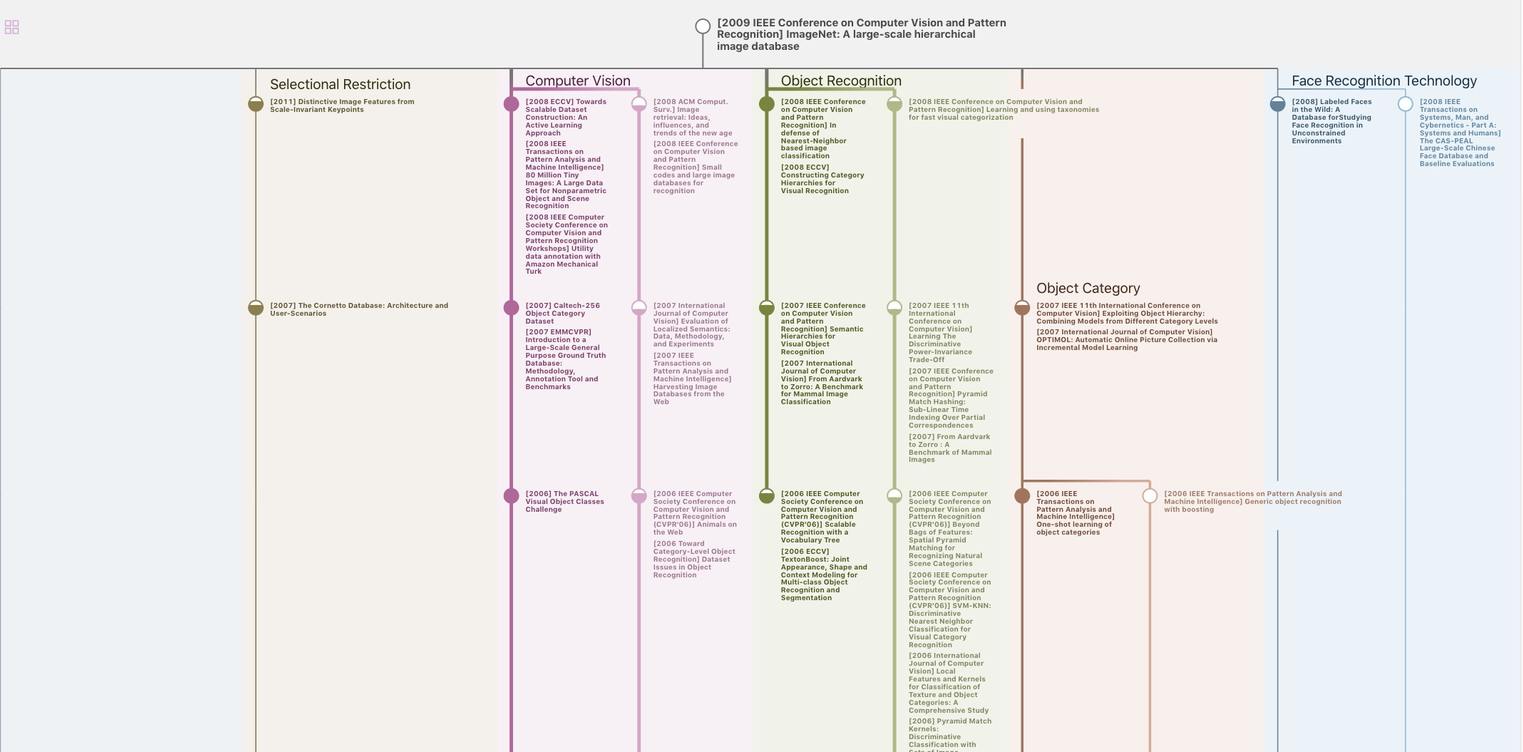
生成溯源树,研究论文发展脉络
Chat Paper
正在生成论文摘要