A Fast, Scalable, and Reliable Deghosting Method for Extreme Exposure Fusion
2019 IEEE International Conference on Computational Photography (ICCP)(2019)
摘要
HDR fusion of extreme exposure images with complex camera and object motion is a challenging task. Existing patch-based optimization techniques generate noisy and/or blurry results with undesirable artifacts for difficult scenarios. Additionally, they are computationally intensive and have high execution times. Recently proposed CNN-based methods offer fast alternatives, but still fail to generate artifact-free results for extreme exposure images. Furthermore, they do not scale to an arbitrary number of input images. To address these issues, we propose a simple, yet effective CNN-based multi-exposure image fusion method that produces artifact-free HDR images. Our method is fast, and scales to an arbitrary number of input images. Additionally, we prepare a large dataset of 582 varying exposure images with corresponding deghosted HDR images to train our model. We test the efficacy of our algorithm on publicly available datasets, and achieve significant improvements over existing state-of-the-art methods. Through experimental results, we demonstrate that our method produces artifact-free results, and offers a speed-up of around 54× over existing state-of-the-art HDR fusion methods.
更多查看译文
关键词
Exposure Fusion,High Dynamic Range Imaging,Deghosting
AI 理解论文
溯源树
样例
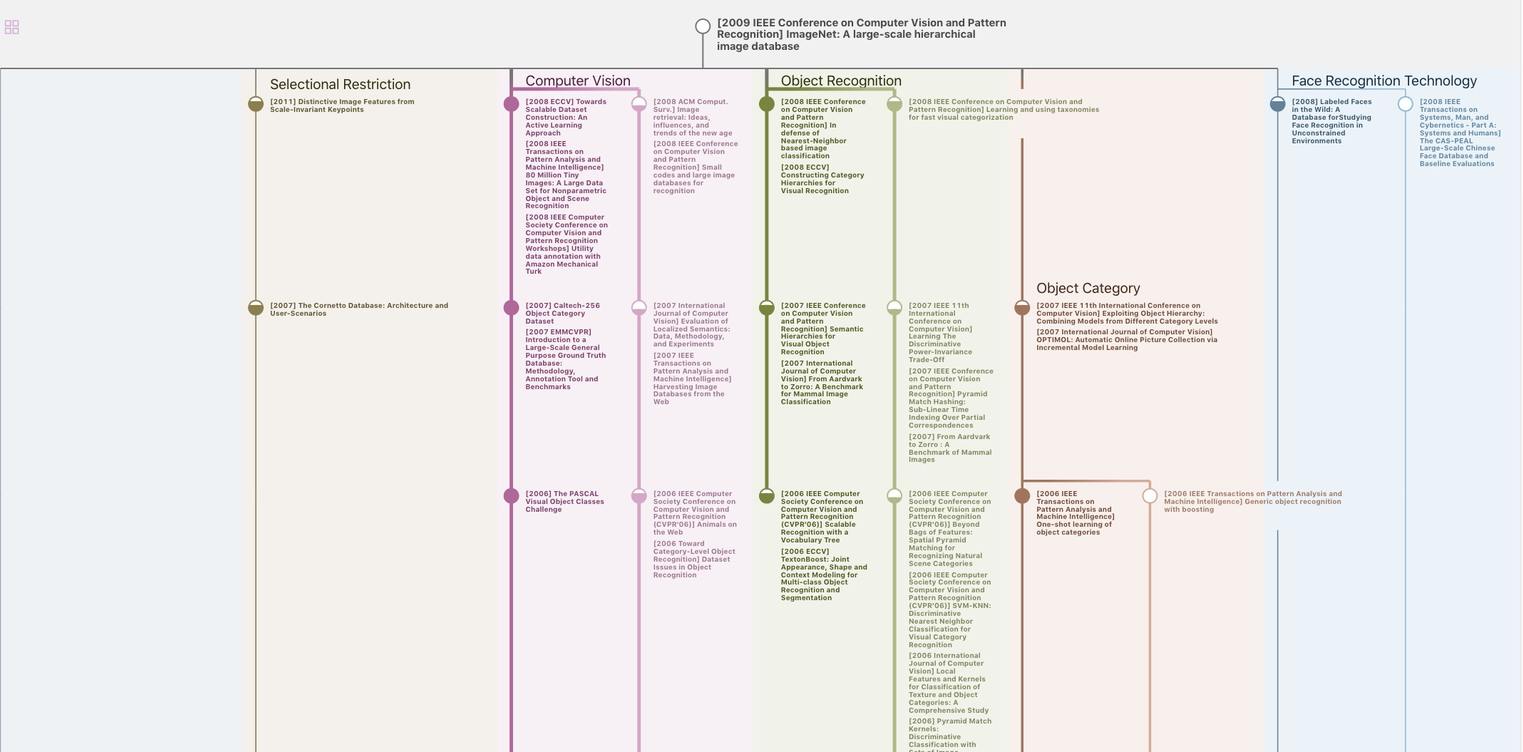
生成溯源树,研究论文发展脉络
Chat Paper
正在生成论文摘要