A Neural Named Entity Recognition and Multi-Type Normalization Tool for Biomedical Text Mining.
IEEE ACCESS(2019)
摘要
The amount of biomedical literature is vast and growing quickly, and accurate text mining techniques could help researchers to efficiently extract useful information from the literature. However, existing named entity recognition models used by text mining tools such as tmTool and ezTag are not effective enough, and cannot accurately discover new entities. Also, the traditional text mining tools do not consider overlapping entities, which are frequently observed in multi-type named entity recognition results. We propose a neural biomedical named entity recognition and multi-type normalization tool called BERN. The BERN uses high-performance BioBERT named entity recognition models which recognize known entities and discover new entities. Also, probability-based decision rules are developed to identify the types of overlapping entities. Furthermore, various named entity normalization models are integrated into BERN for assigning a distinct identifier to each recognized entity. The BERN provides a Web service for tagging entities in PubMed articles or raw text. Researchers can use the BERN Web service for their text mining tasks, such as new named entity discovery, information retrieval, question answering, and relation extraction. The application programming interfaces and demonstrations of BERN are publicly available at https://bern.korea.ac.kr.
更多查看译文
关键词
Biomedical text mining,decision rules,multi-type,named entity recognition,neural networks,normalization,Web service
AI 理解论文
溯源树
样例
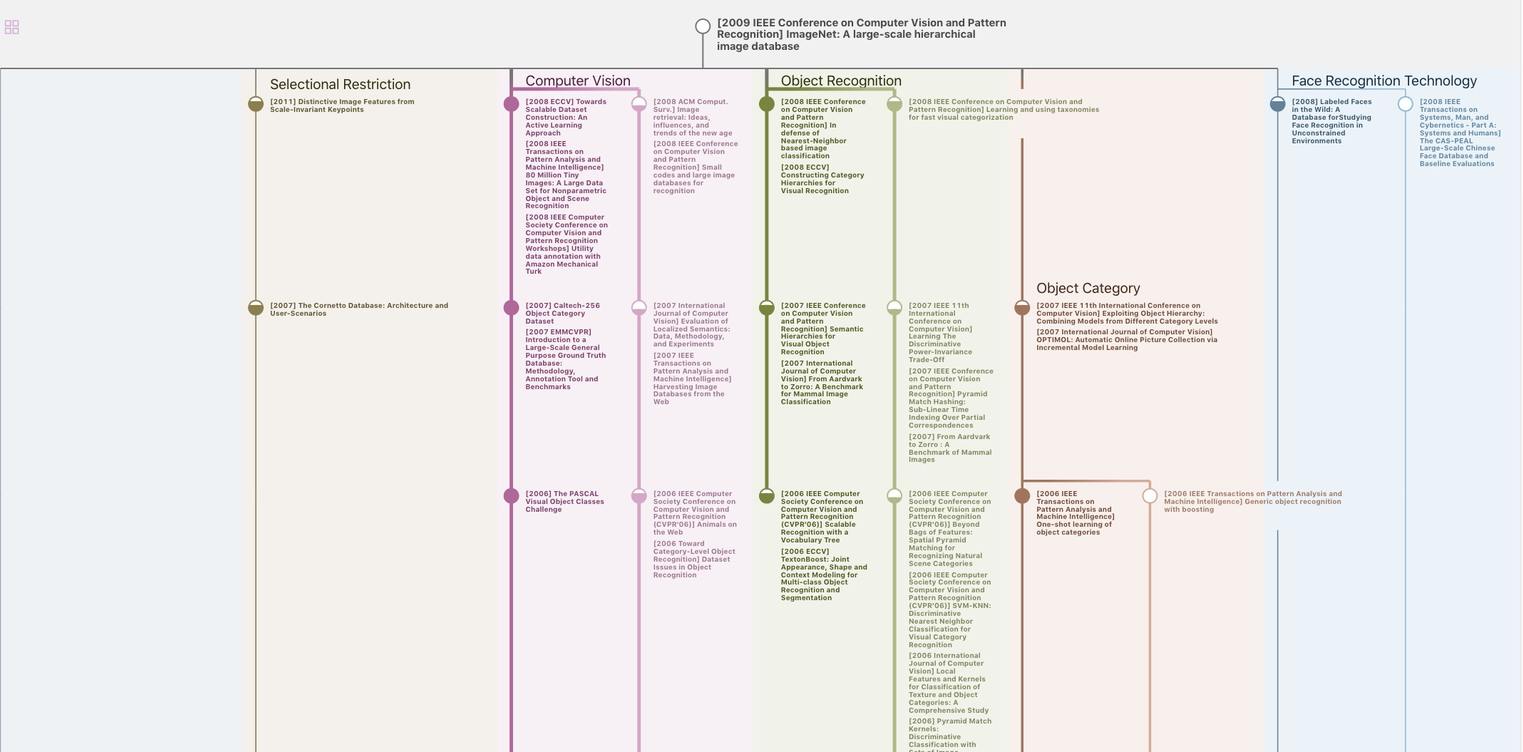
生成溯源树,研究论文发展脉络
Chat Paper
正在生成论文摘要