Hierarchically Non-continuous Regression Prediction for Short-Term Photovoltaic Power Output
2019 IEEE 16th International Conference on Networking, Sensing and Control (ICNSC)(2019)
摘要
Photovoltaic (PV) power generation utilizing clean solar energy is increasingly conducive to relieving energy crisis and environmental pollution. Affected by the fluctuation and uncertainty of meteorological factors, short-term PV power is volatile in nature, posing threats to power supply reliability and stability. Consequently, accurate PV production forecasting plays a vital role in steadily running and managing a power system. However, due to the intrinsic characteristics of variability and fluctuation in PV data, it is challenging to get acceptable output prediction via conventional regression methods. Moreover, the raw data in our regression task originates from a PV plant whose stored PV values are hierarchically non-continuous with conspicuously diverse classes, making it even harder to conduct precise prediction. In this paper, we propose a tree-based prediction model based on the XGBoost regression algorithm. The experimental results show that the proposed prediction model achieves the highest average forecasting accuracy and stable generalization performance, indicating its validity for hierarchically non-continuous short-term PV output prediction.
更多查看译文
关键词
short-term photovoltaic (PV) power forecasting,hierarchical non-continuous regression,outlier detection
AI 理解论文
溯源树
样例
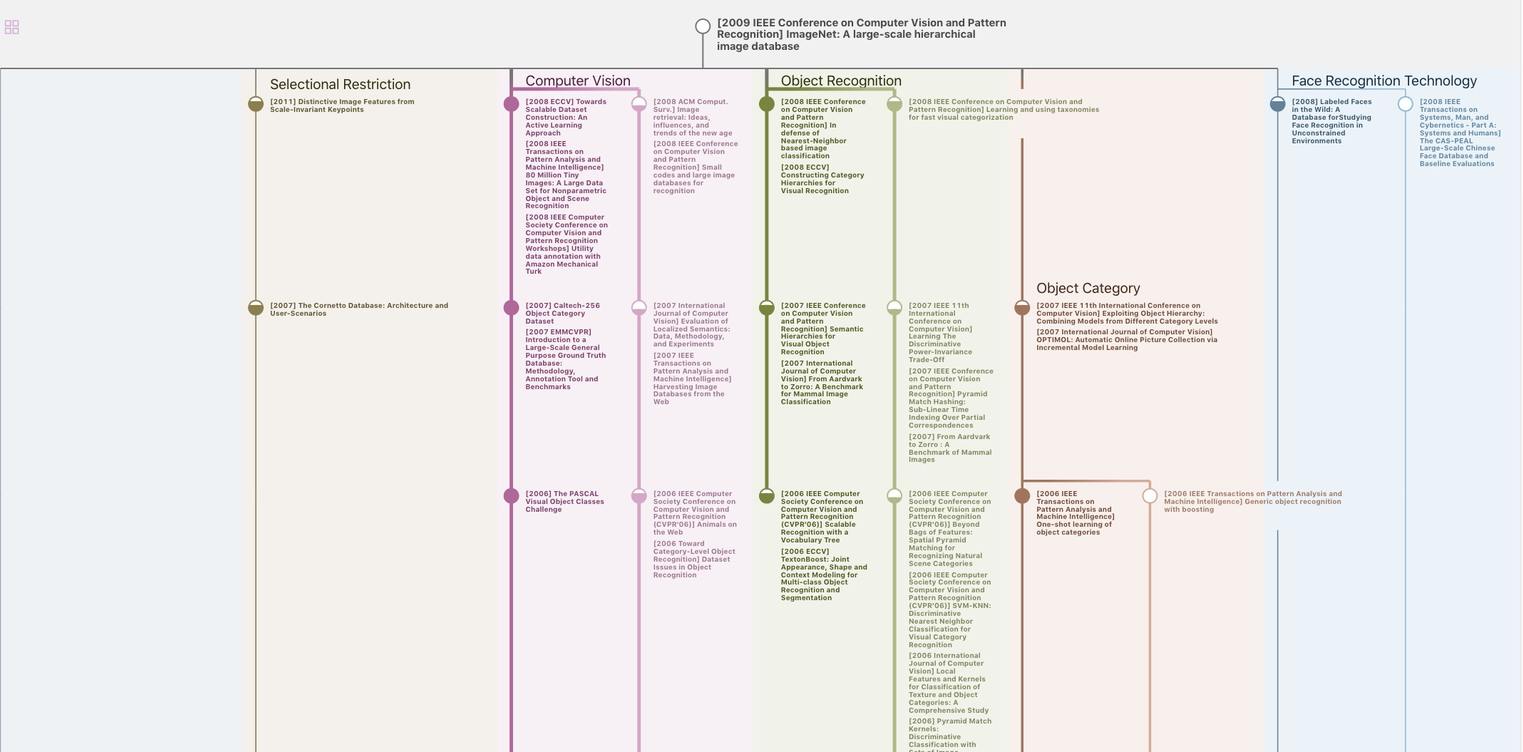
生成溯源树,研究论文发展脉络
Chat Paper
正在生成论文摘要